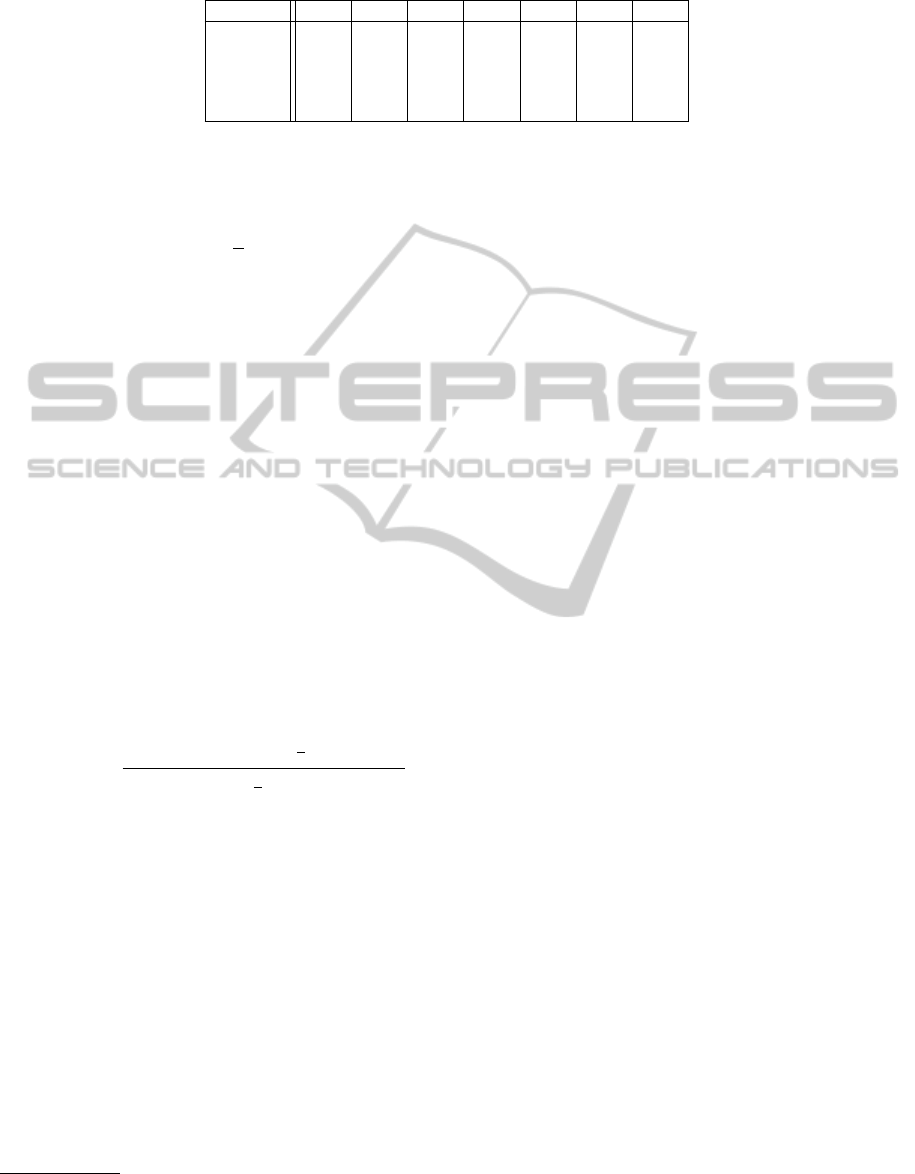
Table 1: Accuracy obtained by cross-validation for different parameter values λ.
subject \ λ 10
−6
10
−4
10
−2
1 10
2
10
4
10
6
aa 0.607 0.597 0.624 0.616 0.633 0.614 0.605
al 0.979 0.979 0.885 0.676 0.821 0.979 0.979
av 0.645 0.635 0.513 0.538 0.620 0.645 0.657
aw 0.681 0.710 0.643 0.618 0.647 0.666 0.683
ay 0.939 0.939 0.857 0.685 0.756 0.823 0.802
with
w
T
=
w
T
0
v
T
1
. . . v
T
S
,
¯
Σ
(2)
s
(λ) =
¯
Σ
(2)
s
+
1
λ
D
0
+ λD
s
,
¯
Σ
(i)
s
= E
s
Σ
(i)
s
E
T
s
and
E
s
=
I
d×d
0
(s−1)d×d
I
d×d
0
(S−s)d×d
,
D
0
=
I
d×d
0
Sd×d
I
d×d
0
d×Sd
,
D
s
=
0
sd×d
I
d×d
0
(S−s)d×d
0
d×sd
I
d×d
0
d×(S−s)d
.
We find the maximum through gradient search. To
avoid finding the optimal step length in each iteration
and speeding up convergence we employ the RProp+
algorithm, proposed in (Riedmiller and Braun, 1993)
for supervised learning in feedforward artificial neu-
ral networks. The gradient can be computed as
∇R(w) =
S
∑
s=1
¯
Σ
(1)
s
w − r
s
(w)(
¯
Σ
(2)
s
+
1
λ
D
0
+ λD
s
)w
w
T
(
¯
Σ
(2)
s
+
1
λ
D
0
+ λD
s
)w
.
The RProp+ method is summarized in Algorithm 1
and uses the weight-backtracking approach. An intu-
itive way to intialize the component vector w
0
in w
is to take the average of the covariance matrices of
all subjects and compute the best filter with the ba-
sic CSP algorithm. Initializing the other component
vectors v
s
in w is even easier, just run the basic CSP
algorithm on the covariance matrices of each subject
separately and select the best filter as starting point.
3 EXPERIMENTS
We use data of the third BCI competition
2
, more pre-
cisely data set IVa. The set contains data recorded
2
On http://www.bbci.de/competition/iii/ you can find
the data sets and results of the 3e BCI competition.
from 118 electrodes where the subjects performed
two tasks: right hand motor imagery and foot im-
agery. Five subjects are included in the set and each
subject recorded 280 trials. From each of these sub-
jects, we use 100 trials for training and 180 for test-
ing. To limit the number of parameters that needs to
be computed by the RProp+ algorithm, the number of
channels is reduced to 22. The ones selected are Fp1,
Fpz, Fp2, F7, F3, Fz, F4, F8, T7, C3, Cz, C4, T8, P7,
P3, Pz, P4, P8, POz, O1, Oz and O2. All remaining
signals are band-pass filtered between 8 and 30 Hz.
The trade-off parameter λ is determined through
cross-validation, which is the reason we still need
a sufficient amount of data to accurately select the
paramter value. For each subject only two spatial fil-
ters are computed: one for each class. The reason
for the limit of one filter per class is the bad con-
vergence of the algorithm after one iteration of pro-
jection deflation (a technique also use in principal
component analysis to compute subsequent princi-
pal components). Table 1 shows the cross-validation
accuracy for each subject and different parameters
λ ∈ {10
−6
, 10
−4
, 10
−2
, 1, 10
2
, 10
4
, 10
6
}. Clearly, for
some subjects a global filter is preferred (subject av),
while for others a more intermediate filter is chosen
(subject aa) or even a subject specific filter (subjects
aw and ay). For subject al it does not matter which
model parameter to choose as both global and specific
filters perform equally well.
Figure 2 shows the spatial filters for two subjects
av and ay, computed both with the basic CSP variant
and with the multitask variant. As subject ay prefers a
subject specific model, one can see that the multitask
CSP variant (msCSP) converges to the same filter as
the basic CSP variant (bCSP) for very low values of
λ. However, for subject av the difference between the
two filter variants can not be unnoticed. The global
filters in the second and fourth column show a more
physiological plausible solution, which is also sup-
ported by a higher accuracy on the test set as one can
see in Table 3. In general, the multitask variant seems
to improve the overall accuracy for each subject, ex-
cept for subject aa, in which case a small decrease in
performance is observed. The improvement in sub-
jects such as av and aw, that initially do not perform
well, can be due to the influence of subjects who do
BIOSIGNALS 2011 - International Conference on Bio-inspired Systems and Signal Processing
380