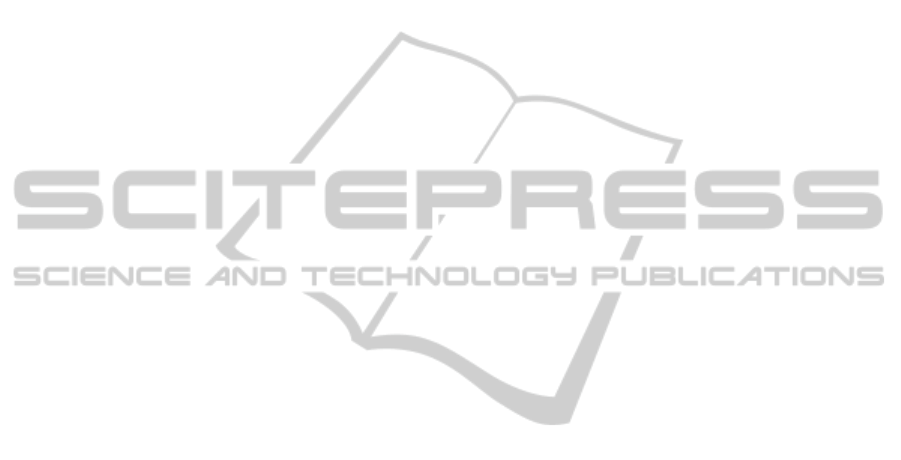
REFERENCES
Brown, L. M., Senior, A. W., Tian, Y., Connell, J., Ham-
papur, A., Shu, C., Merkl, H., and Lu, M. (2005).
Performance evaluation of surveillance systems under
varying conditions. In IEEE International Workshop
on Performance Evaluation of Tracking and Surveil-
lance (PETS). http://www.research.ibm.com/people
vision/performanceevaluation.html.
Chen, Y.-T., Chen, C.-S., Huang, C.-R., and Hung, Y.-P.
(2007). Efficient hierarchical method for background
subtraction. Pattern Recognition, 40(10):2706–2715.
Cheung, S. S. and Kamath, C. (2004). Robust techniques
for background subtraction in urban traffic video. In
Proceedings of SPIE, volume 5308, pages 881–892.
De Bruyne, S., Poppe, C., Verstockt, S., Lambert, P., and
Van de Walle, R. (2009). Estimating motion reliability
to improve moving object detection in the h.264/avc
domain. In IEEE International Conference on Multi-
media and Expo (ICME), pages 330–333.
Dhome, Y., Tronson, N., Vacavant, A., Chateau, T., Gabard,
C., Goyat, Y., and Gruyer, D. (2010). A benchmark
for background subtraction algorithms in monocular
vision: a comparative study. In IEEE International
Conference on Image Processing Tools, Theory and
Applications (IPTA). To appear.
Goyat, Y., Chateau, T., Malaterre, L., and Trassoudaine, L.
(2006). Vehicle trajectories evaluation by static video
sensors. In IEEE Intelligent Transportation Systems
Conference (ITSC), pages 864–869.
Gruyer, D., Royere, C., du Lac, N., Michel, G., and Blos-
seville, J.-M. (2006). SiVIC and RTMaps, intercon-
nected platforms for the conception and the evaluation
of driving assistance systems. In World Congress and
Exhibition on Intelligent Transport Systems and Ser-
vices (ITSC), pages 1–8.
Hayman, E. and Eklundh, J.-O. (2003). Statistical back-
ground subtraction for amobile observer. In IEEE In-
ternational Conference on Computer Vision (CVPR).
Kaewtrakulpong, P. and Bowden, R. (2001). An im-
proved adaptive background mixture model for real-
time tracking with shadow detection. In European
Workshop on Advanced Video Based Surveillance Sys-
tems (AVSS).
Kim, K., Chalidabhongse, T., Harwood, D., and Davis, L.
(2005). Real-time foreground-background segmen-
tation using codebook model. Real-time Imaging,
11(3):167–256.
Liu, Z., Lu, Y., and Zhang, Z. (2007). Real-time spatiotem-
poral segmentation of video objects in the H.264 com-
pressed domain. Journal of Visual Communication
and Image Representation, 18(3):275–290.
Mehmood, K., Mrak, M., Calic, J., and Kondoz, A. (2009).
Object tracking in surveillance videos using com-
pressed domain features from scalable bit-streams.
Image Communication, 24(10):814–824.
Poppe, C., De Bruyne, S., Paridaens, T., Lambert, P., and
Van de Walle, R. (2009). Moving object detection in
the H.264/AVC compressed domain for video surveil-
lance applications. Journal of Visual Communication
and Image Representation, 20(6):428–437.
Poppe, C., Martens, G., Lambert, P., and Van de Walle,
R. (2007). Improved background mixture models for
video surveillance applications. In Yagi, Y., Kang, S.,
Kweon, I., and Zha, H., editors, ACCV 2007, volume
4843 of LNCS, pages 251–260. Springer.
Sigari, M. H. and Fathy, M. (2008). Real-time back-
ground modeling/subtraction using two-layer code-
book model. In International MultiConference of En-
gineers and Computer Scientists.
Solana-Cipres, C., Fernandez-Escribano, G., Rodriguez-
Benitez, L., Moreno-Garcia, J., and Jimenez-Linares,
L. (2009). Real-time moving object segmentation in
H.264 compressed domain based on approximate rea-
soning. International Journal of Approximate Reason-
ing, 51(1):99–114.
Stauffer, C. and Grimson, W. E. L. (1999). Adaptative back-
ground mixture models for a real-time tracking. In
IEEE International Conference on Computer Vision
and Pattern Recognition (CVPR), volume 2, pages
246–252.
Tuzel, O., Porikli, F., and Meer, P. (2005). A bayesian ap-
proach to background modeling. In Conference on
Computer Vision and Pattern Recognition (CVPR).
Wiegand, T., Sullivan, G., Bjontegaard, G., and Luthra, G.
(2003). Overview of the H.264/AVC video coding
standard. IEEE Transactions on Circuits and Systems
for Video Technology, 13(7):560–576.
XiaHou, X.-J. and Gong, S.-R. (2008). Adaptive shad-
ows detection algorithm based on Gaussian mixture
model. In International Symposium on Information
Science and Engineering.
You, W., Houari Sabirin, M. S., and Munchurl, K. (2007).
Moving object tracking in H.264/AVC bitstream. In
Multimedia Content Analysis and Mining (MCAM),
volume 4577 of LNCS, pages 483–492. Springer.
Zivkovic, Z. (2004). Improved adaptive gaussian mixture
model for background subtraction. In IEEE Interna-
tional Conference on Pattern Recognition (ICPR).
VISAPP 2011 - International Conference on Computer Vision Theory and Applications
58