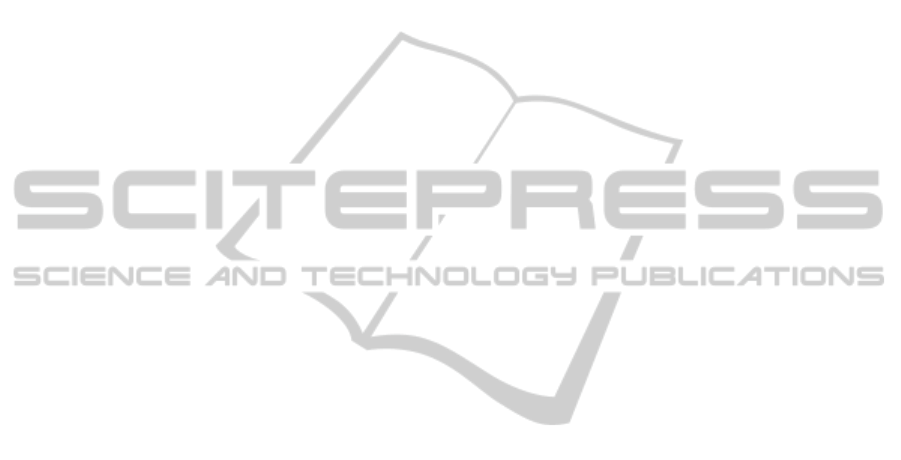
the visualization and usage of the knowledge gained
from building these graphs.
Although the simple implementation developed
in less than one man month gave interesting and
useful results that are currently being evaluated
during a field test with a partner company, some
limitations as well as ways to improve the tool can
be mentioned.
5.1 Limitations
An obvious limitation of the current state of the tool
is that all relations are co-occurrence relations and
are presented in the same way, regardless of their
actual meaning and importance. It would be helpful
to define a certain number of relation types and to
use the possibilities of a visual user interface to
distinguish between types and between relations that
can be defined as of main importance and of
secondary importance with regard to the use case.
Not only relations could be distinguished but
also company types. With regard to the competitive
intelligence use case, it would be helpful to display
partner companies in one color and competitor
companies in another color, further distinguishing
between customers, suppliers, etc.
Another kind of limitations comes from the
implementation: the whole graph being loaded once
and either completely updated or not at all. A more
interactive and on-the-fly data retrieval would help
the navigation in large company networks.
5.2 Future Work
Some of these limitations are to be addressed in our
future work. Using and adapting advanced text-
mining tools, it is possible to detect and classify a
certain number of relations between companies, such
as “customer of” or “acquirer of”, as shown e.g. by
Hu et al. (2009). This work will be accompanied by
a proposed classification of company relations types
(customer, supplier, etc.) and attributes (directed,
transitive, etc.). Another important aspect related to
this classification is the analysis of internal company
or group structures.
Another part of the planned work consists in the
improvement of the organization name
normalization algorithm. An aspect that was ignored
as of today is the multilingualism of Web sources,
which means that “Microsoft Germany” and
“Microsoft Deutschland” will be considered as two
distinct companies. This could for many cases be
addressed by well-built look-up lists.
An evaluation of the recall achieved by
automatic detection of company relations is also
planned.
REFERENCES
Finzen, Jan, Kintz, Maximilien, Kett, Holger, Koch,
Steffen. 2009. Strategic Innovation Management on
the Basis of Searching and Mining Press Releases.
Proceedings of the 5th WEBIST conference, Lisbon,
Portugal, March 23-26, 2009.
Finzen, Jan, Kintz, Maximilien: Innovation Mining. 2011.
Proceedings of the 7
th
WEBIST conference,
Noordwijkerhout, The Netherlands, May 06-09, 2011.
Heer Jeffrey, Card, Stuart K., Landay, James A. 2005.
Prefuse: a toolkit for interactive information
visualization. Proceedings of the SIGCHI conference
on Human factors in computing systems, Portland,
Oregon, USA, April 02-07, 2005.
Freeman, Linton C. 2000. Visualizing Social Groups.
Proceedings of the Section on Statistical Graphics.
American Statistical Association.
Buzgar, Adrian N., Buraga, Sabin C. 2008. Visualizing
Online Social Networks in the Context of Web 2.0.
Sisteme Distribuite, University Stefan cel Mare of
Suceava, Suceava, Romania.
Hu, Changjian, Xu, Liqin, Shen, Guoyang, Fukushima,
Toshikazu. 2009. Temporal Company Relation Mining
from the Web. Lecture Notes in Computer Science,
2009, Volume 5446/2009, 392-403.
Magnani, M., and Montesi, D. 2007. A study on company
name matching for database integration. Technical
Report UBLCS-07-15. May 2007.
Matsuo, Yutaka, Mori, Junichiro, Hamasaki, Masahiro,
Nishimura, Takuichi, Takeda, Hideaki, Hasida, Koiti,
and Ishizuka, Mitsuru. 2007. POLYPHONET: An
advanced social network extraction system from the
Web. Web Semantics. 5, 4 (December 2007), 262-278.
2007
WEBIST 2011 - 7th International Conference on Web Information Systems and Technologies
602