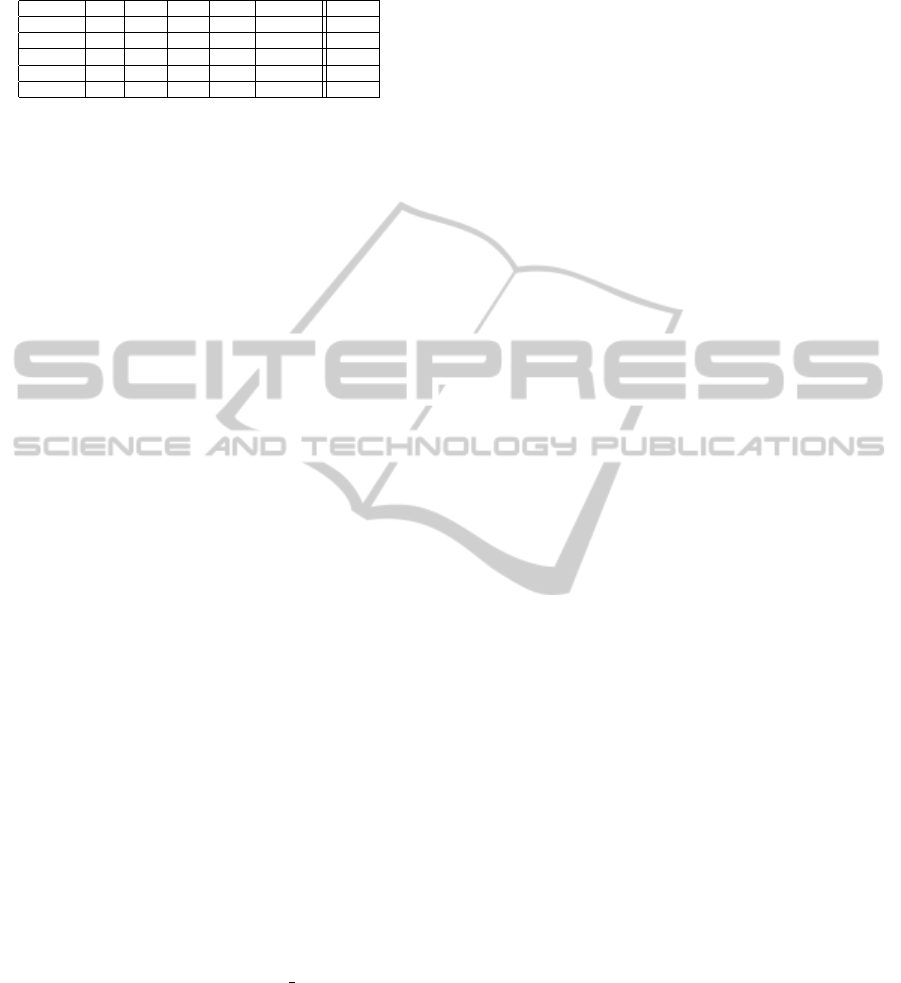
Table 2: Confusion matrix of classification results. Main
diagonal: correct rate. Off diagonal: misclassification rates.
79% coast forest street in. city highways no class
coast 84% 16%
forest 96% 4%
street 72% 12% 4% 12%
in. city 8% 76% 16%
highways 24% 12% 64%
4 DISCUSSION
In this paper we presented a biologically plausible
scheme for gist vision or scene categorisation. The
model proposed is strictly bottom-up and data-driven,
employing state-of-the-art cortical models for feature
extractions. Scene classification is achieved by a hi-
erarchy of grouping and gating cells with dendritic
fields, with local to global processing, also imple-
menting a sort of decision tree at the highest cell
level. The proposed scheme can be used to bootstrap
the process of object categorisation and recognition,
in which the same multi-scale cortical features are
employed (Rodrigues and du Buf, 2009a). This can
be done by biasing scene-typical objects in memory,
likely in concert with local gist vision and spatial lay-
out, i.e., which types of objects are about where in the
scene, but driven by attention. Although our model
of global gist does not yet yield perfect results, it is
already possible to combine it with a model of local
gist which addresses geometric shapes (Martins et al.,
2009).
In the future we have to increase the number of
test images and scene categories. This poses a prac-
tical problem because of the CPU time involved in
computing all multiscale features. This problem is be-
ing solved by re-implementing the feature extractions
using GP-GPUs.
ACKNOWLEDGEMENTS
Research supported by the Portuguese Foundation
for Science and Technology (FCT), through the
pluri-annual funding of the Inst. for Systems and
Robotics (ISR/IST) through the POS
Conhecimento
Program which includes FEDER funds, and by the
FCT project SmartVision: active vision for the blind
(PTDC/EIA/73633/2006).
REFERENCES
Bar, M. (2004). Visual objects in context. Nature Rev.:
Neuroscience, 5:619–629.
Bosch, A., Zisserman, A., and Munoz, X. (2009). Scene
classification via pLSA. Proc. Europ. Conf. on Com-
puter Vision, 4:517–530.
Fei-Fei, L. and Perona, P. (2005). A Bayesian hierarchi-
cal model for learning natural scene categories. Proc.
IEEE Comp. Vis. Patt. Recogn., 2:524–531.
Greene, M. and Oliva, A. (2009). The briefest of glances:
the time course of natural scene understanding. Cog-
nitive Psychology, 20(4):137–179.
Grossberg, S. and Huang, T. (2009). Artscene: A neural
system for natural scene classification. Journal of Vi-
sion, 9(4):1–19.
Lowe, D. (2004). Distinctive image features from scale-
invariant keypoints. Int. J. Comp. Vision, 2(60):91–
110.
Martins, J., Rodrigues, J., and du Buf, J. (2009). Focus of
attention and region segregation by low-level geome-
try. Proc. Int. Conf. on Computer Vision Theory and
Applications, Lisbon, Portugal, Feb. 5-8, 2:267–272.
Oliva, A. and Torralba, A. (2001). Modeling the shape of
the scene: a holistic representation of the spatial enve-
lope. Int. J. of Computer Vision, 42(3):145175.
Oliva, A. and Torralba, A. (2006). Building the gist of a
scene: the role of global image features in recognition.
Progress in Brain Res.: Visual Perception, 155:23–26.
Rodrigues, J. and du Buf, J. (2006). Multi-scale keypoints
in V1 and beyond: object segregation, scale selection,
saliency maps and face detection. BioSystems, 2:75–
90.
Rodrigues, J. and du Buf, J. (2009a). A cortical frame-
work for invariant object categorization and recogni-
tion. Cognitive Processing, 10(3):243–261.
Rodrigues, J. and du Buf, J. (2009b). Multi-scale lines and
edges in v1 and beyond: brightness, object categoriza-
tion and recognition, and consciousness. BioSystems,
95:206–226.
Ross, M. and Oliva, A. (2010). Estimating perception of
scene layout properties from global image features.
Journal of Vision, 10(1):1–25.
Tailor, D., Finkel, L., and Buchsbaum, G. (2000). Color-
opponent receptive fields derived from independent
component analysis of natural images. Vision Re-
search, 40(19):2671–2676.
Vogel, J., Schwaninger, A., Wallraven, C., and B¨ulthoff, H.
(2006). Categorization of natural scenes: Local vs.
global information. Proc. 3rd Symp. on Applied Per-
ception in Graphics and Visualization, 153:33–40.
Vogel, J., Schwaninger, A., Wallraven, C., and B¨ulthoff, H.
(2007). Categorization of natural scenes: Local versus
global information and the role of color. ACM Trans.
Appl. Perception, 4(3):1–21.
Xiao, J., Hayes, J., Ehinger, K., Oliva, A., and Torralba, A.
(2010). Sun database: Large-scale scene recognition
from abbey to zoo. Proc. 23rd IEEE Conf. on Com-
puter Vision and Pattern Recognition, San Francisco,
USA, pages 3485 – 3492.
A CORTICAL FRAMEWORK FOR SCENE CATEGORISATION
371