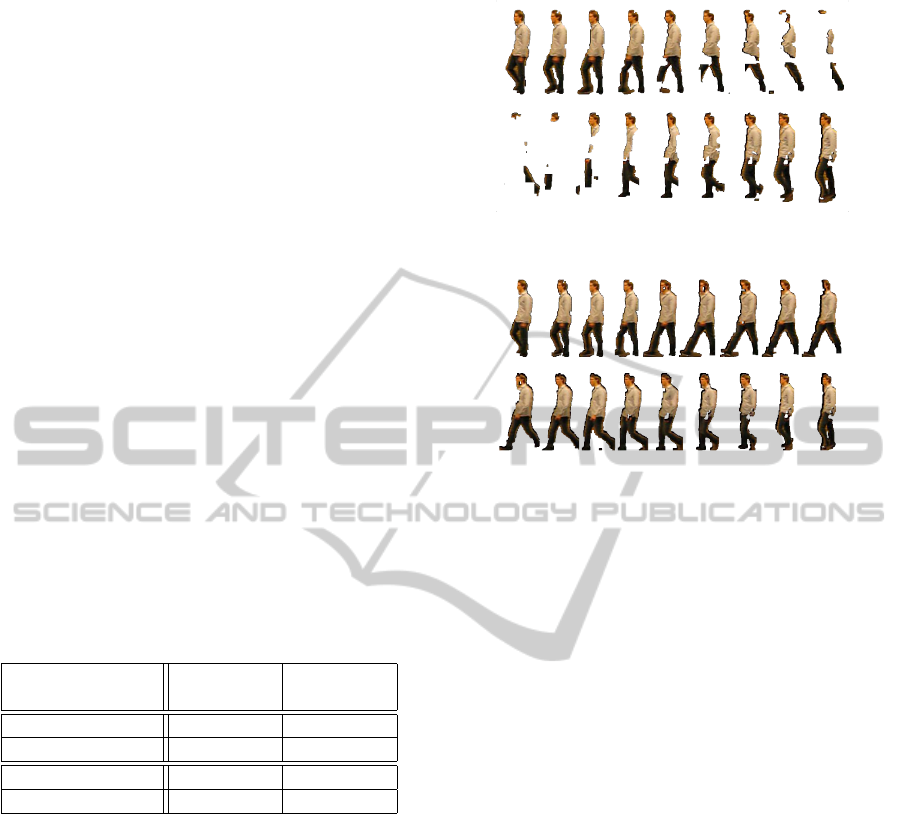
4 RESULTS
To our knowledge, not much work has focused on
gait recognition in the presence of occlusions. How-
ever it is a very important issue when moving to-
wards a real working gait recognition system. There
are many gait recognition databases, e.g. HumanID,
UCSD, CMU Mobo, Soton, CASIA, and others (Hof-
mann et al., 2011). We use the TUM-IITKGP gait
recognition database (Hofmann et al., 2011), because
it focuses on gait recognition with occlusions. This
database features 35 individuals which are recorded
in six different configurations (Regular, hands-in-
pocket, backpack, gown, dynamic occlusions, static
occlusions). In this paper we use the first configura-
tion for training and focus on the last two configura-
tions for recognition. For all our experiments we set
[α = 200, β = 80, γ = 30, δ = 40.000].
We compare our results to the two baseline algo-
rithms described in (Hofmann et al., 2011). In Table
1 evaluation results are shown. The first algorithm
(based on color histograms) is clearly outperformed.
The second baseline algorithm (based on Gait Energy
Image) could not work without the preprocessing we
propose in this paper.
Table 1: Top 1 recognition rates for Baseline 1 (Color His-
togram) and Baseline 2 (Gait Energy Image).
dynamic
occlusions
static
occlusions
Baseline 1 43.7% 70.0%
Baseline 1 + ours 84.3% 87.5%
Baseline 2 - -
Baseline 2 + ours 67.2% 72.7%
Qualitative results of our proposed reconstruction
method are shown in Figure 4. It can be seen that the
corrupted sequence is nicely reconstructed.
5 CONCLUSIONS
In this paper we have shown a preprocessing stage
that allows to reconstruct complete gait cycles such
that gait recognition is possible in spite of occlusions.
In principle this preprocessing can be applied to any
gait recognition algorithm. In our experiments we
have shown that a preprocessing like ours is in fact
beneficial in the case of occlusions.
(a) Sequence with occlusions
(b) Reconstructed sequence
Figure 4: Example of reconstruction (b) of gait cycle which
was originally (a) corrupted by occlusions.
REFERENCES
Boykov, Y., Veksler, O., and Zabih, R. (2001). Efficient ap-
proximate energy minimization via graph cuts. IEEE
TPAMI, Number 20(12):1222-1239.
Bugeau, A. and P´erez, P. Track and cut: Simultaneous
tracking and segmentation of multiple objects with
graph cuts.
Delong, A., Osokin, A., Isack, H. N., and Boykov, Y.
(2010). Fast approximate energy minimization with
label costs. CVPR.
Farneb¨ack, G. (2002). Polynomial Expansion for Orienta-
tion and Motion Estimation. PhD thesis, Link¨oping
University, Sweden.
Han, J. and Bhanu, B. (2006). Individual recognition using
gait energy image. IEEE TPAMI, Volume 28, Number
2.
Hofmann, M., Sural, S., and Rigoll, G. (2011). Gait recog-
nition in the presence of occlusion: A new dataset and
baseline algorithms. In International Conferences on
Computer Graphics, Visualization and Computer Vi-
sion (WSCG).
Kolmogorov, V. and Zabih, R. (2004). What energy func-
tions can be minimized via graph cuts? IEEE TPAMI,
Number 26(2):147-159.
Lee, L. and Grimson, W. E. L. (2001). Gait analysis for
recognition and classification. MIT Artificial Intelli-
gence Lab, Cambridge.
Wang, L., Tan, T., Ning, H., and Hu, W. (2003). Silhouette
analysis-based gait recognition for human identifica-
tion. IEEE TPAMI, Vol. 25, No. 10.
IDENTIFICATION AND RECONSTRUCTION OF COMPLETE GAIT CYCLES FOR PERSON IDENTIFICATION IN
CROWDED SCENES
597