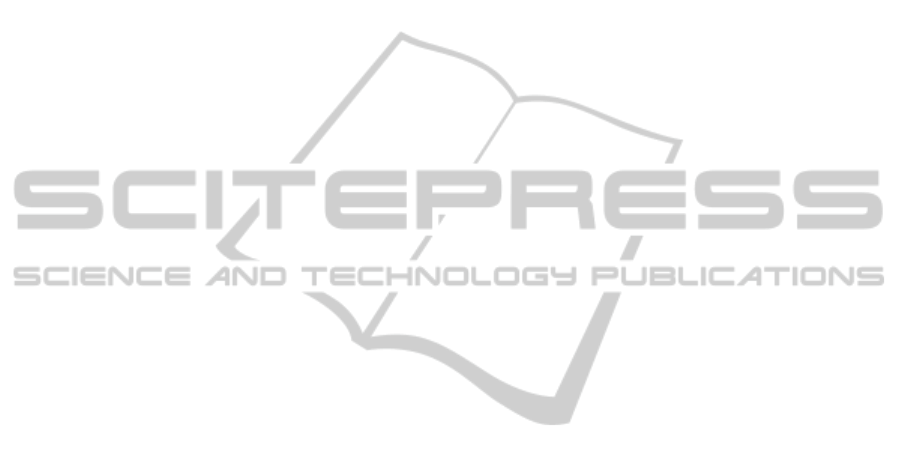
scheme have been tested with a small dataset, the obtained accuracy of 70% seems to
be promising for automatic classification of breast masses. These preliminary results
have opened up new strategies for the development of computer-aided tools, based on
the sparse representation framework, for mammographic diagnosis. Further work in-
cludes to perform extensive validations with bigger datasets and to include other breast
mass characteristics, like shape, margin and density.
References
1. American Cancer Society: American Cancer Statistics. (2007) Updated: September 2, 2008.
2. American College of Radiology (ACR): Illustrated Breast Imaging Reporting and Data Sys-
tem (BI-RADS). ACR (1998)
3. R. Bird, T. Wallace, and B. Yankaskas, Analysis of cancers missed at screening mammogra-
phy, Radiology 178 (1992), 234–247.
4. S. Buseman, J. Mouchawar, N. Calonge, and T. Byers., Mammography screening matters for
young women with breast carcinoma., Cancer 97 (2003), 352–358.
5. A. M. Bruckstein, D. L. Donoho, and M. Elad., From Sparse Solutions of Systems of Equa-
tions to Sparse Modeling of Signals and Images., SIAM Review 51 (2009), 34–81.
6. H. D. Cheng, X. J. Shi, R. Min, L. M. Hu, X. P. Cai, H. N. Du. Approaches for automated
detection and classification of masses in mammograms., Pattern Recognition 39 (2006), 646–
668
7. D. Gur, J. S Stalder, L. A. Hardesty, B. Zheng, J. H. Sumkin, D. M Chough, B. E. Shindel,
and H. E. Rockette, Computer-aided detection performance in mammographic examination
of masses: assessment., Radiology 233 (2004), 418–423.
8. M. Heath, K. Bowyer, D. Kopans, R. Moore, and W. P. Kegelmeyer, The digital database
for screening mammography, in Proceedings of the Fifth International Workshop on Digital
Mammography, Medical Physics Publishing M.J. Yaffe, ed (2001), 212–218.
9. J. Herredsvela, K. Engan, T. O. Gulsrud, and K. Skretting, Detection of masses in mam-
mograms by watershed segmentation and sparse representation using learned dictionaries.
(paper in pdf-format), Proceedings NORSIG (2005), 35–40.
10. H. Kim and J. Kim, Region-based shape descriptor invariant to rotation, scale and transla-
tion., Signal Proc.: Image Communication 16 (2000), 87–93.
11. S. G. Mallat and Z. Zhang, Matching pursuits with time-frequency dictionaries, IEEE Trans-
actions on signal processing 41 (1993), no. 12, 3397–3415.
12. R. M. Nishikawa, Current status and future directions of computer-aided diagnosis in mam-
mography, Computerized Medical Imaging and Graphics 31 (2007), 224–235.
13. F. Narvaez, G. Diaz, E. Romero, Automatic BI-RADS description for mammographic
masses, IWDM2010 Digital Mammographhy, LNCS 6136 (2010), 673–681.
14. B. A. Olshausen, Principles of image representation in visual cortex, pp. 1603–1615, MIT
Press, 2003.
15. N. A. Rosa, J. C. Felipe, A. J. Traina, R. M Rangayyan, and P. M. Azevedo-Marques, Using
relevance feedback to reduce the semantic gap in content-based image retrieval of mammo-
graphic masses., Conf Proc IEEE Med Biol Soc (2008), 406–409.
16. Y. Tao, S. B. Lo, M. T. Freedman, and J. Xuan, A preliminary study of content-based mam-
mographic masses retrieval, Proc SPIE 6514 (2007), 65141Z.
17. K. Verma and J. Zakos, A computer-aided diagnosis system for digital mammograms based
on fuzzy-neural and feature extraction techniques, IEEE Transactions on Information Tech-
nology in Biomedicine 16 (2002), 219–223.
32