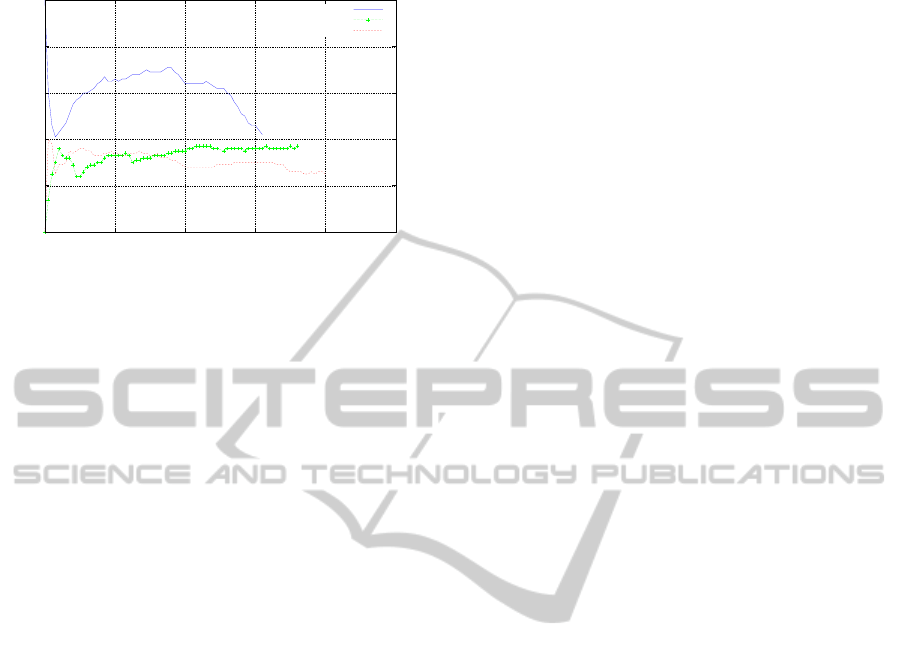
0
0.2
0.4
0.6
0.8
1
0 0.2 0.4 0.6 0.8 1
precision
recall
Precision vs. Recall VOC2005 Dataset 1
cars
people
bicycles
Figure 8: PR curve for the three classes of objects bicycles,
people e cars. The model used for this graph is MNOD-SI.
same type but different forms in different poses or
even articulated and occluded.
The proposed algorithm suffers from some issues
related to datasets with conspicuous differences of
scale for the objects that we want to detect. Therefore
we are working on a different strategy to overcome
this problem. Detection results are dependent on net-
work topology and from the selected set of features.
Then, we are analyzing new search algorithms able to
select the best network configuration.
REFERENCES
Bouchard, G. and Triggs, B. (2005). Hierarchical part-
based visual object categorization. In Proc. CVPR,
pages 710–715.
Crandall, D., Felzenszwalb, P., and Huttenlocher, D. (2005).
Spatial priors for part-based recognition using statisti-
cal models. In Proc. CVPR, pages 10–17.
Csurka, G., Dance, C. R., Fan, L., Willamowski, J., and
Bray, C. (2004). Visual categorization with bags of
keypoints. In In Workshop on Statistical Learning in
Computer Vision, ECCV, pages 1–22.
Dalal, N. and Triggs, B. (2005). Histograms of oriented
gradients for human detection. In Proc. CVPR, pages
886–893.
Everingham, M., Zisserman, A., Williams, C. K. I., Gool,
L. V., Allan, M., Bishop, C. M., Chapelle, O., Dalal,
N., Deselaers, T., Dork, G., Duffner, S., Eichhorn, J.,
Farquhar, J. D. R., Fritz, M., Garcia, C., Griffiths, T.,
Jurie, F., Keysers, D., Koskela, M., Laaksonen, J., Lar-
lus, D., Leibe, B., Meng, H., Ney, H., Schiele, B.,
Schmid, C., Seemann, E., Shawe-taylor, J., Storkey,
A., Szedmak, O., Triggs, B., Ulusoy, I., Viitaniemi,
V., and Zhang, J. (2005). The 2005 pascal visual ob-
ject classes challenge. In Selected Proceedings of the
First PASCAL Challenges Workshop.
Fei-fei, L. (2005). A bayesian hierarchical model for learn-
ing natural scene categories. In Proc. CVPR, pages
524–531.
Fergus, R., Perona, P., and Zisserman, A. (2003). Ob-
ject class recognition by unsupervised scale-invariant
learning. In Proc. CVPR, pages 264–271.
Fischler, M. and Elschlager, R. (1973). The representation
and matching of pictorial structures. Computers, IEEE
Transactions on, C-22(1):67 – 92.
Leibe, B. and Schiele, B. (2003). Interleaved object cate-
gorization and segmentation. In Proc. BMVC, pages
759–768.
Riedmiller, M. and Braun, H. (1993). A direct adap-
tive method for faster backpropagation learning: The
rprop algorithm. In IEEE INTERNATIONAL CON-
FERENCE ON NEURAL NETWORKS, pages 586–
591.
Riesenhuber, M. and Poggio, T. (1999). Hierarchical mod-
els of object recognition in cortex. Nature Neuro-
science, 2(11):1019–1025.
Schmid, C. (2006). Beyond bags of features: Spatial
pyramid matching for recognizing natural scene cat-
egories. In Proc. CVPR, pages 2169–2178.
Serre, T., Wolf, L., and Poggio, T. (2005). Object recog-
nition with features inspired by visual cortex. In
Proceedings of the Conference on Computer Vision
and Pattern Recognition, CVPR ’05, pages 994–1000,
Washington, DC, USA. IEEE Computer Society.
Sharkey, A. J. (1999). Combining Artificial Neural Nets:
Ensemble and Modular Multi-Net Systems, chapter
Multi-Net Systems. Springer.
Todorovic, S. and Ahuja, N. (2006). Extracting subimages
of an unknown category from a set of images. In Proc.
CVPR, pages 927–934.
Utgoff, P. E. (1988). Perceptron trees: A case study in hy-
brid concept representations. In AAAI, pages 601–606.
Zhu, L. and Yuille, A. (2006). A hierarchical compositional
system for rapid object detection. In Proc. NIPS.
VISAPP 2011 - International Conference on Computer Vision Theory and Applications
136