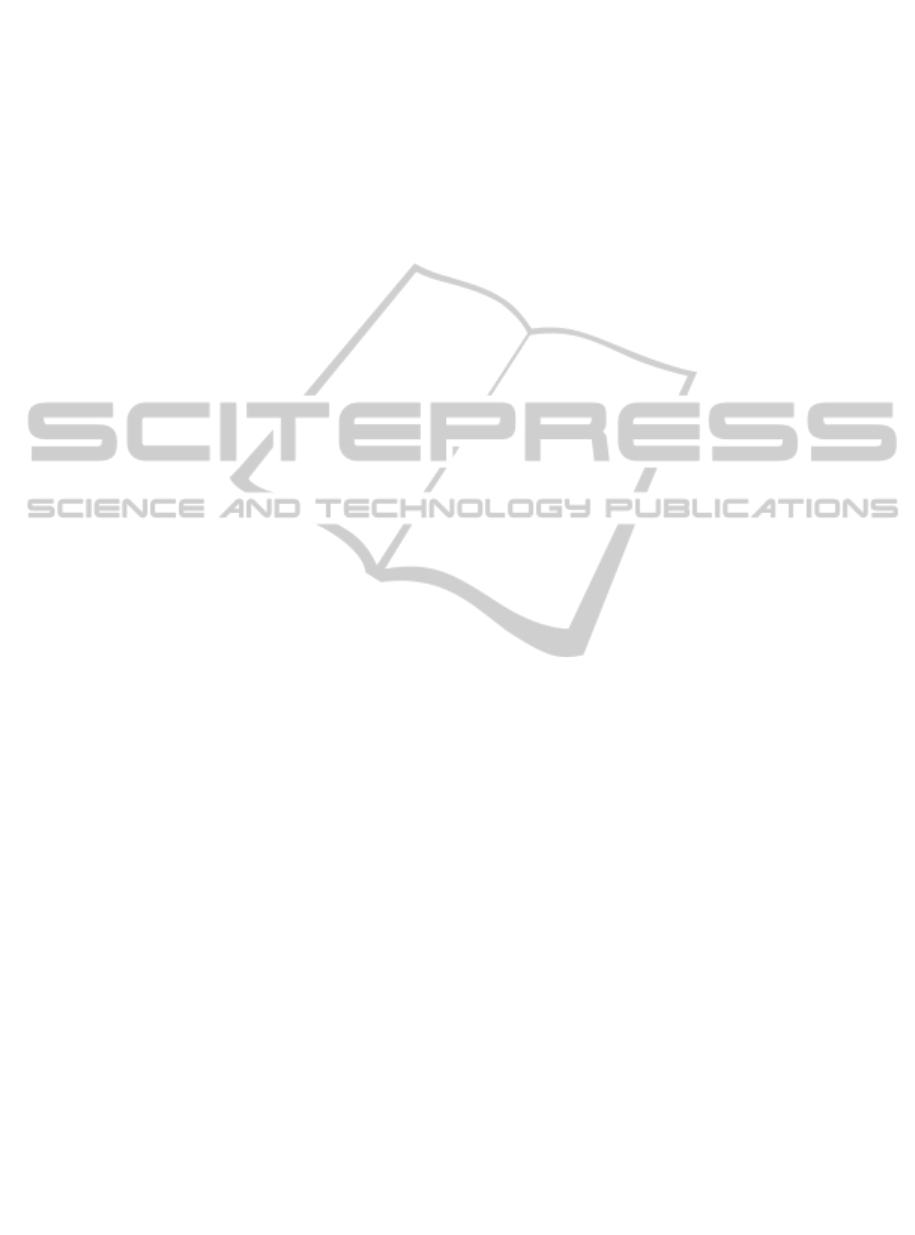
(respectively concave and planar) indicates a good
fit of the method in question for the segmentation of
convex regions (respectively concave and planar).
Our experimental results have shown that the
segmentation techniques adopting non-local shape
properties (Rand Cuts, Norm Cuts, Core Extra and
Shape Diameter) are better than those based on the
local shape properties. We note in particular that
Rand Cuts is the best method to segment convex,
concave and planar regions. Nevertheless,
segmentation by Rand Walks is the least suited for
the segmentation of planar and concave regions. The
RG method is however the less good for segmenting
convex regions.
The results concerning the evaluation of the
convex and concave regions segmentation present
the quality measures the most dispersed
(variance
cnv
= 0.00119277, variance
cnc
= 0.0087033).
This is explained by the variety of the convex and
the concave forms, which can be segmented in
different ways by methods using various criteria.
However, planar regions segmentation methods
evaluation present the similarity measures the most
closest (variance
pln
= 9.6161E-05). Indeed, the planar
regions have the same geometric shape to be
segmented in nearly the same way and it helps to
have very similar results.
Moreover, our results concerning planar regions
show the performance of some algorithms that are
frequently used in CAD (Computer Aided Design)
in the segmentation of such regions. For example,
the method Fit Prim, which is composed of
geometric primitives, such as CAD models, is the
best suited for the segmentation of this type of object
(Attene et al., 2006b).
Thus, the criteria used in each method in the
segmentation process have an influence on the
quality of segmented regions. Indeed, each method
uses some criteria to guide the segmentation process
where the type of extracted regions depends on the
adopted criteria. Therefore, through the
classification phase done before the application of
the evaluation metric, our approach provides better
understanding of the use of these criteria in the mesh
segmentation process. This allows providing a better
comparison of the strengths and the weaknesses of
each technique in the segmentation of each type of
the mesh regions. For that reason, we thought to
evaluate the performance of a segmentation method
on each regions type of the image and not on the
entire image. Furthermore, this approach may help
in making the better choice of the segmentation
algorithm that is the most adapted to each 3D image
zone and this can be in applications such as:
watermarking, compression, medical imaging, etc.
7 CONCLUSIONS
This paper proposes a new approach of objective
quantitative evaluation of 3D mesh segmentation.
For this purpose, we have firstly selected a corpus of
various 3D models and their ground-truth. We have
adopted secondly a method for the classification of
segmented regions of each ground-truth object
according to the values of its principal curvatures.
Then, we have proposed three similarity measures
for the evaluation of the segmentation quality for
every region type (convex, concave or planar). To
validate our approach, we have selected eight recent
segmentation algorithms on heterogeneous images.
In terms of improving our results, there are a
number of interesting directions to explore.
Currently, we are working to fusion the compared
methods permitting to combine the results of the best
selected algorithms for each type of region. We also
plan to perform experiments with larger corpus in
terms of number of images to establish a complete
comprehensive study for an objective evaluation of
the 3D meshes segmentation.
REFERENCES
Amri S. and Zagrouba E. (2006). Evaluation and fusion of
image segmentation methods. In ICTTA :
International Conference on Information &
Communication Technologies: From Theory to
Applications, vol. 1, 1524-1529.
Attene M., Katz S., Mortara M., Patan G., Spagnuolo M.
and Tal A. (2006). Mesh segmentation, a comparative
study. In SMI : Proceedings of the IEEE International
Conference on Shape Modeling and Applications
2006, IEEE Computer Society, Washington, DC,
USA, 7.
Attene, M., Falcidieno, B., and Spagnuolo, M. (2006).
Hierarchical mesh segmentation based on fitting
primitives. Vis. Comput., vol. 22(3), 181-193.
Benhabiles H., Vandeborre J., Lavoué G. and Daoudi M.
(2009). A framework for the objective evaluation of
segmentation algorithms using a ground-truth of
human segmented 3d models. In SMI : Proceedings of
the IEEE International Conference on Shape
Modeling and Applications, 36-43.
Chen X., Golovinskiy A. and Funkhouser T. (2009). A
Benchmark for 3D Mesh Segmentation. ACM
Transactions on Graphics (Proc. SIGGRAPH), vol.
28(3), 1.
Golovinskiy A. and Funkhouser T. (2008). Randomized
cuts for 3D mesh analysis. ACM Transactions on
Graphics (Proc. SIGGRAPH ASIA), vol. 27(5), 145-
157.
Katz S., Leifman G. and Tal A. (2005). Mesh
segmentation using feature point and core extraction.
VISAPP 2011 - International Conference on Computer Vision Theory and Applications
208