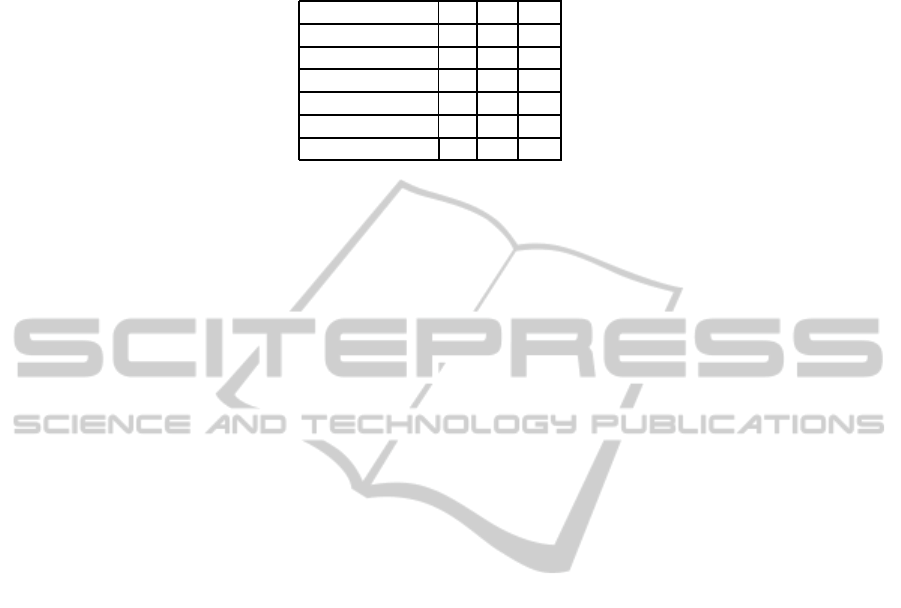
Table 2. Comparisons of different methods for false positive reduction.
TPR FPR ROIs
Li et al. 1% 56% 25
Angelini et al. 13% 38% 69
Tourassi et al. 10% 65% 1820
Varela et al. 22% 85% 120
Masotti et al. 0% 30% 884
Proposed method 0% 58% 469
a two classes classification problem, with the aim to assign to each suspicious ROI a
degree of abnormality and a degree of not abnormality, thus reducing the whole number
of ROIs to be presented to the radiologist. A large set of features have been extracted
from the ROIs identified by an automatic identification algorithm proposed by the au-
thors. Then, the selected features have been used to train a fuzzy classifier, properly
structured for medical applications. Different working points have been considered so
that the radiologist could choose the best tradeoff between sensitivity and false positive
per image, according to the clinical application.
References
1. M. Bazzocchi and F. Mazzarella, “CAD systems for mammography: a real opportunity? A
review of the literature,” http://www.springerlink.com/content/x3157r8u72196h45/
fulltext.pdf/, 2006.
2. A. Oliver, “A new approach to the classification of mammographic masses and normal breast
tissue,” in Proceedings of the 18th International Conference on Pattern Recognition, 2006,
vol. 4, pp. 707 – 710.
3. J. Wei, H-P. Chan, B. Sahiner, C. Zhou, and L. M. Hadjiiski, “Computer-aided detection
of breast masses on mammograms: Dual system approach with two-view analysis,” Med.
Phys., vol. 36, no. 10, pp. 4451 – 4460, 2009.
4. C. Balleyguier, S. Ayadi, K. Van Nguyen, D. Vanel, C. Dromain, and R. Sigal, “BIRADS
classification in mammography,” European Journal of Radiology, vol. 61, pp. 192–194,
2007.
5. University of South Florida, “DDSM: Digital database for screening mammography,”
http://marathon.csee.usf.edu/Mammography/Database.html, 2000.
6. A. Mencattini, G. Rabottino, M. Salmeri, and R. Lojacono, “Assessment of a breast masses
identification procedure using an iris detector,” IEEE Transactions on Instrumentation and
Measurement, in press.
7. K. I. Laws, “Laws’ texture measures,” http://www.ccs3.lanl.gov/ kelly/ZTRANSITION/
notebook/laws.shtml, 2001.
8. R. M. Haralick, “Statistical and structural approaches to texture,” Proceedings of the IEEE,
vol. 67, no. 5, pp. 786 – 804, 1979.
9. M. Masotti, N. Lanconelli, and R. Campanini, “Computer aided mass detection in mammog-
raphy: False positive reduction via gray scale invariant ranklet texture features,” Medical
Physics, vol. 36, no. 2, 2009.
10. F. Smeraldi, “Ranklets: Orientation selective non-parametric features applied to face de-
tection,” in 16th International Conference on Pattern Recognition, 2002, vol. 3, pp. 351 –
359.
24