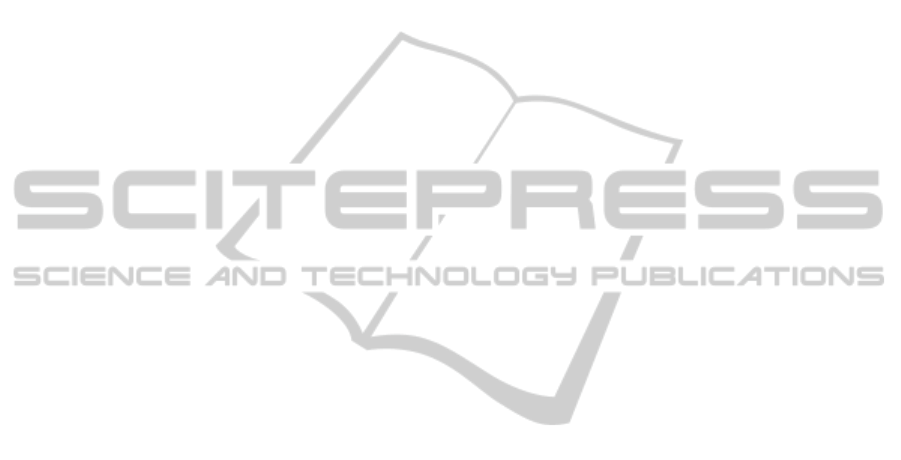
several types of music (jazz, rock, blue), etc. The
10000 cover MP3 audios are compressed by using
MP3Stego without any hidden date. We created
10000 steganograms by using MP3Stego with
random selected embedding strength less than the
maximum embedding capacity. The payloads
include voice, video, image, text, executable codes,
etc., and each steganogram carries a unique payload.
All MP3 audio files are obtained in 128 kbps bit-rate
and 44.1 kHz sample-rate. In the experiment, two
DENFIS systems will be trained and tested
separately, which provide embedding strength
estimation for audio streams and embedding
probabilities for individual frames. The prediction
accuracy will be used for evaluation.
5 CONCLUSIONS
In this paper we propose a method to predict the
embedding locations of MP3 steganography, which
is a critical step towards extracting hidden data from
steganogram. We design a reference based approach
to extract stream and frame features of the quantized
MDCT coefficients from audio streams and audio
frames, and dynamic evolving neural-fuzzy
inference systems are applied to the features for the
prediction.
Since we have a feature set with large dimension,
non-discriminative features will compromise the
prediction efficiency and accuracy. Therefore,
elaborate feature selection methods will contribute
to the enhancement of prediction.
ACKNOWLEDGEMENTS
We gratefully appreciate the Institute for Complex
Additive Systems Analysis of New Mexico Tech for
supporting this study.
REFERENCES
http://www.msnbc.msn.com/id/38028696/ns/technology_a
nd_science-science/
http://www.justice.gov/opa/documents/062810complaint1.
pdf
http://www.justice.gov/opa/documents/062810complaint2.
pdf
Ru, X., Zhang, H. and Huang, X. (2005). Steganalysis of
Audio: Attaching the Steghide. Proc. the Fourth
International Conference on Machine Learning and
Cybernetics, 3937-3942.
Kraetzer, C. and Dittmann, J. (2008). “Pros and Cons of
Mel-cepstrum Based Audio Steganalysis Using SVM
Classification. Lecture Notes in Computer Science,
4567, 359-377.
Liu, Q., Sung, A., and Qiao, M. (2009a). Temporal
Derivative-based Spectrum and Mel-cepstrum Audio
Steganalysis. IEEE Trans. on Info. Forensics and
Security, 4(3), 359-368.
Liu, Q., Sung, A., and Qiao, M. (2009b). Novel Stream
Mining for Audio Steganalysis. Proc. of 17th ACM
International Conference on Multimedia, 95-104.
Qiao, M., Sung, A., and Liu, Q. (2009a). Feature Mining
and Intelligent Computing for MP3 Steganalysis. Proc.
of International Joint Conference on Bioinformatics,
Systems Biology and Intelligent Computing, 627-630.
Qiao, M., Sung, A., and Liu, Q. (2009b). Steganalysis of
MP3Stego. Proc. of 22nd International Joint
Conference on Neural Networks, 2566-2571.
Shi, Y., Chen, C., and Chen, W. (2007). A Markov
Process Based Approach to Effective Attacking JPEG
Steganography. Lecture Notes in Computer Sciences,
437, 249-264.
Kasabov, N. and Song, Q. (2002). DENFIS: Dynamic
Evolving Neural-fuzzy Inference System and Its
Application for Time-series. IEEE Trans. Fuzzy
Systems, 10(2), 144-154.
LOCATING INFORMATION-HIDING IN MP3 AUDIO
507