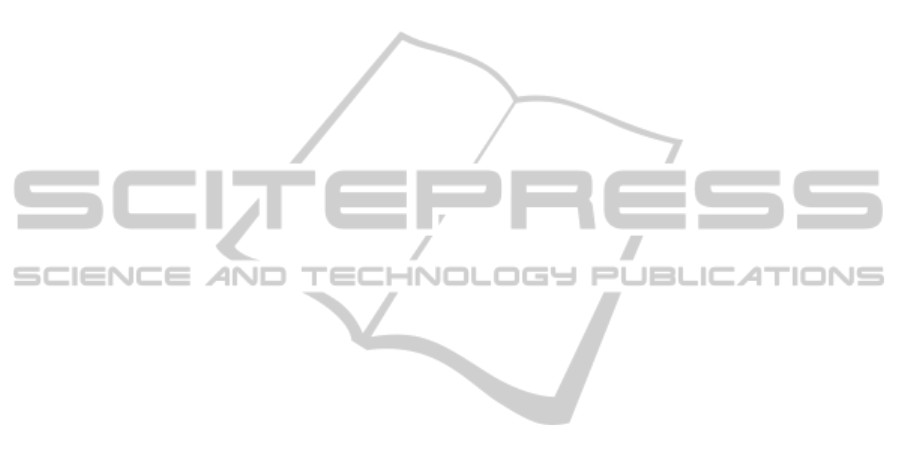
We take two steps to identify B, i.e., whitening
step and joint-diagonalization step.
The whitening step is achieved by applying to x(t)
a whitening matrix W satisfying:
E{Wx(t)x(t)
T
W
T
} = WC
x
(0)W
T
= WAA
T
W
T
= I.
In other words, whitening step correspond to principal
component analysis (PCA) procedure which removes
correlations between the observations x(t). This fol-
lows that if W is a whitening matrix, then there ex-
ists a orthogonal matrix U such that WA = U for
any whitening matrix W. With the orthogonal ma-
trix U, the mixture matrix A can be determined as
A = W
−1
U. The whitening step reduces the determi-
nation of the matrix A to that of a orthogonal matrix
U.
The next joint-diagonalization step is achieved
by finding U as a rotetion matrix which diagonalize
the time-delayed correlation matrices E{Wx(t)x(t +
τ
k
)
T
W
T
} simultaneously. Here, any rotating proce-
dure does not change the correlation matrix of zero
time delay.
Though only one additional time delayed corre-
lation matrix is required theoretically (Tong et al.,
1991)(Molgedey and Schuster, 1994), we may fail to
find the diagonalization matrix B if C
x
(τ) does not
have distinct eigenvalues. This can be avoided by
simultaneous diagonalization of multiple C
x
(τ
k
),1 ≤
k ≤ K (Belouchrani et al., 1997)(Ziehe and M¨uller,
1998). We use Jacobi like algorithm proposed by
Cardoso and Souloumiac (Cardoso and Souloumiac,
1996) based on several Givens rotations to find U.
2.3 Selection of Time Delays for
Periodic Sources on Ill-posed
Problem
In most theoretical discussions on the BSS problem,
we treat exactly determined problem in which we
can get exact solutions. In the joint-diagonalization
scheme, not much focus on the selection of time
delays is required if the problems are well-posed.
Theoretically, in case each source signal s(t) has its
auto-correlations and the correlation matrix for the
time delay has distinct eigenvalues, only one time de-
lay is required. Several time delays and their joint-
diagonalizations are effective in case the correlation
matrices have the same or almost the same eigen-
values (Belouchrani et al., 1997)(Ziehe and M¨uller,
1998).
However, the problems are ill-posed in most prac-
tical cases, especially in MEG recordings, and only
approximated solutions can be obtained. There is
no orthogonal matrix that makes all the correlation
matrices with the time delay diagonal on the prac-
tical ill-posed situations. In this case, the joint-
diagonalization algorithm minimizes the sum of the
off-diagonal values to obtain the target orthogonal
matrix.
Generally, in ill-posed problems, the separation
performance depends on the trade-off between the
source signals. In the case of joint-diagonalization,
the results vary depending on the selection of the set
of the time delay τ. If specific sources have to be ex-
tracted, we have to set the number and value of time
delays depending on the condition and achive good
separation performance.
In case the target signals have specific features in
the auto-correlation function, we can select the values
of time delays depending on that. If the signals are
periodic with cycle T, the auto-correlation function
has peaks on the time delay kT, k = 1, 2, · ·· and we
can get larger evaluation values to minimize the peaks
by setting τ = kT, k = 1, 2, · ··.
Furthermore, if brain signals are not the target to
be separated, it is important to choose the time delays
to avoid the effect of brain signals. Because almost all
brain signals have prominent auto-correlation values
within 1 s of the time delay, it is effective to choose
time delays over 1 s to avoid the effect of brain sig-
nals.
In conclusion, for the separation and elimination
of power supply noise of 60 Hz, we set the time delay
as τ = kT, k = 61, 62, ··· , and T = 1/60 (s). The total
number of τ may be chosen such that it is sufficient for
convergence.
3 RESULTS
As an example of the separation of periodic signals
from MEG data, we tried to separate 60 Hz power
supply noise and eliminate them from the data.
Figure 1 shows auto-correlation functions of sep-
arated signals (SS). We selected five signals from sep-
arated 64 signals, labeled SS1 to SS4, which have
remarkable peaks showing time delays associated
with 60 Hz cycle and its higher harmonics on auto-
correlation functions. The peak values become con-
stant over 0.05 s, which approximately indicates the
noise originated power supply has little temporal fluc-
tuations. On the other hand, the signals originate from
the brain activities have temporal fluctuations, so that
the values of auto-correlations decrease after a short
period (SS9). This difference is a useful factor for the
separation. The time delay parameter used here was
τ = kT, k = 61,62,·· · ,80, T = 1/60 (s) for 60 Hz
power supply noise. This parameter set was chosen
BIOSIGNALS 2011 - International Conference on Bio-inspired Systems and Signal Processing
374