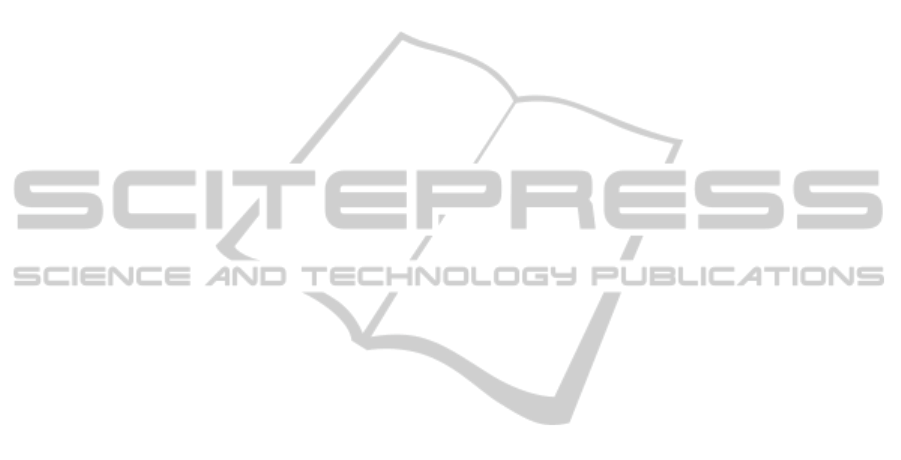
6 FUTURE RESEARCH
The definition of the KnoVA reference model and
the DSL based implementation is the first step in the
KnoVA approach. Currently we are working on a for-
mal description of the DSL based system states, to
create a visualization state process model. The ba-
sic idea is, that knowledge is applied implicitly by
the users interaction, which leads to a change in the
system states. In addition to this, a rule language for
knowledge extraction has to be defined. This work
being done, the complete KnoVA approach for knowl-
edge based visual analytics applications can be used
to create visual analytics applications that allow for
an extraction of implicit expert knowledge.
An open research questions is whether the knowl-
edge extraction can take place automatically or
whether user interaction is necessary. Another open
question is how the knowledge can be applied to other
tasks. One possibility to use the knowledge in a dif-
ferent context can be the automatic generation of pos-
sible next step suggestions or the generation of sug-
gestions for other suitable visualizations. This will
address the third challenge identified above.
REFERENCES
Aigner, W., Bertone, A., Miksch, S., Tominski, C., and
Schumann, H. (2007). Towards a conceptual frame-
work for visual analytics of time and time-oriented
data. In WSC ’07: Proceedings of the 39th conference
on Winter simulation, pages 721–729, Piscataway, NJ,
USA. IEEE Press.
Brunk, C., Kelly, J., and Kohavi, R. (1997). Mineset: An in-
tegrated system for data mining. In KDD, pages 135–
138.
Chi, E. H. (2002). Expressiveness of the data flow and data
state models in visualization systems. In AVI ’02: Pro-
ceedings of the Working Conference on Advanced Vi-
sual Interfaces, pages 375–378, New York, NY, USA.
ACM.
Chuah, M. C., Roth, S. F., Mattis, J., and Kolojejchick, J.
(1995). Sdm: Selective dynamic manipulation of vi-
sualizations. In ACM Symposium on User Interface
Software and Technology, pages 61–70.
Eick, S. G. (2009). Data visualization software — advizor
solutions. ”‘Website”’.
Elmqvist, N., Stasko, J., and Tsigas, P. (2008).
Datameadow: a visual canvas for analysis of large-
scale multivariate data. Information Visualization,
7(1):18–33.
Fl
¨
oring, S. and Hesselmann, T. (2010). Tap: Towards vi-
sual analytics on interactive surfaces. In Collabora-
tive Visualization on Interactive Surfaces - CoVIS ’09,
number 2010-2, pages 9–12, Munich, Germany. LMU
Media Informatics. Technical Report.
Haber, R. and McNabb, D. A. (1990). Visualization id-
ioms: A conceptual model for scientific visualization
systems. In Visualization in Scientific Computing.
Hinneburg, A., Keim, D. A., and Wawryniuk, M. (2003).
Hd-eye - visual clustering of high dimensional data: a
demonstration. IEEE Computer Graphics and Appli-
cations, 19(5):735–755.
Keim, D. A. (2001). Visual exploration of large data sets.
Commun. ACM, 44(8):38–44.
Keim, D. A., Mansmann, F., Stoffel, A., and Ziegler, H.
(2009). Visual analytics. In Encyclopedia of Database
Systems. Springer.
Levendovszky, T., Lengyel, L., Mezei, G., and Charaf, H.
(2005). A systematic approach to metamodeling envi-
ronments and model transformation systems in vmts.
In Electronic Notes in Theoretical Computer Science,
pages 65–75.
Liu, Z., Stasko, J., and Sullivan, T. (2009). Selltrend:
Inter-attribute visual analysis of temporal transaction
data. IEEE Transactions on Visualization and Com-
puter Graphics, 15(6):1025–1032.
Meister, J., Rohde, M., Appelrath, H.-J., and Kamp, V.
(2003). Data-warehousing im gesundheitswesen. it
- Information Technology, 45(4):179–185.
Pfitzner, D., Hobbs, V., and Powers, D. M. W. (2003). A
unified taxonomic framework for information visual-
ization. In Pattison, T. and Thomas, B. H., editors, In-
Vis.au, volume 24 of CRPIT, pages 57–66. Australian
Computer Society.
Tang, D., Stolte, C., and Bosch, R. (2004). Design choices
when architecting visualizations. Information Visual-
ization, 3(2):65–79.
Tobias, M., Isenberg, P., and Carpendale, S. (2009). Lark:
Coordinating co-located collaboration with informa-
tion visualization. IEEE Transactions on Visualization
and Computer Graphics, 15(6):1065–1072.
Valiati, E. R. A., Pimenta, M. S., and Freitas, C. M. D. S.
(2006). A taxonomy of tasks for guiding the evalu-
ation of multidimensional visualizations. In BELIV
’06: Proceedings of the 2006 AVI workshop on BE-
yond time and errors, pages 1–6, New York, NY,
USA. ACM.
Wehrend, S. and Lewis, C. (1990). A problem-oriented
classification of visualization techniques. In VIS ’90:
Proceedings of the 1st conference on Visualization
’90, pages 139–143, Los Alamitos, CA, USA. IEEE
Computer Society Press.
Wenzel, S., Bernhard, J., and Jessen, U. (2003). Visual-
ization for modeling and simulation: a taxonomy of
visualization techniques for simulation in production
and logistics. In Chick, S. E., Sanchez, P. J., Ferrin,
D. M., and Morrice, D. J., editors, Winter Simulation
Conference, pages 729–736. ACM.
KNOVA: INTRODUCING A REFERENCE MODEL FOR KNOWLEDGE-BASED VISUAL ANALYTICS
235