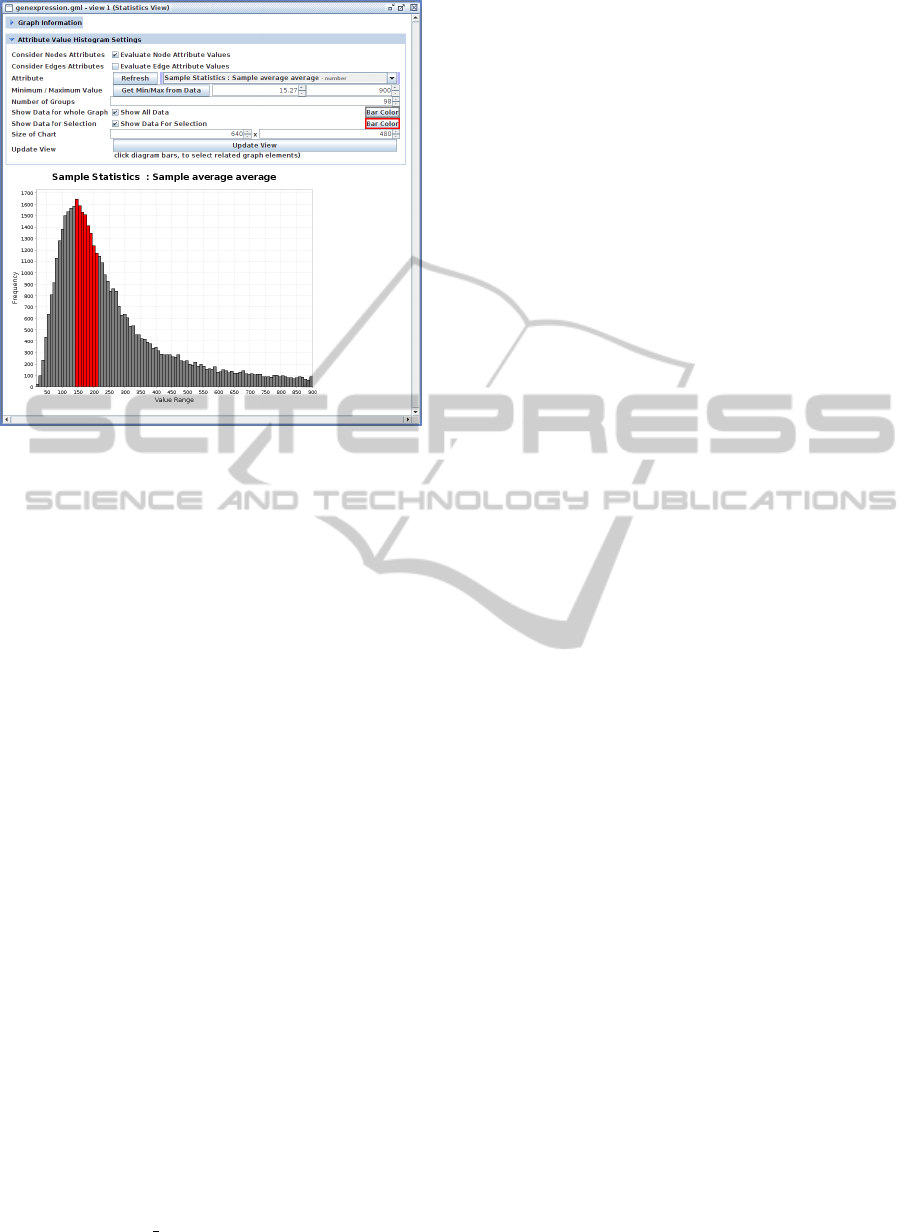
Figure 6: Screenshot of the Statistics View visualizing hu-
man gene expression rate values mapped onto a network as
a histogram. The user is able to select parts of the data (red
bars) and this selection will also be applied to the underly-
ing network.
pairwise measurement values. These displayed data
points may have different colors, indicating measure-
ments of different conditions.
2.4.3 Statistics View
The last view is depicted in Figure 6. This view shows
the distribution of graph-element attribute values as a
histogram. This view can be used to visually inspect
graph properties or experimental data mapped onto
networks. An example is the investigation of compre-
hensive gene expression data sets in order to perform
a quality check by recognizing the distribution of the
data, or by selecting and removing outlier values.
3 CONCLUSIONS
We described a number of views, which enables do-
main scientists to visualize and analyze integrated and
flexibly combined biological data of different types.
Interaction techniques were developed to support do-
main scientists to visually explore their data. Many
of the described techniques and views are already im-
plemented in the HIVE add-on for VANTED. The next
version of the add-on will provide users all described
features. A video showing the described views and in-
teraction techniques is available at http://vanted.ipk-
gatersleben.de/hive ivapp11.
The set of visualization and interaction tools are
at the moment used in cooperation with domain ex-
perts, in order to create different integrated views
on datasets consisting of large-scale gene-expression
data, metabolic time-series data, microscopy im-
ages, photographs, volumes derived from NMR Spec-
troscopy and KEGG metabolic pathways. We were
not yet able to exploit the full capabilities of the pre-
sented approaches, as it is hard to find comprehensive
experimental datasets of the same origin, biological
material and methods, which would ideally cover all
of the supported data domains at the same time. We
are giving the tools into the hands of researchers in or-
der to overcome this limitation. Based on their com-
ments and experiences in using the system we will
iteratively improve and extend the system as well as
the underlying methods, promoting the realization of
complex biological use cases.
ACKNOWLEDGEMENTS
This work was partly supported by grant BMBF
0315044A
REFERENCES
Abramoff, M., Magelhaes, P., and Ram, S. (2004). Image
processing with ImageJ. Biophotonics International,
11:36–42.
Ashburner, M., Ball, C., Blake, J., Botstein, D., Butler, H.,
Cherry, J., Davis, A., Dolinski, K., Dwight, S., Ep-
pig, J., et al. (2000). Gene ontology: tool for the uni-
fication of biology. The Gene Ontology Consortium.
Nature Genetics, 25(1):25–29.
Davidson, D., Bard, J., Brune, R., Burgerc, A., Dubreuil,
C., Hill, W., Kaufman, M., Quinn, J., Stark, M., and
Baldock, R. (1997). The mouse atlas and graphical
gene-expression database. Seminars in Cell & Devel-
opmental Biology, 8(5):509–517.
Eick, S. G. and Wills, G. J. (1995). High interaction
graphics. European Journal of Operations Research,
81(3):445–459.
Gansner, E. R. and Koren, Y. (2007). Improved circular lay-
outs. Lecture Notes in Computer Science, 4372:386–
398.
Holten, D. and Wijk, J. J. V. (2009). Force-directed edge
bundling for graph visualization. Computer Graphics
Forum, 28(3):983–990.
Kanehisa, M. and Goto, S. (2000). KEGG: Kyoto encyclo-
pedia of genes and genomes. Nucleic Acids Research,
28(1):27–30.
Kanehisa, M., Goto, S., Hattori, M., Aoki-Kinoshita, K. F.,
Itoh, M., Kawashima, S., Katayama, T., Araki, M.,
IVAPP 2011 - International Conference on Information Visualization Theory and Applications
260