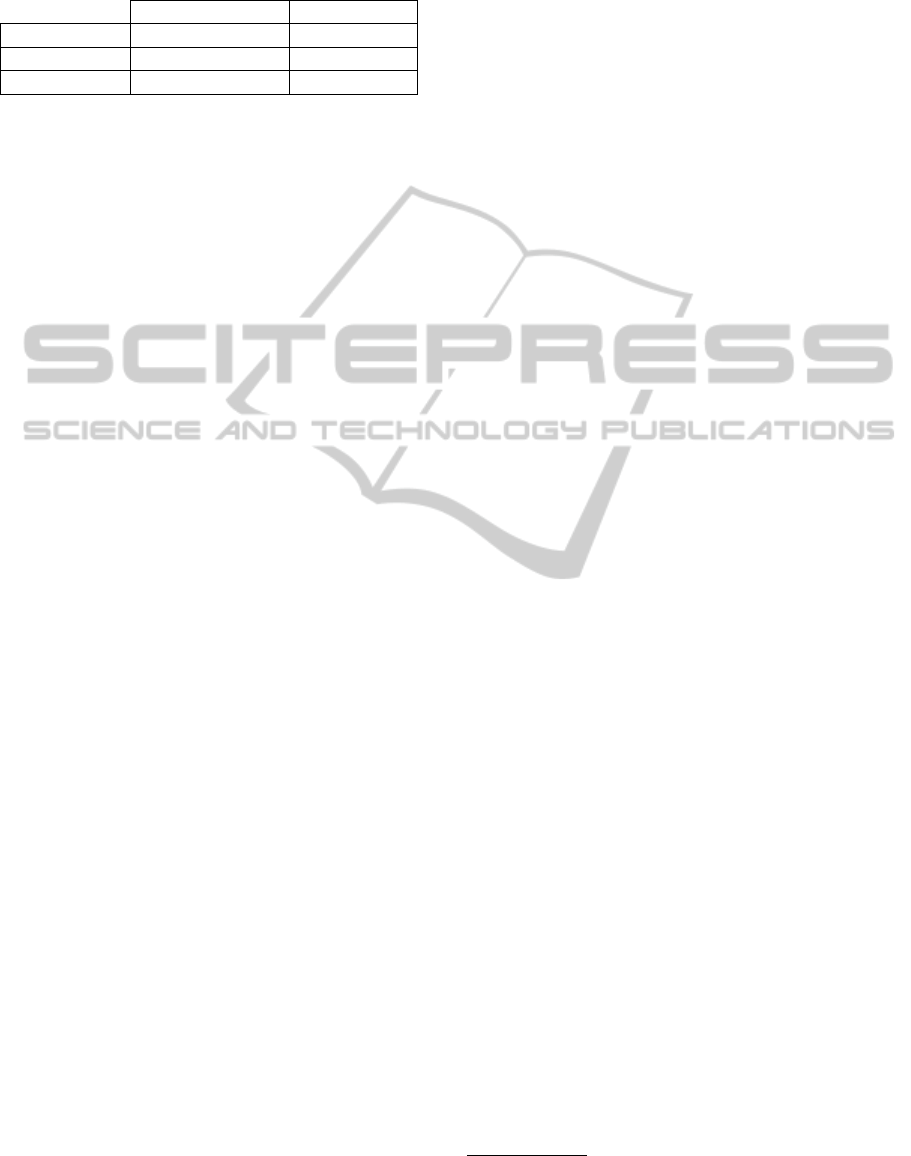
Table 2: A comparison of the pageviews, ratings and feed-
back rate of anonymous users and users who were logged-
in.
Anonymous Logged-in
Pageviews 1395289 (98.5%) 21221 (1.5%)
Ratings 7730 (95%) 371 (5%)
Feedback rate 0.55% 1.75%
events available on the website. Only 18% (=5446) of
them were rated at least once. Of the 5446 different
events that were rated, 23% (=1238) was rated more
than once, the remaining 77% (=4208) in the tail was
rated exactly once.
4 CONCLUSIONS
In this paper we described an online experiment
on explicit feedback mechanisms as used in recom-
mender systems. On a popular cultural events website
we randomly allowed browsing users to use one of
four most common feedback systems for a period of
183 days. Results showed that the static 5-star rating
mechanism collected the most feedback, closely fol-
lowed by the dynamic thumbs up/down system. This
is somewhat unexpected because it was the oldest sys-
tem and supposed to be the least attractive one. We
assume this has in fact favored this system as it was
easier recognizable as a feedback system.
The 5-star systems failed however to produce
more accurate feedback than the thumbs systems. De-
spite the fact that the items in our platform are events
rather than movie content, we have seen that users
interacted with the 5-star rating system in a similar
manner as they did on the youtube.com site which is
to rate either very high or very low values. Motiva-
tions for this behavior are unclear. It is however likely
that users tend to give more positive feedback (e.g.
higher rating values) because they only look at items
that seemed appealing in the first place. Counterintu-
itive was that users do not seem to prefer the dynamic
systems over the static ones.
The feedback rate of users who were logged-in
was more than 3 times higher than for anonymous
users. Logged-in users seemed to be more actively
involved and were more keen to provide explicit feed-
back. Still we think recommender systems should
carefully consider what to do with anonymous users,
as we saw that they generated 98.5% of all traffic in
our experiment.
We believe the collection of feedback data to be
a very important part of the recommendation pro-
cess that is often overlooked. The best recommender
may fail if it lacks sufficient input data. We have
shown that the design of the feedback system influ-
ences the rate at which users provide feedback and
should therefore be taken into consideration by online
recommender systems.
In future research we will continue to collect data
and extend the experiment with incentives for users
to start (and continue) rating, and thus creating better
data quality for recommender systems. We also plan
to de-anonymize users by means of cookie tracking
and integrate implicit feedback into this research.
ACKNOWLEDGEMENTS
We would like to thank CultuurNet Vlaanderen
2
for
the effort and support they were willing to provide for
deploying the experiment described in this paper.
REFERENCES
Amatriain, X., Pujol, J. M., Tintarev, N., and Oliver, N.
(2009). Rate it again: increasing recommendation ac-
curacy by user re-rating. In RecSys ’09: Proceedings
of the third ACM conference on Recommender sys-
tems, pages 173–180, New York, NY, USA. ACM.
Burke, R. (2002). Hybrid recommender systems: Survey
and experiments. User Modeling and User-Adapted
Interaction, 12:331–370. 10.1023/A:1021240730564.
Cosley, D., Lam, S., Albert, I., Konstan, J., and Riedl, J.
(2003). Is seeing believing?: how recommender sys-
tem interfaces affect users’ opinions. In Proceedings
of the SIGCHI conference on Human factors in com-
puting systems, pages 585–592. ACM.
Jawaheer, G., Szomszor, M., and Kostkova, P. (2010). Com-
parison of implicit and explicit feedback from an on-
line music recommendation service. In HetRec ’10:
Proceedings of the 1st International Workshop on In-
formation Heterogeneity and Fusion in Recommender
Systems, pages 47–51, New York, NY, USA. ACM.
Srinivas, K. K., Gutta, S., Schaffer, D., Martino, J., and
Zimmerman, J. (2001). A multi-agent tv recom-
mender. In proceedings of the UM 2001 workshop
”‘Personalization in Future TV”’.
Vintila, B., Palaghita, D., and Dascalu, M. (2010). A new
algorithm for self-adapting web interfaces. In 6th In-
ternational Conference on Web Information Systems
and Technologies, pages 57–62.
Yu, Z. and Zhou, X. (2004). Tv3p: an adaptive assistant for
personalized tv. Consumer Electronics, IEEE Trans-
actions on, 50(1):393–399.
2
http://www.cultuurnet.be
WEBIST 2011 - 7th International Conference on Web Information Systems and Technologies
394