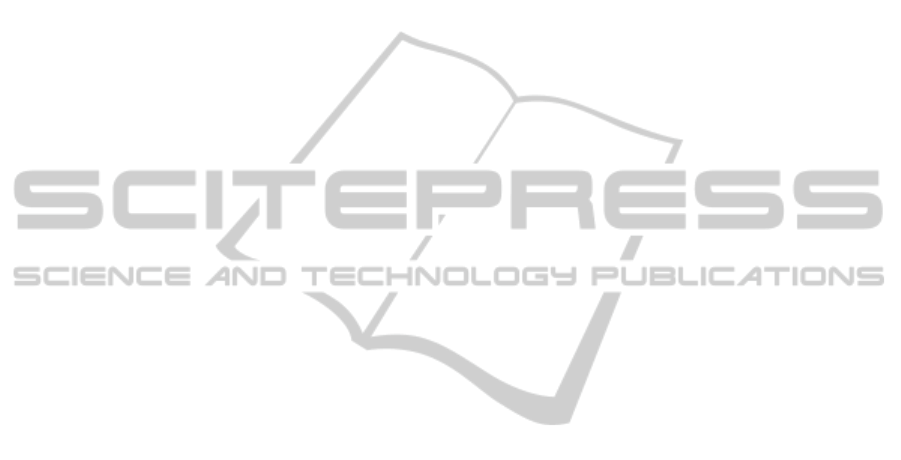
because it represents 105 interventions (out of 175,
i.e. about 60%).
The case of partial correspondence represents 64
interventions (out of 175, i.e. 36.6). In 4 of these,
teachers selected too many indicators while
intervening. We noticed while listening to the audio
conversations that these interventions lasted more
than 5 minutes. In analyzing the contents, we also
learned that in these cases teachers dealt with one
problem and interacted with the learner about it but
the latter had difficulty resolving the problem.
Teachers took time to explain, step by step, how to
come to a resolution of the problem. Thus, we can
suppose that in these situations they did not want to
overload the learner in giving him a lot of
information. They tried consequently to help
learners gradually by first resolving the problem
with which they had difficulties, and then took the
remaining ones into account.
In the case of partial correspondence, 60
interventions concern situations where teachers do
more than they had declared. They have done the job
in so far as they have actually interacted with the
learner about the selected problems. Moreover,
doing more than was originally declared poses
problems because the identification of teaching
intention is not complete. Thus, since teaching
intention is at the core of teachers’ self-regulation,
its incomplete identification can bring up some
issues at the time of the instrumentation of the
self-regulation process.
7 CONCLUSION AND OUTLOOK
In our work, we want to instrument teachers’
activities during learning session. Here, we focus on
identifying teachers’ intentions through a declarative
approach by asking teachers what makes them
intervene. For that, we offer them a tool in which
they can select a set of indicators, which are
supposed to be the triggers of their interventions.
Experimental results show that most of the time (in
82% of interventions) when they intervened teachers
declared their intentions through indicators
selection. However, partial correspondence between
the interventions contents and the problems
underlined by indicators teachers selected while
intervening arise new issues. Indeed, incomplete
identification of teaching intentions could lead to
failure or errors during teachers’ self-regulation
support since this latter is based on teaching
intentions. Addressing these issues will be our
short-term objectives by giving teachers the
opportunity to adjust their intentions after the
interventions (add or deletion of some indicators
from the list of indicators selected pre-intervention).
Our mid-term objectives will be the implementation
of teachers’ self-regulation process and its
evaluation by carrying out a new experimentation.
We also plan for our long-term objectives to propose
learners some of the indicators available for teachers
in order to support self-regulated learning (Butler et
al., 1995).
REFERENCES
Bandura, A. (1977). Social Learning Theory. NJ Prentice
Hall Publishers, Englewood Cliffs.
Benbenutty, H. (2007). Teachers’self-efficacy and
self-regulation. http://www.thefreelibrary.com/.
Butler, D. L., Winne, P. H. (1995). Feedback and self-
regulated learning: A theoretical synthesis. Review of
Educational Research, 65:245-281.
Capa-Aydin, Y., Sungur, S., and Uzuntiryaki, E. (2009).
Teacher self-regulation: Examining a
multidimensional construct. Educational Psychology,
29:345–356.
Després, C. (2003). Synchronous tutoring in distance
learning. In International Conference on Artificial
Intelligence, pages 271–278, Australia.
ICALTS-JEIRP (2004). Interaction and collaboration
analysis’ supporting teachers and students’
self-regulation, delibrables 1, 2 and 3.
http://www.rhodes.aegean.gr/ltee/kaleidoscope-icalts.
Kazanidis, I., Satratzemi, M. (2010). Modeling user
progress and visualizing feedback: the case of ProPer.
In 2
nd
International Conference on Computer
Supported Education. pages 46-53, Spain.
Labat, J.-M. (2002). Quel retour pour le tuteur ? In
Technologies de l’Information et de la Communication
dans les Enseignements et dans l’industrie, France.
Lefevre, M., Cordier, A., Jean-Daubias, A., and Guin,
S.(2009). A teacher-dedicated tool supporting
personalization of activities. In World Conference on
Educational Multimedia, Hypermedia and
Telecommunications, pages 1136–1141, Hawaii.
Lekira, A., Després, C., and Jacoboni, P. (2009).
Supporting teacher’s self-regulation process. In 9th
IEEE International Conference on Advanced Learning
Technologies, pages 589–590, Latvia.
Martinez, A., Dimitriadis, Y., Gomez, E., Rubia, B., and
De La Fuente, P. (2003). Combining qualitative and
social network analysis for the study of classroom
social interactions. Computers and Education,
41:353-368.
Zimmerman, B. J. (2000). Attaining self-regulation: A
social cognitive perspective. Handbook of
self-regulation, pages 13–38.
CSEDU 2011 - 3rd International Conference on Computer Supported Education
116