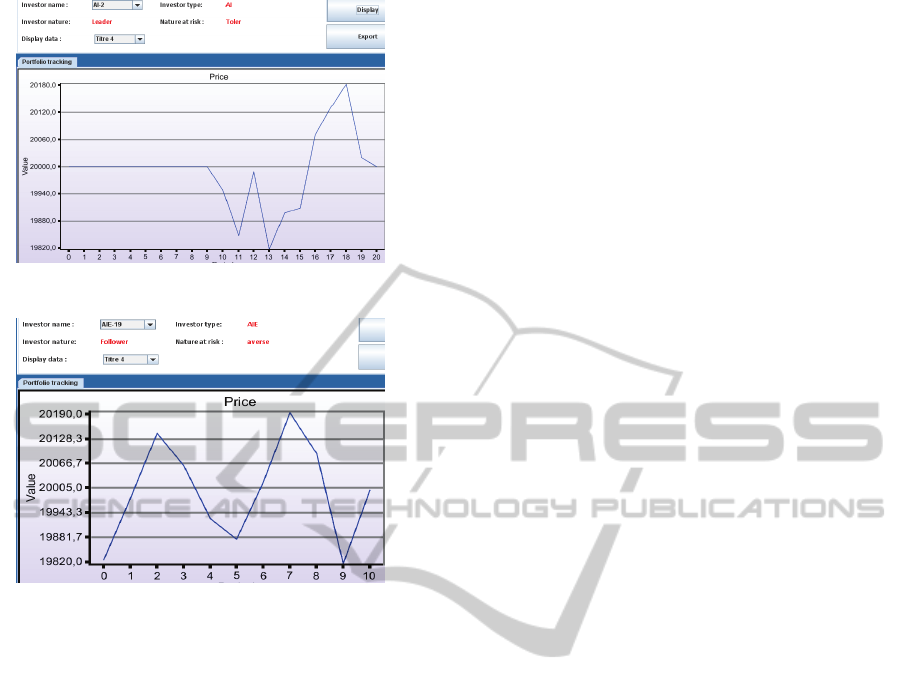
Figure 16: AI2 portfolio evolution with overhearing.
Figure 17: AIE19 portfolio evolution with overhearing.
The market liquidity i.e. there is at any time
purchasing and sales agents is maintained by agents
not based on overhearing according to the important
number of their transactions. If overhearing is
generalized in the market will certainly be less liquid
and more instable.
7 CONCLUSIONS
In this work, we have shown the need to simulate
financial markets in order to understand the
emergence of complex phenomena as unpredictable
as difficult to explain. We have analyzed different
existing models of artificial markets, and found that
most of them do not deal with order-driven financial
markets. In addition, these models do not pay
attention to the informal interactions between
investors. So we designed and implemented a new
model of order-driven markets, which operates
asynchronously and in which agents have been
endowed with sophisticated reasoning. The mental
models of the agents are supported by classifier
systems allowing them to learn from their
experiences and thereby improve their decisions.
These models have been tested, analyzed, and
proved their efficiency in finding the best behaviours
for investor agents. In addition, we have introduced
in our model an overhearing mechanism by offering
the opportunity to study the impact of informal
exchanged information in a financial market.
Through the proposed model, we have tested the
impact of overhearing on the global dynamic of the
market. We showed and discussed the results of
simulations and conducted experiments. Our
prototype can be extended and combined with a
social network structure for studying recurring
events in financial markets as speculative bubbles.
REFERENCES
Andersen, J., Sornette, D., 2003. The $-game, European
Physics Journal, pages 141–145.
Arthur, W. B., 1994. Inductive Reasoning and Bounded
Rationality, American Economic Review, 84(2).
Bak, P., Paczuski, M., Shubik, M., 1996. Price variations
in a stock market with many agents, arxiv.
Balbo, F., Maudet, N., Saunier, J., 2004. Interactions
opportunistes par l’écoute flottante, Université Paris-
Dauphine.
Buche, C., Septseault, C., De Loor, P., 2006. Proposition
d’un modèle générique pour l’implémentation d’une
famille de systèmes de classeurs, RSTI–RIA, 20(1).
Cincotti, S., Ponta, L., Pastore, S., 2006. Information-
based multi-assets artificial stock market with
heterogeneous agents, working paper, Geneva
University.
Derveeuw, J., 2008. Simulation multi-agents de marchés
Financiers, Thèse Doctorat de l’Université des
Sciences et Technologies de Lille.
Dugdale, J., Pavard, B., SOUBIE, J.L., 2000. A Pragmatic
Development of a Computer Simulation of an
emergency Call Center, In Cooperative Systems
Design, Frontiers in Artificial Intelligence and
Applications, Rose Dieng et al., IOS Press.
Holland, J. H., Holyoak, K. J., Nisbett, R. E., Thagard, P.
R., 1982. Classifier systems, Q-morphisms and
induction, In: Genetic Algorithms and Simulated
Annealing, ed. L. D. Davis.
Kotzé, Dr A. A., 2005. Stock Price Volatility: a primer,
Financial Chaos Theory.
LeBaron, B. L., Arthur, W.B., Palmer, R., 1999. Time
series properties of an artificial stock market, Journal
of Economic Dynamics and Control, 23, 1487–1516.
Legras, F., 2003. Organisation dynamique d’équipes
d’engins autonomes par écoute flottante, Thèse
doctorat, ONERA, Toulouse.
Markowitz, H. M., 1952. Portfolio Selection, Journal of
Finance, 7(1), 77–91.
Streichert F., Tanaka-Yamawaki M., Iwata M., 2006.
Effect of Moving Averages in the Tickwise Tradings in
the Stock Market. KES (3): 647-654.
ICAART 2011 - 3rd International Conference on Agents and Artificial Intelligence
350