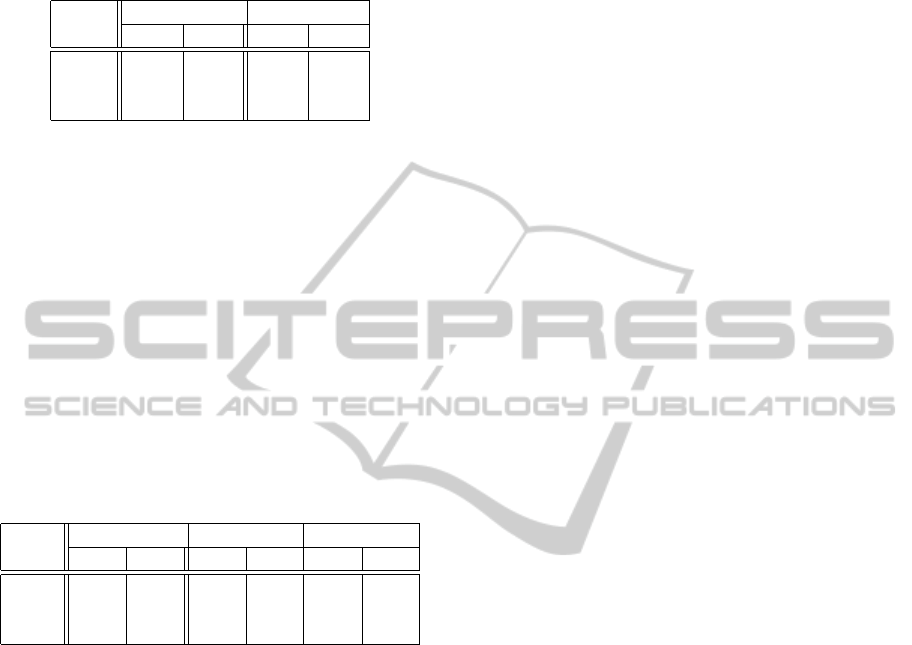
Table 1: Table shows the precision, recall and sensitivity
for the different evaluated classes, i.e., the musculo-skeletal
disorder (M), the normal pattern (N) and the parkinsonian
gait (P), using both the RBF and polynomial kernels.
Class Precision Recall
RBF Poly RBF Poly
M 0.67 0.75 0.33 0.75
N 0.6 0.7 0.95 0.66
P 0.72 0.61 0.41 0.64
call figures above 0.6, except for the musculo-skeletal
patterns, for which the RBF is 0.33, a very large dif-
ference that can be attributed to the fact that the group
of musculo-skeletal is composed of a larger number
of patterns and therefore the variance is much larger.
The RBF kernel shows a recall of 0.95 for the nor-
mal group, indicating that the RBF kernel works bet-
ter with the data with smaller variance. Of course
the fact that the normal group was the larger group (8
cases, compared with 7 and 6) can bias these results,
together with the fact that the chosen parameters were
set by the fact that they detected the larger number of
true positives.
Table 2: Confusion Matrix using RBF and polynomial ker-
nels for the three evaluated classes.
Class M N P
RBF Poly RBF Poly RBF Poly
M 4 9 5 1 3 2
N 1 2 20 14 0 5
P 1 1 8 5 8 11
Likewise the confusion matrix shows that correla-
tion between the nomal class is the higher.
5 CONCLUSIONS
This paper has introduced a novel markerless method
that allows to characterize normal and pathological
human gait patterns. The whole markerless strategy
consists in determining a feature vector for describing
normal and pathological movement, using a temporal-
spatial gait characterization from 3 differents views.
The feature vector is constructed by associating the
spatial information obtained from SURF and the tem-
poral information from a Σ − ∆ operator. Motion is
classified using a classical Support Vector Machine
strategy. Results demonstrate that this method can
complement the conventional gait analysis since it as-
signs objective pattern measurements. The method-
ology presented in this work constitutes a first ap-
proximation to understanding the complex dynamic
of the gait. From this kind of analyzes, we expect it
would be possible to set up an assembly of descriptors
which allow to accurately describe motions patterns
and quantify gait semantics.
REFERENCES
Cristani, M., Farenzena, M., Bloisi, D., and Murino,
V. (2010). Background subtraction for automated
multisensor surveillance: A comprehensive review.
EURASIP Journal on Advances in Signal Processing,
24:17.
Elgammal, A., Harwood, D., and Davis, L. (2000). Non-
parametric model for background subtraction. pages
751–767.
Flake, G. W. and Lawrence, S. (2001). Efficient svm regres-
sion training with smo.
Haiyan Luo, S. C. and et al., D. W. (2010). A remote mark-
erless human gait tracking for e-healthcare based on
content-aware wireless multimedia communications.
IEEE Wireless Communications,.
Herbert Bay, Andreas Ess, T. T. and Gool, L. V. (2008).
Speeded-up robust features (surf). Comput. Vis. Image
Underst, 110:346359.
Howe, N. R. and Deschamps, A. (2004). Better Foreground
Segmentation Through Graph Cuts. ArXiv Computer
Science e-prints.
Kamruzzaman, J. and Begg, R. K. (2006). Support vector
machines and other pattern recognition approaches to
the diagnosis of cerebral palsy. IEEETrans. Biomed.
Eng., 53:2479–2490.
Klempous, R. (2009). Biometric motion identification
based on motion capture. 243:335–348.
Manzanera, A. and Richefeu, J. (2007). A new motion
detection algorithm based on [sigma]-[delta] back-
ground estimation. 28(3):320–328.
McHugh, J., Konrad, J., Saligrama, V., and Jodoin, P.-M.
(2009). Foreground-adaptive background subtraction.
Signal Processing Letters, IEEE, 16(5):390 –393.
Perry, J. and Burnfield, J. M. (2010). Gait Analysis: Normal
and Pathological Function. NJ.Slack.
Turaga, P., Chellappa, R., Subrahmanian, V. S., and Udrea,
O. (2008). Machine recognition of human activities:
A survey. Circuits and Systems for Video Technology,
IEEE Transactions on, 18(11):1473–1488.
Viola, P. and Jones., M. J. (2004). Robust real-time face
detection. Int. J. Comput. Vision,, 57:137 154.
Wolf, S. and et al, T. L. (2006). Automated feature assess-
ment in instrumented gait analysis. Gait and Posture,
23:331–338.
PATHOLOGY CLASSIFICATION OF GAIT HUMAN GESTURES
713