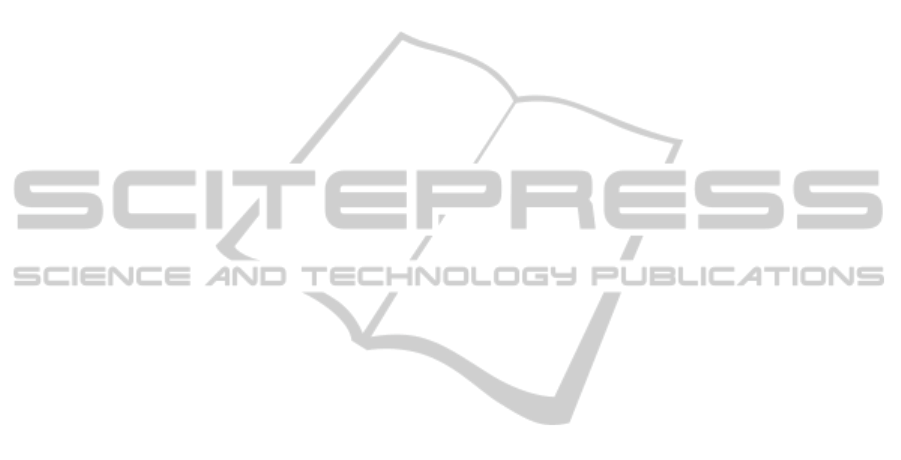
For instance, in the perceptual meaning of salience,
the context is the part of the rest of the image that
has features different to those of the salient image
region. But in other meanings of salience, such as
the probability-based, the context does not necessar-
ily play such a role: bikes can be found next to each
other even if they do not visually protrude from the
background.
The when question is relevant when the temporal
variable is included in the problem domain. This is
the case, for instance, of video sequences. However, if
a sequence is considered a 3D volume, then the when
would become the where. Therefore, depending on
its particular statement, a given problem may require
different questions or different answers to these ques-
tions.
The why question has to do with the interpreta-
tion of the results. As far as we know, this issue
has not been addressed up to now or, at least, has
not received significant research attention. While hu-
man observers might be able to report why something
has been detected as salient, it would be desirable to
work towards the automation of the explanation pro-
cess. Studies on how the task being undertaken might
influence the attention (Navalpakkam and Itti, 2005)
are partially related to this issue. However, generally
speaking, the why question is an open research issue.
The how question provides the opportunity to dis-
tinguish salience-based solutions depending on the
models, approaches, methodologies or even tech-
nologies being used. For instance, a biologically-
motivated visual attention model might be used in one
case, and a mathematically-based sound technique in
another.
Besides being useful as a taxonomy tool, this
framework can be helpful in finding new problems,
in stating existing problems in new ways, etc., even
for problems outside the visual domain. For exam-
ple, feature selection is a traditional problem in Pat-
tern Recognition, but it can also be seen as a salience
problem, since discriminative features form patterns
within a same class which are different from those be-
longing to other classes. Thus, answers to the where
question could be, in these cases, the feature space for
feature selection, or the set of classes in classification.
4 CONCLUSIONS
The main goal of this position paper was to make
readers aware that the term salience is used with
different meanings in the computer vision literature.
This lack of agreement in the terminology may affect
practitioners, specially new ones, who may find dif-
ficult or confusing some usages of the salience con-
cept or, even worse, be confused without being aware
they are. To explore the implications of this situation,
possible meanings of salience have been grouped into
four categories, and illustrated with a few representa-
tive examples drawn from the literature.
However, besides the differences across these sev-
eral usages, some commonalities may also be identi-
fied. Because both, differences and similarities, can
be useful to find new problems, or new approaches to
solve old problems, a simple formal framework has
been suggested as a tool to (i) help use a consistent
vocabulary; (ii) avoid ambiguities in meanings; and
(iii) get inspiration to reuse concepts and ideas, even
in the case of problems not related to visual attention
but that could be conceptualized similarly.
It is our hope that this paper has helped to clarify
ideas on salience, motivated authors to use the term
unambiguously, and suggested scientists new research
avenues by exploring and exploiting similarities and
differences across the several usages of salience.
ACKNOWLEDGEMENTS
The authors acknowledge the funding from the Span-
ish research programme Consolider Ingenio-2010
CSD2007-00018, and from Fundaci
´
o Caixa-Castell
´
o
Bancaixa under project P1·1A2010-11.
REFERENCES
Boiman, O. and Irani, M. (2007). Detecting irregularities
in images and in video. Intl. J. of Computer Vision,
74(1):17–31.
Han, J. W. and Guo, L. (2003). A shape-based image re-
trieval method using salient edges. Signal Processing:
Image Communication, 18(2):141–156.
iLab (2000). iLab, University of Sourthern California.
http://ilab.usc.edu.
Itti, L. and Koch, C. (2000). A saliency-based search mech-
anism for overt and covert shifts of visual attention.
Vision Research, 40(10–12):1489–1506.
Itti, L., Koch, C., and Niebur, E. (1998). A model of
saliency-based visual attention for rapid scene anal-
ysis. IEEE T-PAMI, 20(11):1254–1259.
Kadir, T. and Brady, M. (2001). Saliency, scale and image
description. Intl. J. of Computer Vision, 45(2):83–105.
Ko, B. C. and Nam, J.-Y. (2006). Automatic object-of-
interest segmentation from natural images. In ICPR
’06: Proceedings of the 18th International Confer-
ence on Pattern Recognition, pages 45–48, Washing-
ton, DC, USA. IEEE Computer Society.
VISAPP 2011 - International Conference on Computer Vision Theory and Applications
344