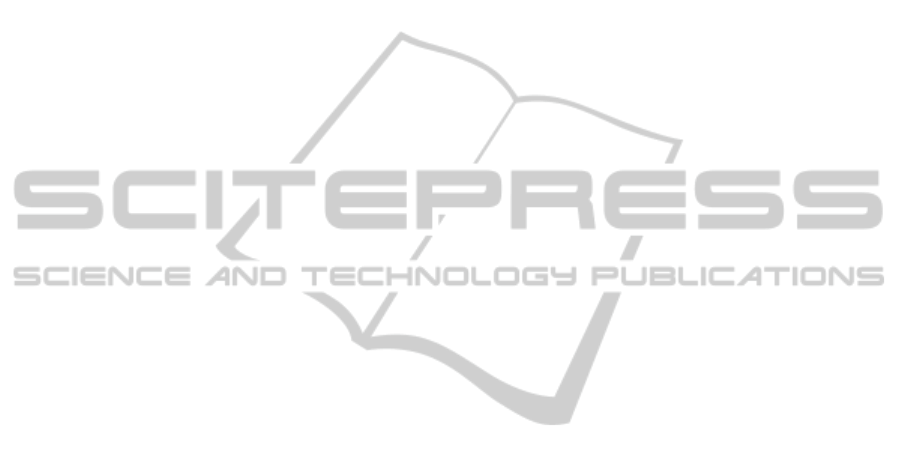
rigid, for example, the torso of human, especially
when the captured subjects perform vigorous exer-
cises like bending their body too low. In this situation,
our method may wrongly labeled markers attached to
these lax rigid bodies. However, the wrongly labeled
markers can be corrected using the constraint of tra-
jectories’ smoothness or solving the problem of abso-
lute orientation(Arun et al., 1987).
Our approach relies on the assumption that each
marker trajectory must be non-interrupted during the
whole motion. To accomplish the clustering task, we
also have to ask captured objects to exercise his joint
through the full range of motion. Although these
limitations are a little strict, but they can be satis-
fied in practice to ask the captured subject to perform
calibration motion in the middle of capturing area.
This requirementcan effectively reduce the number of
invisible markers and obtain almost non-interrupted
marker trajectories. For the task of clinical gait anal-
ysis, we should let the number of invisible markers
be as less as possible. In future work, we plan to re-
duce the limitations mentioned above in order to label
markers with noisy marker trajectories.
ACKNOWLEDGEMENTS
This work was supported by the Knowledge
Innovation Program of Chinese Academy of
Science(KGCX2-YW-610) and the National Key
Technology Research and Development Program of
China(2008BAI50B07).
REFERENCES
Aguiar, E. D., Theobalt, C., and Seidel, H.-P. (2006). Auto-
matic learning of articulated skeletons from 3d marker
trajectories. International Symposium on Visual Com-
puting, 1:485–494.
Arun, K., Huang, T., and Blostein, S. (1987). Least-squares
fitting of two 3-d point sets. IEEE Transactions on
Pattern Analysis and Machine Intelligence, 9(5):698–
700.
Bentley, J. L. (1975). Multidimensional binary search trees
used for associative searching. Communications of the
ACM, 18:509–517.
Bentley, J. L. (1990). K-d trees for semidynamic point sets.
In Proc.6th Ann. ACM Symp. on Computational Ge-
ometry, pages 187–197.
CMU, G. L. (2009). Motion capture database.
http://mocap.cs.cmu.edu.
Cox, G. S. and de Jager, G. (1992). A survey of point pattern
matching techniques and a new approach to point pat-
tern recognition. In COSMIG’92, Proc. South African
Symposium on Communications and Signal Process-
ing.
Gaede, V. and Gnther, O. (1998). Multidimensional access
methods. J. of Computer Surveys, 30(2):170–231.
Gleicher, M. (1999). Animation from observation: Mo-
tion capture and modtion editing. Computer Graphics,
33(4):51–55.
Guerra-Filho, G. (2005). Optical motion capture: Theory
and implementation. J. of Theoretical and Applied In-
formatics, 12(2):61–90.
Hornung, A., Sar-Dessai, S., and Kobbelt, L. (2005). Skele-
tal parameter estimation from optical motion capture
data. In VR’05,In: Proceedings of the IEEE Virtual
Reality Conference, pages 75–82. IEEE.
Kirk, A. G., O’Brien, J. F., and Forsyth, D. A. (2005).
Skeletal parameter estimation from optical motion
capture data. In CVPR’05,In: Proceedings of the
18th IEEE Conference on Computer Vision and Pat-
tern Recognition. IEEE.
Li, B., Meng, Q., and Holstein, H. (2003). Point pattern
matching and applicationsła review. In SMC’03,In:
Proceedings of the IEEE International Conference on
Systems,Man and Cybernetics.
Li, B., Meng, Q., and Holstein, H. (2004). Articulated pose
identification with sparse point features. IEEE Trans-
actions on Systems, Man, and Cybernetics PartB,
34(3):1412–1422.
Li, B., Meng, Q., and Holstein, H. (2005). Similarity
k-d tree method for sparse point pattern matching
with underlying non-rigidity. Pattern Recognition,
38(12):2391–2399.
Li, B., Meng, Q., and Holstein, H. (2008). Articulated
motion reconstruction from feature points. Pattern
Recognition, 41(1):418–431.
Mount, D., Netanyahu, N., and Moigne, J. (1999). Effi-
cient algorithms for robust feature matching. Pattern
Recognition, 32:17–38.
Ng, A. Y., Jordan, M. I., and Y.Weiss (2001). On spectral
clustering: Analysis and an algorithm. In NIPS’01,In:
Proceedings of the 15th Advances in Neural Informa-
tion Processing Systems, pages 849–856. MIT Press.
OMG (2009). Motion capture systems from vicon.
http://www.vicon.com/.
Wolfson, H. and Rigoutsos, I. (1997). Geometric hashing:
an overview. IEEE Transactions on Computational
Science and Engineering, 4(4):10–21.
Yu, Q., Li, Q., and Deng, Z. (2007). Online motion cap-
ture marker labeling for multiple interacting articu-
lated targets. Computer Graphics Forum (Proceedings
of Eurographics 2007), 26(3):477–483.
Zelnik-manor, L. and Perona, P. (2004). Self-tuning spectral
clustering. In Advances in Neural Information Pro-
cessing Systems 17, pages 1601–1608. MIT Press.
BIODEVICES 2011 - International Conference on Biomedical Electronics and Devices
294