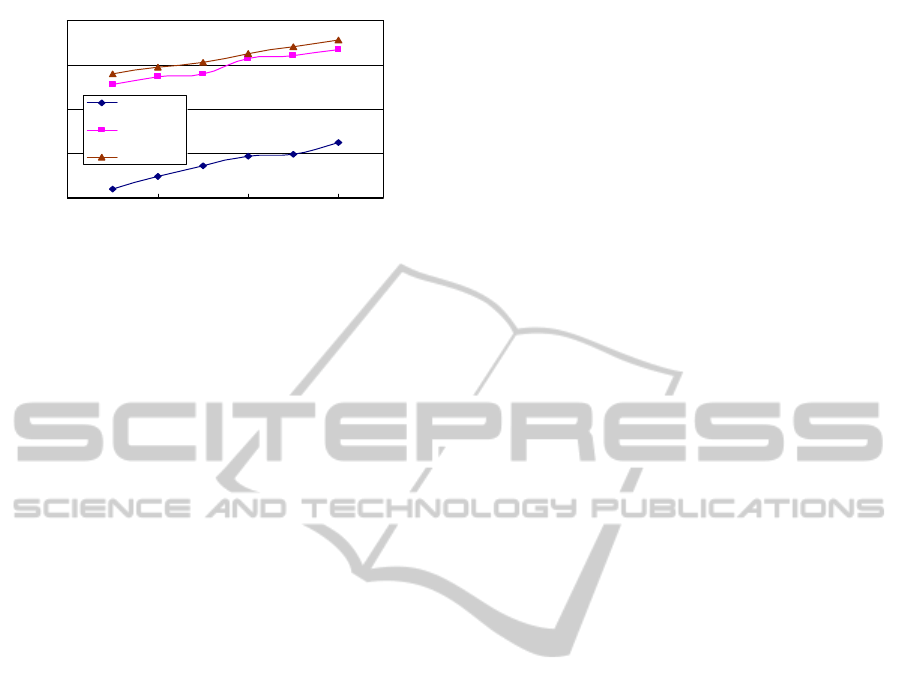
AR
63%
68%
73%
78%
83%
15 25 35 45
No. ei
envectors
Rec. rate
PCA
PCA+Vs
PCA+Vs+MQD
Figure 5: PCA based face recognition on the AR.
The above three experiments are repeated on an
LDA based platform instead of PCA. We first
project the grey level face images onto an
eigenspace. All the training and testing sets as well
as generated samples remain unchanged. Similar
improvements in recognition rates are obtained.
4 DISCUSSIONS
AND CONCLUSIONS
We propose an efficient method for matching facial
images and use this method for generating a large
number of additional training samples by matching
and morphing between pairs of real images. The
matching algorithm does not require a one-to-one
correspondence between the set of feature points in
the pair of images. The method is efficient since it
does not involve face modeling and is entirely based
on 2D images. Experiments show that the
recognition rates for PCA and LDA based face
recognition systems are both improved by a large
margin, ranging from 8% to 17%. Moreover, with
the large number of generated samples for training,
more sophisticated statistical classifiers for face
recognition can be used. Experiments show that the
MQDF1 classifier generally gives a higher
recognition rate than the NN-classifier.
A limitation is that it cannot match two images in
which the face orientation is quite different, because
it only relies on 2D information. Also, the
intermediate images generated are not perfect.
Nevertheless the quality is already good enough to
serve as additional training samples.
REFERENCES
Beier, T., & Neely, S. (1992). Feature-Based Image
Metamorphosis. Siggraph 92 : Conference
Proceedings, 26, 35-42.
Cootes, T. F., Edwards, G. J., & Taylor, C. J. (2001).
Active appearance models. Ieee Transactions on
Pattern Analysis and Machine Intelligence, 23(6),
681-685.
Durbin, R., & Willshaw, D. (1987). An Analogue
Approach to the Travelling Salesman Problem Using
an Elastic Net Method. Nature, 326(6114), 689-691.
Karam, H., Hassanien, A., & Nakajima, M. (2001).
Feature-based image metamorphosis optimization
algorithm. Vsmm 2001: Seventh International
Conference on Virtual Systems and Multimedia,
Proceedings, 555-564.
Kimura, F., Takashina, K., Tsuruoka, S., & Miyake, Y.
(1987). Modified Quadratic Discriminant Functions
and the Application to Chinese Character-Recognition.
Ieee Transactions on Pattern Analysis and Machine
Intelligence, 9(1), 149-153.
Lanitis, A., Taylor, C. J., & Cootes, T. F. (1997).
Automatic interpretation and coding of face images
using flexible models. Ieee Transactions on Pattern
Analysis and Machine Intelligence, 19(7), 743-756.
Li, W. C., Leung, C. H., & Hung, Y. S. (2004). Matching
of uncalibrated stereo images by elastic deformation.
International Journal of Imaging Systems and
Technology, 14(5), 198-205.
Martinez, A. M. (2002). Recognizing imprecisely
localized, partially occluded, and expression variant
faces from a single sample per class. Ieee Transactions
on Pattern Analysis and Machine Intelligence, 24(6),
748-763.
Vetter, T., & Poggio, T. (1997). Linear object classes and
image synthesis from a single example image. Ieee
Transactions on Pattern Analysis and Machine
Intelligence, 19(7), 733-742.
VISAPP 2011 - International Conference on Computer Vision Theory and Applications
674