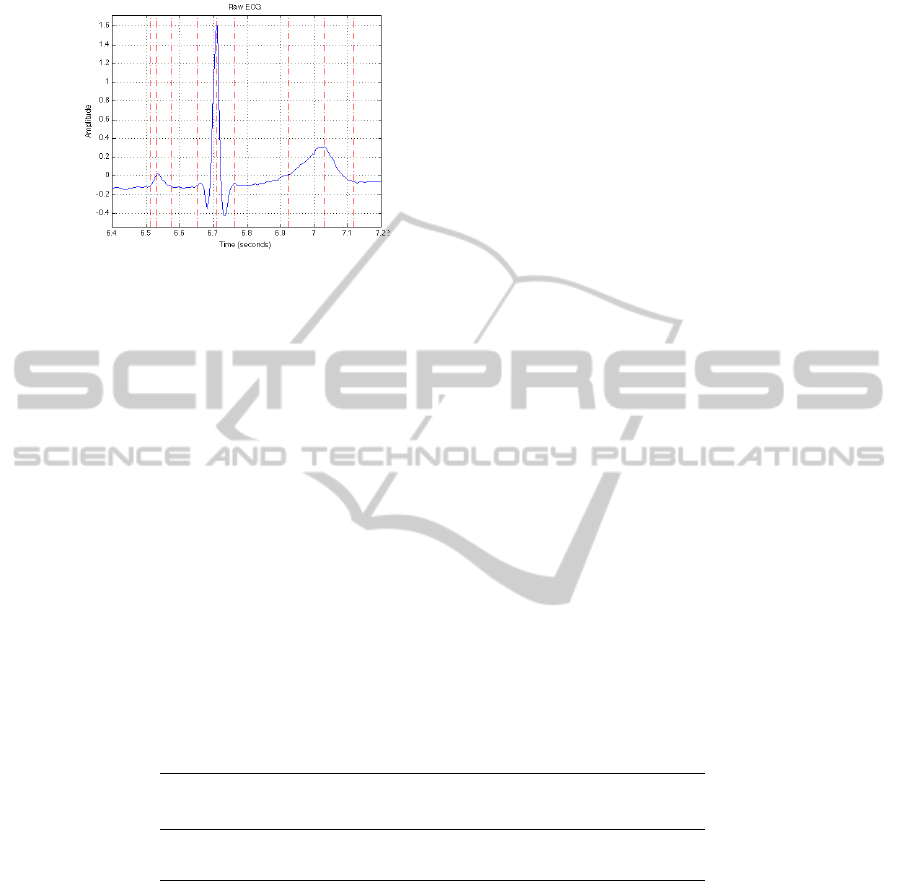
Table 1. Error (mean and standard deviation), in milliseconds, produced by the automatic seg-
mentation system, for the specific fiducial points and intervals.
P
on
P P
end
Q R S
µ 21.98 13.06 8.54 13.16 12.34 11.81
σ 21.06 4.12 5.98 11.61 3.20 3.01
T
on
T T
end
QT RR QT
c
µ 14.45 11.42 11.25 10.44 1.76 11.18
σ 20.25 21.53 7.71 10.90 2.07 12.07
Fig. 2. Segmentation results for one beat on the
raw signal.
Fig. 3. Segmentation results for one beat on the
processed signal.
that our method is promising concerning the improvement of the precision of such au-
tomatic segmentation systems. We must highlight that the proposed algorithm relies on
the analysis of a single signal which greatly reduces the refinement possibilities when
additional signals are considered. Furthermore, regarding the duration of the QT, RR
and corrected QT intervals, the obtained results show good possibilities of application
in an automatic segmentation system. In any case the system’s segmentation accuracy
showed enough reliability to robustly feed the following classification module.
To evaluate the automatic warning generator, based on the described classifier sys-
tem, we trained the system by randomly selecting 80% of dataset records and keeping
the remaining 20% for evaluation purposes. This procedure was repeated 100 times in
order to obtain confidence intervals for the evaluation results. Table 2 shows the classi-
fication confusion matrix as well as the related confidence intervals.
The proposed framework provided promising results despite the very high diversity
of ECG morphologies. The number of correctly predicted situations is high even when
the signal is polluted with Gaussian additive noise (SNR=14dB). In scientific literature
there are very few works that address the described problem with the proposed objec-
tives. Most works are directed to medical instrumentation systems that only grasp a
single pathology whereas few cover broad spectrum classification tasks based on sim-
ple, low resource, wearable devices.
Fig. 2. Segmentation results for one beat on
the raw signal.
Table 1. Error (mean and standard deviation), in milliseconds, produced by the automatic seg-
mentation system, for the specific fiducial points and intervals.
P
on
P P
end
Q R S
µ 21.98 13.06 8.54 13.16 12.34 11.81
σ 21.06 4.12 5.98 11.61 3.20 3.01
T
on
T T
end
QT RR QT
c
µ 14.45 11.42 11.25 10.44 1.76 11.18
σ 20.25 21.53 7.71 10.90 2.07 12.07
Fig. 2. Segmentation results for one beat on the
raw signal.
Fig. 3. Segmentation results for one beat on the
processed signal.
that our method is promising concerning the improvement of the precision of such au-
tomatic segmentation systems. We must highlight that the proposed algorithm relies on
the analysis of a single signal which greatly reduces the refinement possibilities when
additional signals are considered. Furthermore, regarding the duration of the QT, RR
and corrected QT intervals, the obtained results show good possibilities of application
in an automatic segmentation system. In any case the system’s segmentation accuracy
showed enough reliability to robustly feed the following classification module.
To evaluate the automatic warning generator, based on the described classifier sys-
tem, we trained the system by randomly selecting 80% of dataset records and keeping
the remaining 20% for evaluation purposes. This procedure was repeated 100 times in
order to obtain confidence intervals for the evaluation results. Table 2 shows the classi-
fication confusion matrix as well as the related confidence intervals.
The proposed framework provided promising results despite the very high diversity
of ECG morphologies. The number of correctly predicted situations is high even when
the signal is polluted with Gaussian additive noise (SNR=14dB). In scientific literature
there are very few works that address the described problem with the proposed objec-
tives. Most works are directed to medical instrumentation systems that only grasp a
single pathology whereas few cover broad spectrum classification tasks based on sim-
ple, low resource, wearable devices.
Fig. 3. Segmentation results for one beat on
the processed signal.
results of the other two methods, unless for the onset of the P wave. This fact suggests
that our method is promising concerning the improvement of the precision of such au-
tomatic segmentation systems. We must highlight that the proposed algorithm relies on
the analysis of a single signal which greatly reduces the refinement possibilities when
additional signals are considered. Furthermore, regarding the duration of the QT, RR
and corrected QT intervals, the obtained results show good possibilities of application
in an automatic segmentation system. In any case the system’s segmentation accuracy
showed enough reliability to robustly feed the following classification module.
To evaluate the automatic warning generator, based on the described classifier sys-
tem, we trained the system by randomly selecting 80% of dataset records and keeping
the remaining 20% for evaluation purposes. This procedure was repeated 100 times in
order to obtain confidence intervals for the evaluation results. Table 2 shows the classi-
fication confusion matrix as well as the related confidence intervals.
Table 2. Confusion matrix for cardiac function classifier. (Values are in percentage and the num-
ber of actual occurrences in the database was used as reference for the calculation. The two
columns on the right represent the actual situations as found on the reference dataset.)
QTdb QTdb noise corrupted
Predicted Normal Not-Normal Normal Not-Normal
Normal 77.3 ± 8.3% 20.6 ± 6.2% 75.2 ± 8.8% 23.9 ± 8.1%
Not-Normal 22.7 ± 8.3% 79.4 ± 6.2% 24.8 ± 8.8% 76.1 ± 8.1%
The proposed framework provided promising results despite the very high diversity
of ECG morphologies. The number of correctly predicted situations is high even when
the signal is polluted with Gaussian additive noise (SNR=14dB). In scientific literature
there are very few works that address the described problem with the proposed objec-
tives. Most works are directed to medical instrumentation systems that only grasp a
single pathology whereas few cover broad spectrum classification tasks based on sim-
ple, low resource, wearable devices.
The number of false negatives and false positives is an issue for real users because
20