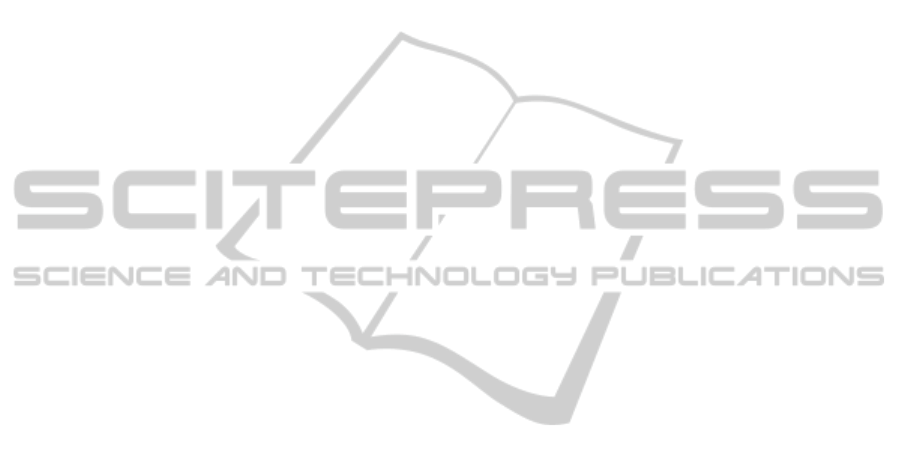
4 Conclusions
Among segmentation algorithms, region growing highly depends on where the grow-
ing process starts and how to control it in order to avoid over growing to homogenous
neighboring areas [12]. There for, we addressed the problem of blind segmentation
and introduced an improved segmentation technique on images of Computed Tomo-
graphy (CT) using images of Positron Emission Tomography (PET). We used the
hotspot data provided by PET image as reference points to start the growing process
in SRG and also as a measure to select segmentation fractions in watershed.
It was taken into consideration to introduce automated segmentation methods
which result in less errors and best performance. The results were compared by defin-
ing three fidelity criteria from segmentation errors to time consumption.
Methods like using boundary maximum gray value and region maximum gray
value had been introduced for certain application and not for segmenting CT images
specifically. We tried to see whether with some modifications and applying data ac-
quired from PET image they can be used for segmenting CT as well. The results sup-
port the fact that their accuracy is not acceptable in contrast with other segmentation
techniques used throughout this paper.
Acknowledgements
The authors would like to thank Department of Nuclear Medicine, Ospedale Santa
Corona, Pietra Ligure, Italy under research collaboration “Manifestation of Extra-
pulmonary Tuberculosis infection on FDG PET CT” in association with Ospedale
Niguarda Ca'Granda, Milano, Italy, for sharing their experience and images with us.
References
1. J. Fan, D. K. Y. Yau, A. K. Elmagarmid and W. G. Aref, "Automatic image segmentation
by integrating color-edge extraction and seeded region growing," IEEE T. Image Process.,
vol. 10; 10, pp. 1454-1466, 2001.
2. J. Fan, G. Zeng, M. Body and M. Hacid, "Seeded region growing: an extensive and com-
parative study," Pattern Recog. Lett., vol. 26, pp. 1139-1156, 6. 2005.
3. A. Mehnert and P. Jackway, "An improved seeded region growing algorithm", Pattern
Recog. Lett., vol. 18, pp. 1065-1071, 10. 1997.
4. R. Adams and L. Bischof, "Seeded region growing," IEEE T. Pattern. Anal., vol. 16, pp.
641-647, 1994.
5. R. C. Gonzalez, R. E. Woods and S. L. Eddins, Digital Image Processing using MATLAB.
Prentice-Hall, Inc. Upper Saddle River, NJ, USA, 2003.
6. S. A. Hojjatoleslami and J. Kittler, "Automatic detection of calcification in mammograms,"
in Image Processing and its Applications, 1995., Fifth International Conference on, 1995,
pp. 139-143.
7. I. Avazpour, M. I. Saripan, A. J. Nordin and R. S. A. Raja Abdullah, "Segmentation of
Extrapulmonary Tuberculosis Infection Using Modified Automatic Seeded Region Grow-
ing," Biol. Proc. Online.
80