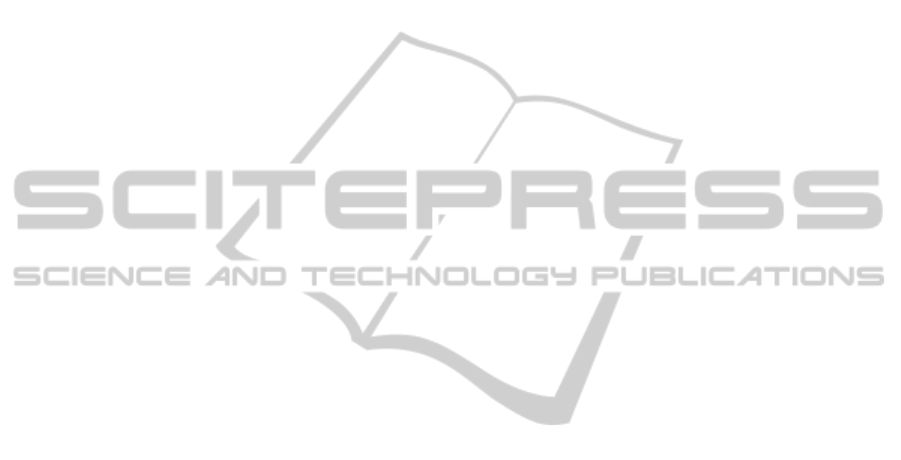
map, based on edge information, and eliminating the
noisy edges in the edge-map, based on region
information. The KSS algorithm works well and
solves the problem of false boundaries pointed out in
other works. Furthermore, all strong edges of both
input maps are held, improving the boundary
detection. Unfortunately, the KSS results present
broken edges, not keeping the contour closed.
The conclusion is that the two-level approach
proposed here improves the boundary detection
results, generating segmented images that match the
human perception better than the results associated
to the individual methods used in the architecture.
ACKNOWLEDGEMENTS
The authors would like to thank CAPES (Brazil) for
financial support.
REFERENCES
Chaudhuri, B. B.; Sarkar, N., 1995. Texture segmentation
using fractal dimension, IEEE Trans. Pattern Anal.
Mach. Intell., 17 (1), 72–77.
Côco, K. F., Salles, E. O. T., Sarcinelli-Filho, M., 2009.
Topographic independent component analysis based
on fractal and morphology applied to texture
segmentation, Lecture Notes in Computer Science,
5441, 491-498.
Deng, Y., Manjunath, B. S., 2001. Unsupervised
segmentation of color-texture regions in images and
video, IEEE Trans. Pattern Anal. Mach. Intell., 23 (8).
Komati, K. S., Salles, E. O. T., Sarcinelli-Filho, M., 2010.
Unsupervised color image segmentation based on local
fractal dimension, In Proc. 17th International
Conference on Systems, Signals and Image Processing
(IWSSIP 2010), 1, 243-246.
Komati, K. S., Salles, E. O. T., Sarcinelli-Filho, M., in
press. A Strategy for Image Boundary Detection
Combining Region and Edge Maps, Computing in
Science and Engineering, IEEE computer Society
Digital Library. doi: 10.1109/MCSE.2010.148.
Martin, D., Fowlkes, C., Tal, D., Malik, J., 2001. A
database of human segmented natural images and its
application to evaluating segmentation algorithms and
measuring ecological statistics, In Proc. 8th IEEE Int´l
Conf. Computer Vision, 2, 416–423.
Martin, D. R., Fowlkes, C. C., Malik, J., 2004. Learning to
detect natural image boundaries using local brightness,
color, and texture cues, IEEE Trans. Pattern Anal.
Mach. Intell., 26(5), 530–549, 2004.
Muñoz, X., Freixenet, J., Cufí, X., Martí, J., 2003.
Strategies for image segmentation combining region
and boundary information, IEEE Pattern Recognition
Letters, 24(1-3), 375–392.
Pentland, A. P., 1984 Fractal-based description of natural
scenes. IEEE Trans. on Pattern Analysis and Machine
Intelligence, 6.
Rotem, O., Greenspan, H., Goldberger, J., 2007.
Combining Region and Edge Cues for Image
Segmentation in a Probabilistic Gaussian Mixture
Framework. In Proc 2007 IEEE Conference on
Computer Vision and Pattern Recognition, 1-8.
Torralba, A., Oliva, A., 2003. Statistics of natural image
categories, Institute of Physics Publishing:
Computation in Neural Systems, 14, 391-412.
VISAPP 2011 - International Conference on Computer Vision Theory and Applications
186