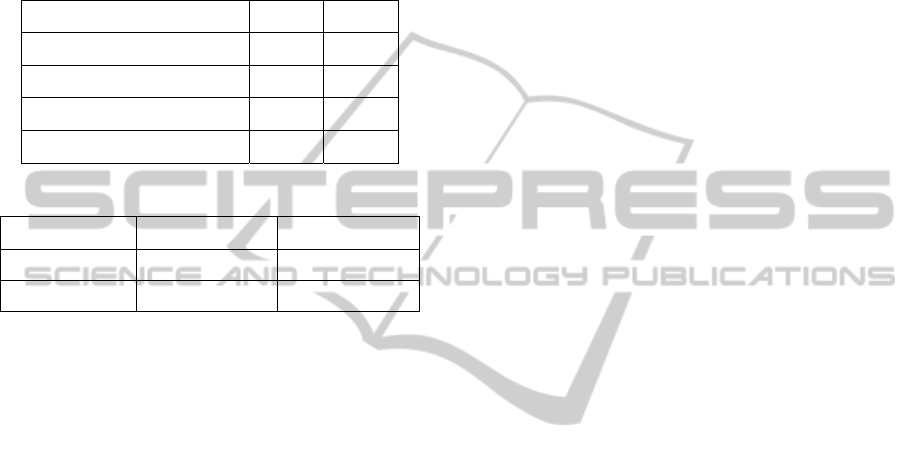
from the Fourier Descriptors, the Precision seems to
drop to 83,33%. This is mainly caused by the fact
that the MHI contour during “Bending” still contains
information of the Walking silhouettes. This extra
information changes the contour of the MHI
contour. This change in contour deteriorates the
results compared with the predefined contours of the
“Bending” en Collapsing actions.
Table 2: Multiple Action Results.
Action Precision Accuracy
Walking -> Fall 100,00% 100,00%
Walking -> Bending 83,33% 91,67%
Bending -> Walking 91,67% 95,83%
Walking - > Bending -> Collapse 83,33% 91,67%
Table 3: Single Action results for (Tao, 2006) method.
Action Precision Accuracy
Walking 80% 88,89%
Falling 75% 85,71%
If we compare our results with the state of the art
we outperform other techniques based on the
bounding box ratio. Table 3 shows the results using
the bounding box ratio principle as used in (Tao et
al, 2005) and (Anderson et al, 2006) on our dataset.
The actions “Walking” and “Falling” are being
detected but with a drop in precision and accuracy,
while the actions “Bending” and “Collapsing”
couldn’t be detected on our dataset at all. Using only
the bounding box ratio proved not to be successful
on our dataset, but since it shows that in some cases
falling and walking can be detected, the combination
of using the bounding box ratio together with our
method can be promising. If we look at the other
methods such as the wearable devices discussed by
(Zhang et al, 2006), we achieve slightly better
performance on a very similar dataset but these
devices have a drawback that patients need to wear
them all the time which is very uncomfortable.
Regarding speed, our system achieves real time
performance.
6 CONCLUSIONS
The research presented in this paper is related to
human fall detection and the detection of different
actions that can be dangerous for elderly people. Our
main goal was to design a system which can work in
real time applications and reduce the implementation
costs by using only one web camera. Our system is
able to detect and distinguish different actions by
using a size and shape information of the motion
history image that characterizes certain action. It
outperforms other methods based on background
subtraction and pose recognition using silhouette
information. It also gives very high fall detection
results, works in real time and is very inexpensive to
implement. For further development we plan to
implement it in an embedded system and test it in
different nursing home environments.
REFERENCES
Anderson, D., Keller, J.M., Skubic, M.; Xi Chen;Zhihai
He, (2006). Recognizing Falls from
Silhouettes. Engineering in Medicine and Biology
Society, EMBS '06, 28th Annual International
Conference of the IEEE , vol., no., pp.6388-6391
Bobick, A. and Davis, J. (2001). The recognition of human
movement using temporal templates. In IEEE
Transactions on Pattern Analysis and Machine
Intelligence, volume23, pages257–267.
Close, J. Ellis, M., Hooper, R., Glucksman, E., Jackson, S.
Swift C. (1999). Prevention of falls in the elderly trial
(PROFET): a randomised controlled trial. Department
of Health Care of the Elderly, Guy's King's, and St
Thomas' School of Medicine, King's College Hospital,
London, UK.
Diraco, G., Leone, A., Siciliano, P. (2010). An active
vision system for fall detection and posture
recognition in elderly healthcare. Design, Automation
& Test in Europe Conference & Exhibition (DATE),
vol., no., pp.1536-1541.
Kannus, P., Sievanen, H., Palvanen, M., Jarvinen, T.,
Parkkari, J. (2005). Prevention of falls and consequent
injuries in elderly people, The Lancet, Volume 366,
Issue 9500, Pages 1885-1893
Nait-Charif, H., McKenna, S.J. (2004). Activity
summarization and fall detection in a supportive home
environment, Pattern Recognition, 2004. Proceedings
of the 17th International Conference on Pattern
Recognition , vol.4, no., pp. 323- 326 .
Tao, J., Turjo, M., Wong, M. F, Wang, M., Tan, Y. P.
(2006). Fall Incidents Detection for Intelligent Video
Surveillance, Information, Communications and
Signal Processing, 2005 Fifth International
Conference on , vol., no., pp.1590-1594.
Zhang, D.S., Lu, J. (2001). Comparative study on shape
retrieval using Fourier descriptors with different
shape signatures, In Proceedings of the International
Conference on Multimedia and Distance Education,
Fargo, ND, USA, pp. 1–9.
Zhang, T., Wang, J., Xuetal, L. (2006). Using wearable
sensor and NMF algorithm to realize ambulatory fall
detection, Lecture Notes in Computer Science,
Advances in Natural Computation, vol. 4222, pp.488-
491.
VISAPP 2011 - International Conference on Computer Vision Theory and Applications
414