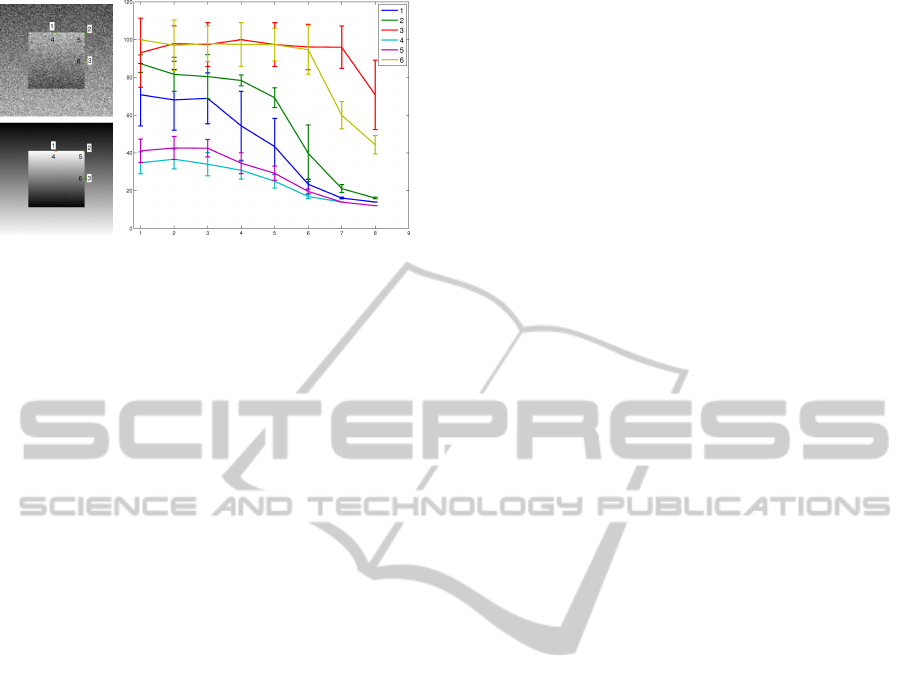
Figure 4: Adaptive scale obtained by ICI rule for the same
image with decreasing noises. Left column: test images
for SNR= 2 and SNR= 32. ‘1’,‘2’,‘3’ are points in Ω
o
;
‘4’,‘5’,‘6’ are the points in Ω
i
. Right column: estimated
kernel size error bars versus increasing SNR values for the
6 chosen points. SNR ∈ {.5,1, 2,4,8,16,25,32}. 20 values
are used for mean and variance estimation.
points (smiliar as in Fig. 1). A number in 1 to 6 is as-
signed to each point as shown in Fig. 4. The study is
carried out for the special case of an ideal segmenta-
tion (to discard the influence of bias estimation). In
order to obtain statistically meaningful estimations,
we run the experiment 20 times and for 8 different
SNR values. The means and the standard deviations,
calculated with the 20 estimated kernel sizes, are vi-
sualized as error bars versus increasing SNR values
on Fig. 4, a curve for every point.
First, we observe that the kernel size is inversely
proportional to the SNR value. Second, the variance
of the estimation is also inversely proportional to the
SNR value. These observations imply that, when the
image SNR increases, the corresponding optimal ker-
nel size decreases, and the proposed segmentation
method tends to be more local. Reversely, if the SNR
of image is small, the proposed method will adap-
tively use large kernel sizes. As expected, bigger ker-
nel sizes are obtained for the exterior points in com-
parison to their adjacent interior points. The pairs of
points ‘3’ and ‘6’ have the largest kernel estimated
sizes. Notice that for SNR values ≤ 8 dB, the ob-
tained sizes are almost equal to the maximum value
of the set h. This implies that the behavior of the
proposed method on such situation will be similar to
global methods, but locally. However, around points
‘1’ and ‘4’ the proposed method will always use local
image statistics. The curves also show that the noise
level has small influence on the estimated kernel size
at this location. This can be explained by the higher
inhomogeneity of the noise free image at this position.
In other words, the optimal scale is more governed by
the bias of the local region.
6 CONCLUSIONS
In this paper, we proposed an adaptive local region-
based segmentation method within the level set
framework. To our knowledge, this is the first work
for data driven local scale selection, in the context of
region based level set segmentation. The ICI rule is
used to derive an optimal scale for interior and exte-
rior points of the segmentation contour. The optimal-
ity is in the sense of the mean-square error minimiza-
tion of a Local Polynomials Approximation of the ob-
served image conditional on the current segmentation.
Although the presented results are preliminary, they
however, illustrate well the improvement on the state
of the art of segmentation methods. The continued
development and refinement of the proposed method,
with more experiments, should be researched in the
foreseeable future.
ACKNOWLEDGEMENTS
The authors would like to thank the Chinese Scholar-
ship Council for its financial support.
REFERENCES
Boukerroui, D., Baskurt, A., Noble, A., and Basset,
O. (2003). Segmentation of ultrasound images–
multiresolution 2D and 3D algorithm based on global
and local statistics. Pattern Recognit. Lett., 24:779–
790.
Boukerroui, D., Basset, O., Baskurt, A., and Noble, A.
(1999). Segmentation of echocardiographic data.
Multiresolution 2D and 3D algorithm based on gray
level statistics. In MICCAI, pages 516–524, Cam-
bridge, England. Springer-Verlag.
Brox, T. and Cremers, D. (2009). On local region mod-
els and a statistical interpretation of the piecewise
smooth Mumford-Shah functional. Int. J. Comput.
Vis., 84(2):184–193.
Chan, T. F. and Vese, L. A. (2001). Active contours without
edges. IEEE Trans. Image Process., 10(2):266–277.
Katkovnik, V., Egiazarian, K., and Astola, J. (2002). Adap-
tive window size image de-noising based on intersec-
tion of confidence intervals (ICI) rule. J. Math. Imag.
Vis., 16(3):223–235.
Katkovnik, V., Egiazarian, K., and Astola, J. (2006). Lo-
cal approximation techniques in signal and image pro-
cessing. The Int. Society for Optical Eng., Washing-
ton.
Katkovnik, V., Foi, A., Egiazarian, K., and Astola, J.
(2010). From local kernel to nonlocal multiple-model
image denoising. Int. J. Comput. Vis., 86:1–32.
IMAGAPP 2011 - International Conference on Imaging Theory and Applications
92