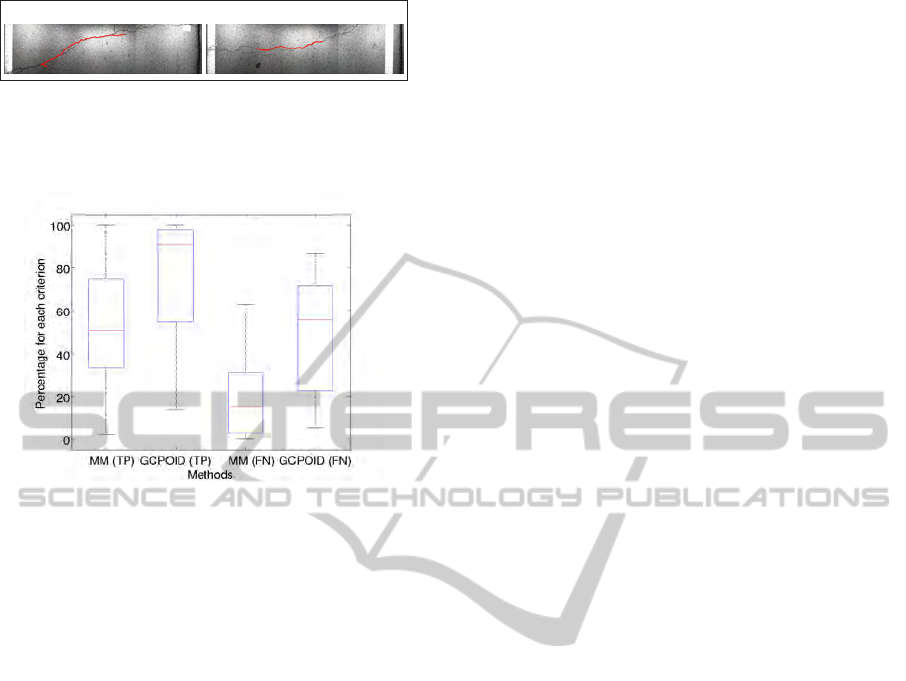
IMAGE F379
IMAGE F382
Figure 5: Detection of road cracks with GCPOID – The
POIs, obtained with AC (NCC), are linked in red with
geodesic contours. Detection is incomplete but with very
few false detections when there is no wrong POI.
Figure 6: Results obtained with GCPOID and MM – These
results are represented by boxes with one box for each cri-
teria (TP, true positives, and FN, false negatives) and each
method. The boxes have lines at the lower quartile, the
minimum, the median, the maximum and the upper quar-
tile. These results show that the median of the percentages
of correct detections is highly improved with GCPOID, be-
cause it becomes 90.54% against 50.68% for MM whereas
the percentage of false negatives for GCPOID is higher (the
median is 55.63%) than for MM (the median is 15.68%).
(2) On the contrary, MM obtains lower percentages
of false negatives (FN) than with GCPOID.
This is why the mean Dice similarity is better with
MM, 0.54, than with GCPOID, 0.46. However, the
result with GCPOID is reliable: the mean of the cor-
rect detections reached 74.43% (MM obtained only
50.89%). In consequence, we can consider that we
have reached our first goal: to obtain very few false
negatives. The second aim (complete detection) can
be obtained if we try to propagate the results of the
detection at the extremities of the crack detection, see
image F382 in Figure 5.
5 CONCLUSIONS
We introduced a new method of road crack detec-
tion based on two steps: automatic detection of
POIs followed by the estimation of geodesic con-
tours. The study reveals the most appropriate detec-
tor of POIs (by introducing the principle and the pa-
rameter settings): auto-correlation detector with nor-
malised cross correlation and fusion with the maxi-
mum. Moreover,we demonstrate the interest of using
geodesic contours for the final detection by compar-
ing to a Markovian segmentation.
Our future work will focus on how adding more
POIs (to have a complete/dense detection), with
the combination of prefiltered images with auto-
correlation detection, and how removing wrong POIs,
by adding some constraints (based on the variation
of grey levels in the neighborhood or on the shape
of the curves determined by each pair of POIs). For
the geodesic contours, we will propose a more real-
istic algorithm for connecting the POIs (possibility to
connect one point with numerous others, to remove a
point if the energy is low with all the paths passing
through this point).
REFERENCES
Chambon, S. and Crouzil, A. (2003). Dense matching using
correlation: new measures that are robust near occlu-
sions. In BMVC, volume 1, pages 143–152.
Chambon, S., Gourraud, C., Moliard, J., and Nicolle, P.
(2010). Road crack extraction with adapted filtering
and markov model-based segmentation – introduction
and validation. In VISAPP.
Cohen, L. and Kimmel, R. (1997). Global minimum for ac-
tive contour models: A minimal path approach. IJCV,
24(1):57–78.
Delagnes, P. and Barba, D. (1995). A markov random field
for rectilinearstructure extraction inpavement distress
image analysis. In ICIP, volume 1, pages 446–449.
Harris, C. and Stephens, M. (1988). A combined corner and
edge detector. In Alvey Vision Conf., pages 147–151.
Kass, M., Witkin, A., and Terzopoulos, D. (1988). Snakes:
Active contour models. IJCV, 1(4):321–331.
Koutsopoulos, H. and Downey, A. (1993). Primitive-based
classification of pavement cracking images. Journal
of Transportation Engineering, 119(3):402–418.
Lowe, D. (2004). Distinctive image features from scale-
invariant keypoints. IJCV, 60(2):91–110.
Moravec, H. (1977). Toward automatic visual obstacle
avoidance. In IJCAI, volume 2, page 584.
Oliveira, H. and Correia, P. (2008). Supervised strategies
for cracks detection in images of road pavement flexi-
ble surfaces. In EUSIPCO.
Oliveira, H. and Correia, P. (2009). Automatic road
crack segmentation using entropy and image dynamic
thresholding. In EUSIPCO.
Tanaka, N. and Uematsu, K. (1998). A crack detection
method in road surface images using morphology. In
Machine Vision Applications, pages 154–157.
DETECTION OF POINTS OF INTEREST FOR GEODESIC CONTOURS - Application on Road Images for Crack
Detection
213