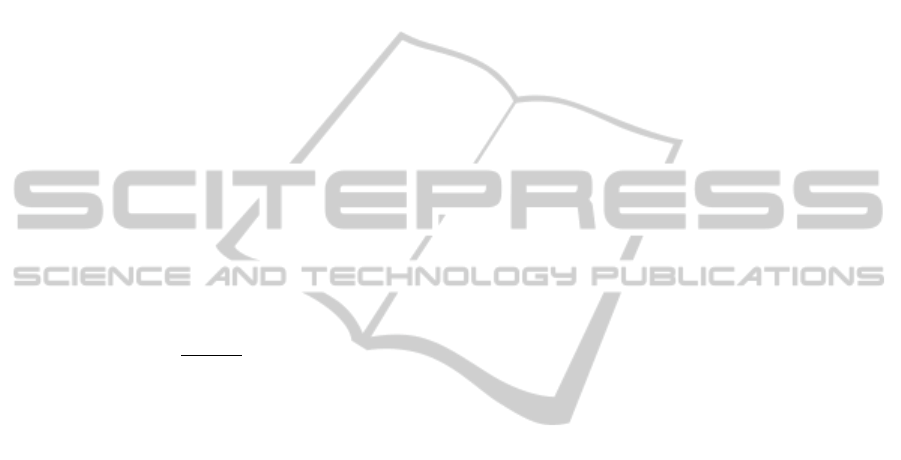
we did not have other similar systems as a
benchmark for these unique characteristics.
• We did not support the implementation and
administration of QSIA to such an extent that builds
significant trust and users' high expected utility, as
could be done with larger resources (Gefen, 2004).
ACKNOWLEDGEMENTS
This completed research report was guided by Prof.
Sheizaf Rafaeli to whom I own much of my humble
research qualifications.
The QSIA system was designed by Dr. Eran
Toch and Mr. Danny Shaham from the Research
Center for the Study of the Information Society
(INFOSOC at: http://infosoc.haifa.ac.il), under the
guidance of Dr. Miri Barak with the support from
the Caesarea Edmond Benjamin de Rothschild
Foundation Institute for Interdisciplinary
Application of Computer Science at the University
of Haifa.
Finally, it must be noted (again) that throughout
the research, we do not claim to prove causality;
rather, we are aiming at relation
establishment.
REFERENCES
Bacon, L. D. (1995). Linking attitudes and behavior -
summary of literature. Paper presented at the
American Marketing Association/Edison Electric
Institute Conference, Chicago, Il.
Barak, M. & Rafaeli, S. (2004). Online question-posing
and peer-assessment as means for web-based
knowledge sharing in learning, International Journal
of Human-Computer Studies, 61(1), 84-103.
Bradley, J. V. (1968). Distribution-Free Statistical Tests.
New Jersey: Prentice-Hall.
Breese, J. S., Heckerman, D., & Kadie, C. (1998).
Empirical analysis of predictive Algorithms for
collaborative filtering. Proceedings of the Fourteenth
Conference on Uncertainty in Artificial Intelligence.
Madison, 43-52.
Cosley, D., Lam, S. K., Albert, I., Konstan, A. J. & Riedl,
J. (2003). Is seeing believing?: how recommender
system interfaces affect users' opinions. Proceedings
of the SIGCHI conference on Human factors in
computing systems, Ft. Lauderdale, Florida, 5(1), 585-
592. New York: ACM Press.
Festinger, L. (1954). A theory of social comparison
processes. Human Relations, 7, 114-140.
Fisher, D., Hildrum, K., Hong, J., Newman, M., Thomas,
M., & Vuduc, R. (2000). SWAMI: A framework for
collaborative filtering algorithm development and
evaluation, Research and Development in Information
Retrieval, 366-368.
Freedman, S. G. (1998). Asking software to recommend a
good book. The New York Times, 1998, June 20.
Gefen, D. (2004). What Makes ERP Implementation
Relationships Worthwhile: Linking Trust Mechanisms
and ERP Usefulness, Journal of Management
Information Systems, 21(1), 275-301.
Goldberg, K., Roeder, T., Gupta, D., & Perkins, C. (2000).
Eigentaste: A Constant Time Collaborative Filtering
Algorithm (Technical Report M00/41).
Herlocker, J. (2000). Understanding and improving
automated collaborative filtering systems.
Unpublished Ph.D. dissertation, UMI Order Number:
AAI9983577, University of Minnesota.
Herlocker, J., Konstan, J., Borchers, A., & Riedl, J.
(1999). An Algorithmic Framework for Performing
Collaborative Filtering, Research and Development in
Information Retrieval (pp. 230-237).
Herlocker, J., Konstan, A. J., Terveen, G. L. & Riedl, J.
(2004). Transactions on Information Systems.
Communications of the ACM, 22(1), 5-53. New York:
ACM Press.
Hosmer, D. W. & Lemeshow, S. (2000). Applied Logistic
Regression. New York: Wiley.
Kalman, Y. M. & Rafaeli, S. (2005). Email Chronemics:
Unobtrusive Profiling of Response Times,
Proceedings of the 38th International Conference on
System Sciences, HICSS 38, 2005. Big Island,
Hawaii. Ralph H. Sprague, (Ed.), 108. Available
online:
http://sheizaf.rafaeli.net/publications/KalmanRafaeliC
hronemics2005Hicss38.pdf
Karypis, G. (2000). Evaluation of Item-Based Top-N
recommendation algorithms (CS-TR-00-46).
Minneapolis: University of Minnesota, Department of
Computer Science and Army HPC Research Center.
Kerlinger, F. N. (1986). Foundations of behavioral
research. Orlando: Holt, Rinehart and Winston, Inc.
Konstan, J., Miller, B. N., Malt, D., Herlocker, J., Gordon,
L. R., & Riedl, J. (1997). GroupLens: applying
collaborative filtering to Usenet news.
Communications of the ACM, 40(3), 77-87.
Konstan, J., & Riedl, J. (1999). Research Resources for
Recommender Systems. Paper presented at the ACM
SIGIR: Workshop on Recommender Systems-
Algorithms and Evaluation, University of California,
Berkeley.
Minard, R. D. (1952). Race relations in the Pocahontas
Coal Field. Journal of Social Issues, 8, 29-44.
Moon, Y. (1998). The Effects of Distance in Local versus
Remote Human-Computer Interaction. In proceedings
of the CHI 98', Los Angeles, CA. 103-108.
Moon, Y., & Nass, C. (1998). Are computers scapegoats?
Attributions of responsibility in human-computer
interaction. International Journal of Human-Computer
Studies, 49, 79-94.
Pescovitz, D. (2000). Accounting for taste. Scientific
American, June 2000.
THE STONE AGE IS BACK - HCI Effects on Recommender Systems
269