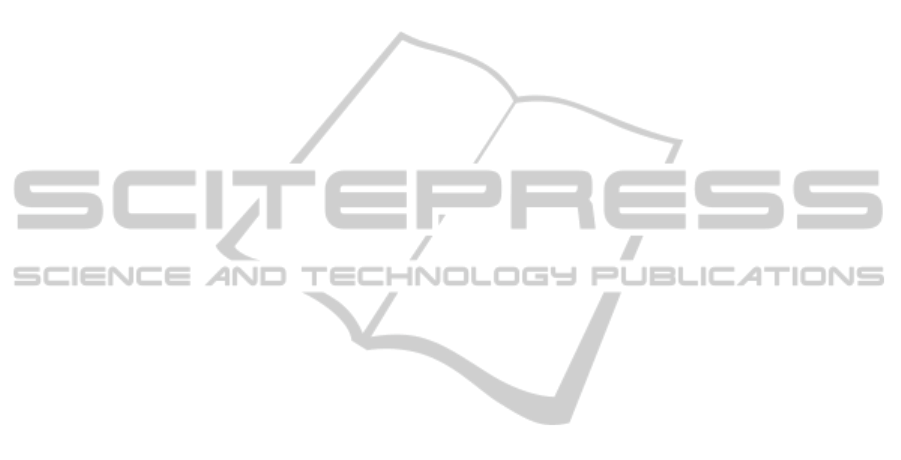
Heer, 2009).
We have processed the data following the seven
steps stressed by (Ben Fry, 2008) in his work on
visualization. This path consists of seven stages:
1.Acquire - 2.Parse - 3.Filter - 4.Mine - 5.Represent
- 6. Refine - 7.Interact:
1 - the acquisition phase was carried out
manually writing out the data from the sources cited
above, in some spreadsheets;
2 - the parsing was made using open source
spreadsheet application (Calc, included in
Openoffice Suite);
3 - the filtering stage involved the exclusion of
the negligible samples (in order to this analysis) and
some adjustment on the wrongly reported values;
4 - mining is consisted in reporting data gotten in
the earlier stages in a JavaScript file
5 - in the representation step two different sketch
were generated with Protovis using as data source
the JavaScript file created in 4th step;
6 - the refinement was made by acting directly
on the Protovis html and JavaScript related files by
an open source code editor (notepad++);
7 - the interaction phase was in part already
developed during the 5st stage and, afterwards
completed by uploading the project on the server.
3 CONCLUSIONS
This work is the first step of an ongoing project.
Further developments include the application of this
kind of analysis and visualization to the actual
contents (audio and video) of TV-series and in the
correlation of these semantic data to quantitative
data types and categories.
This analysis reveals some interesting insights.
First we see that the TV series aired on the same
network tend to have a similar number of spectators.
Notwithstanding the difference in the dataset and the
type of analysis, the two visualizations must be
viewed in relation: if visualization A shows how the
universe of the TV series is divided and segmented
according to market and consumption logic, typical
of large television networks, visualization B,
contrariwise, shows a dense set of correlations that
suggest a deeply interconnected world, so the Tv
series universe emerges as united and unified,
regardless of the commercial logic of the single
network. For example, visualization B shows a
dense network of cooperation between the products
aired by different networks.
Another insight revealed by the analysis of
audience datas (visualization A) concerns the
proportion between the number of viewers and
longevity of the series. On large networks free-to-air
longevity is directly linked to the number of
spectators and to its stability over time, instead pay-
TV networks keep on air for a longer time even
niche products (e.g. Dexter, Weeds, Californication).
This new prospective model of analysis could be
the basis for a new cultural analysis (Manovich,
2007) of the television system as an agency of
cultural dissemination.
REFERENCES
Abbruzzese, A. (2001). Forme estetiche e società di
massa: arte e pubblico nell'età del capitalismo.
Venezia: Marsilio.
Bostock, M., Heer, J. (2009). Protovis: A
GraphicalToolkit for Visualization. TVCG’09 15, 6,
1121-1128.
Brancato, S. (2007). Senza fine: immaginario e scrittura
della fiction seriale in Italia. Napoli: Liguori.
Fry, B. (2008). Visualizing data. O'Reilly Media, Inc.
Harrison, C. (2008) Visualizing the Bible. http://www.c
hrisharrison.net/projects/bibleviz.
Keim, D. A., Oelke, D. (2007). Literature fingerprinting:
A new method for visual literary analysis. In IEEE
Symposium on Visual Analytics and Technology
(VAST 2007), pages 115–122.
Latour, B. (2005) Reassembling the Social: An
Introduction to Actor-network-theory. Oxford, Oxford
University Press.
Lotman, Y. (1990). Universe of the Mind: A Semiotic
Theory of Culture. Bloomington, IN: Indiana
University Press.
Manovich, L. (2007). Cultural Analytics: Analysis and
Visualizations of Large Cultural Data Sets,
Unpublished manuscript. Retrieved October 15, 2010
from http://lab.softwarestudies.com
Manovich, L. (2009). How to Follow Global Digital
Cultures, or Cultural Analytics for Beginners. In K.
Becker & F. Stalder (Eds), Deep Search: The Politics
of Search beyond Google, London: Transaction
Publishers.
Manovich, L. (2010). Software culture. Milano: Olivares.
Moretti, F. (2005). Graphs, Maps, Trees. Absract Models
for a Literary History. London and New York: Verso.
Paley, W. B. (2002) TextArc: Showing Word Frequency
and Distribution in Text. Poster presented at IEEE
Symposium on Information Visualization 2002.
Venturini, T. (2008) Introducing the cartography of
controversies in Etnografia e ricerca qualitativa, vol.
3, 2008.
Wattenberg, M. (2002) Arc Diagrams: Visualizing
Structure in Strings, Proceedings of the IEEE
Symposium on Information Visualization, Boston,
MA, October 28-29, 2002 pp. 110-116.
TOWARD A POST-SERIALITY MAP OF TV SERIES - Visualizing the New TV Seriality System with Protovis
265