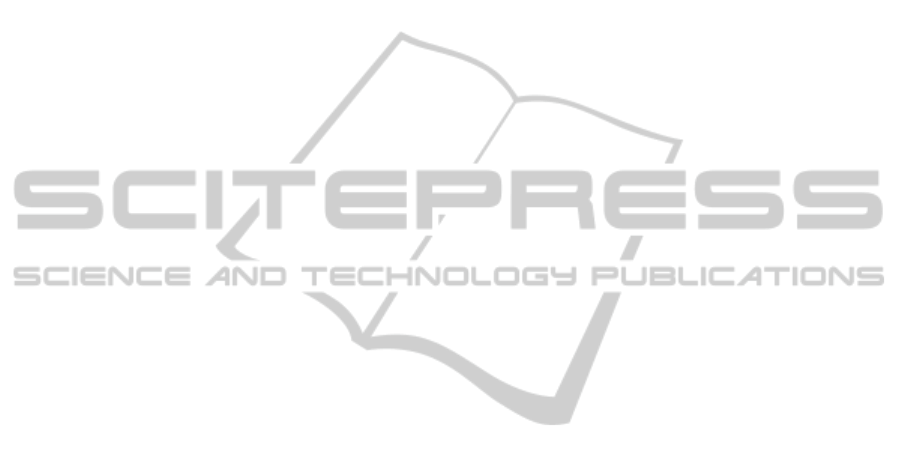
insights into explaining and predicting complaint
behaviors in the online shopping.
Several important practical implications arise
from our findings. The survey result reported a high
proportion of online shoppers (over 65%) that has the
experience of a service or produce failure in online
shopping. Consumers not only want to express
negative feelings and to seek for redress, but also
want to give advices for improving the process of
products and services offered in online stores.
Therefore, online stores should take account of
consumer’s complaints, and pay attention on the
communication channel between online stores and
consumers. Specifically, distributive and interactional
justices and ECM-based components have indicated
well as the underlying drivers in determining
complaint intentions. The communication channel
should be considered from both marketing and
technological aspects and can be effectively
improved accordingly.
For marketing aspect, the products and treatments
of online stores play an important role in consumer’s
perceived justice. Online stores should maintain and
improve the quality of products and treat consumers
fairly with the implementation of customer
relationship management. For technological aspect,
online stores need to improve front-end and back-end
mechanisms simultaneously. In the front-end part,
online stores should implement new and useful
information and communication technologies, design
user-friendly system interface, build effective
searching engines, and develop easy understanding
form of layout. In the back-end part, online stores
can provide useful customized information to fulfill
consumer requirements and let consumers manage
their orders, payments, and deliveries in a more
efficient way.
Finally, a limitation of this study showed that
approximately 65% and 35% of the respondents are
female and male respectively. The result may not
reflect properly the regular population distribution of
gender and cause a potential bias against the current
findings. However, in fact, women are more likely to
do online shopping than man and this would, in
essence, reflect the actual situation.
REFERENCES
Alexander, S., Ruderman, M., 1987. The role of procedural
and distributive justice in organizational behavior.
Social Justice Research 1 (2), 177-198.
Bhattacherjee, A., 2001. Understanding information
systems continuance: an expectation-confirmation
model. MIS Quarterly 25 (3), 351-370.
Bies, R. J., Moag, J. F., 1986. Interactional justice:
communication criteria of fairness. Research on
Negotiations in Organizations 1 (1), 43-55.
Blodgett, J. G., Granbois, D. H., Walters, R. G., 1993. The
effects of perceived justice on complainants' negative
word-of-mouth behavior and repatronage intentions.
Journal of Retailing 69 (4), 399-428.
Blodgett, J. G., Hill, D. J., Tax, S. S., 1997. The effects of
distributive, procedural, and interactional justice on
postcomplaint behavior. Journal of Retailing 73 (2),
185-210.
Breazeale, M., 2009. Word of mouse. International Journal
of Market Research 51 (3), 297-318.
Chea, S., Luo, M. M., 2008. Post-adoption behaviors of
e-service customers: the interplay of cognition and
emotion. International Journal of Electronic Commerce
12 (3), 29-56.
Chiu, C.-M., Lin, H.-Y., Sun, S.-Y., Hsu, M.-H., 2009.
Understanding customers' loyalty intentions towards
online shopping: an integration of technology
acceptance model and fairness theory. Behaviour &
Information Technology 28 (4), 347-360.
Clemmer, E. C., 1993. An investigation into the
relationships of justice and customer satisfaction with
services. In: Cropanzano, R. (Ed.), Justice in the
Workplace: Approaching Fairness in Human Resources
Management. Erlbaum, Hillsdale, NJ.
Colquitt, J. A., Wesson, M. J., Porter, C. O. L. H., Conlon,
D. E., Ng, K. Y., 2001. Justice at the millennium: a
meta-analytic review of 25 years of organizational
justice research. Journal of Applied Psychology 86 (3),
425-445.
Davis, F. D., Bagozzi, R. P., Warshaw, P. R., 1989. User
acceptance of computer technology: a comparison of
two theoretical models. Management Science 35 (8),
982-1003.
Finn, A., Wang, L., Frank, T., 2009. Attribute perceptions,
customer satisfaction and intention to recommend
e-services. Journal of Interactive Marketing 23 (3),
209-220.
Gefen, D., Karahanna, E., Straub, D. W., 2003. Trust and
TAM in online shopping: an integrated model. MIS
Quarterly 27 (1), 51-90.
Hair, J. F., Black, W. C., Babin, B. J., Anderson, R. E.,
Tatham, R. L., 2006. Multivariate Data Analysis, sixth
ed. Pearson Education Inc, New Jersey.
Kang, Y. S., Hong, S., Lee, H., 2009. Exploring continued
online service usage behavior: the roles of self-image
congruity and regret. Computers in Human Behavior
25 (1), 111-122.
Kim, S. S., Son, J.-Y., 2009. Out of dedication or
constraint? A dual model of post-adoption phenomena
and its empirical test in the context of online services.
MIS Quarterly 33 (1), 49-70.
Liu, R. R., McClure, P., 2001. Recognizing cross-cultural
differences in consumer complaint behavior and
WEBIST 2011 - 7th International Conference on Web Information Systems and Technologies
478