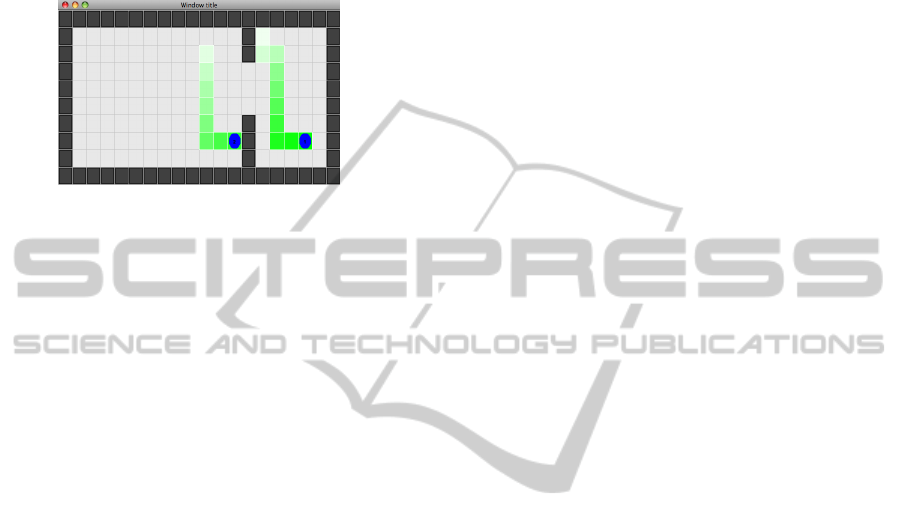
ent shades of green represent pheromones having dif-
ferent intensity. The experiments we carried out on
this simple case study were encouraging and demon-
strated the suitability of the Hive-BDI model and of
its implementation to cope with distributed coordina-
tion problems of this kind.
Figure 1: Screenshot taken from a simulation run.
4 CONCLUSIONS
Hive-BDI is suitable for modeling MASs composed
by a relatively large number of agents with limited
capabilities: the system draws its strength from the
cooperation and coordination of agents, giving birth
to an emerging intelligence.
Of course more complex agents, fully exploiting
the BDI potential, can be part of the MAS: the re-
sult would be a MAS involving agents that, despite
being homogeneous and fully interoperable, can be
clustered in different layers according to their reactiv-
ity/deliberativity. More reactive layers would consist
of many simple agents fully exploiting the Hive-BDI
features whereas more deliberative layers would con-
sist of “pure” Jason agents.
Using simpler entities means having less require-
ments in terms of computational and material re-
sources. This may be a desirable feature in real sce-
narios where software agents control physical robots.
When talking about robots, limited capabilities (e.g.,
limited strength or storage capacity, in the case of a
cleaning robot) may imply limited size, thus allowing
such machines to operate in environments otherwise
precluded to bigger ones.
As far as the future of this research activity is
concerned, we are working at enhancing the shared
knowledge base class by fully exploiting the ReSpecT
tuple center and we are experimenting Hive-BDI on
other case studies.
To conclude, the possibility given by Hive-BDI to
define conditions in an agent’s plan’s context, refer-
ring to the shared knowledge of the hive cluster rather
than the agent’s personal belief base really allows to
think of the MAS as a single entity, and therefore to
write plans accordingly: this actually would repre-
sent a paradigm shift in Multi Agent System program-
ming.
REFERENCES
Barbieri, M. (2010). Hive-BDI extending BDI agents with
shared memory. Bachelor’s thesis, University of gen-
ova. http://www.disi.unige.it/person/MascardiV/
Download/Barbieri-Matteo.pdf.
Bonabeau, E. (1999). Editor’s introduction: Stigmergy. Ar-
tificial Life, 5(2):95–96.
Bordini, R. H., Wooldridge, M., and H
¨
ubner, J. F. (2007).
Programming Multi-Agent Systems in AgentSpeak us-
ing Jason. John Wiley & Sons.
Coffey, S. and Clark, K. (2006). A hybrid, teleo-reactive
architecture for robot control. In MARS’06.
Dorigo, M., Maniezzo, V., and Colorni, A. (1996). The
Ant System: Optimization by a colony of cooperat-
ing agents. IEEE Transactions on Systems, Man, and
Cybernetics Part B: Cybernetics, 26(1):29–41.
Glinton, R., Sycara, K., Scerri, D., and Scerri, P. (2010).
The statistical mechanics of belief sharing in multi-
agent systems. Inf. Fusion, 11(3):256–266.
Hepple, A., Dennis, L. A., and Fisher, M. (2008). A com-
mon basis for agent organisation in BDI languages. In
LADS 2007.
Omicini, A. and Denti, E. (2001). Formal ReSpecT. Electr.
Notes Theor. Comput. Sci., 48.
Parunak, H. V. D., Brueckner, S., and Sauter, J. A. (2002).
Digital pheromone mechanisms for coordination of
unmanned vehicles. In AAMAS 2002. ACM.
Piunti, M. and Ricci, A. (2009). Cognitive use of artifacts:
Exploiting relevant information residing in MAS en-
vironments. In KRAMAS 2008.
Pynadath, D. V., Tambe, M., Chauvat, N., and Cavedon,
L. (2000). Toward team-oriented programming. In
ATAL’99.
Rao, A. S. (1996). AgentSpeak(L): BDI agents speak out in
a logical computable language. In MAAMAW’96.
Rao, A. S. and Georgeff, M. P. (1991). Modeling rational
agents within a BDI-architecture. In KR’91. Morgan
Kaufmann.
Ricci, A., Omicini, A., Viroli, M., Gardelli, L., and Oliva,
E. (2007). Cognitive stigmergy: Towards a framework
based on agents and artifacts. In E4MAS 2006.
Ricci, A., Viroli, M., and Omicini, A. (2008). The A&A
programming model and technology for developing
agent environments in MAS. In ProMAS 2007.
Valckenaers, P., Van Brussel, H., Kollingbaum, M. J., and
Bochmann, O. (2001). Multi-agent coordination and
control using stigmergy applied to manufacturing con-
trol. In EASSS 2001.
Velagapudi, P., Prokopyev, O., Scerri, P., and Sycara, K.
(2008). A token-based approach to sharing beliefs in
a large multiagent team. In Optimization and Cooper-
ative Control Strategies.
Velagapudi, P., Prokopyev, O., Sycara, K., and Scerri, P.
(2007). Maintaining shared belief in a large multia-
gent team. In FUSION 2007.
Vieira, R., Moreira, A. F., Wooldridge, M., and Bordini,
R. H. (2007). On the formal semantics of speech-act
based communication in an Agent-Oriented Program-
ming language. J. of Art. Intell. Research, 29.
ICAART 2011 - 3rd International Conference on Agents and Artificial Intelligence
482