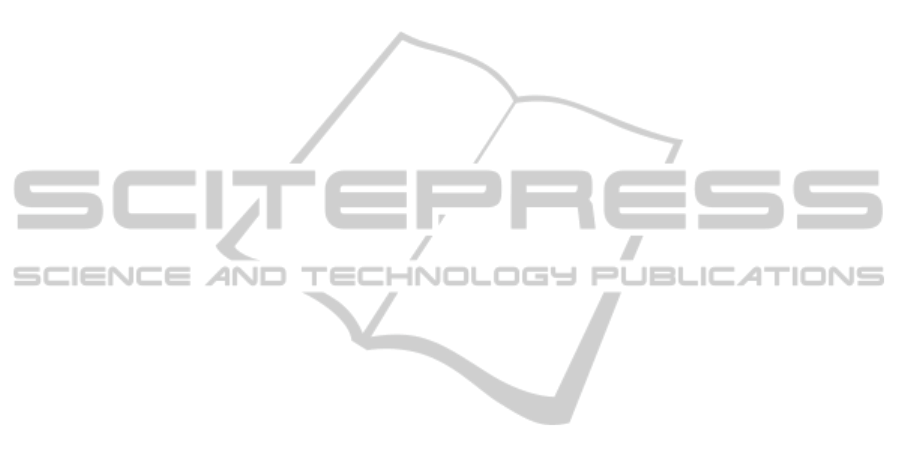
few limiting factors that may pose major challenges
towards the implementation of the context-
dependent opinion retrieval approach. By identifying
these factors, more research opportunities are
created for opinion retrieval. Existing approaches
can be improved such that effective opinion retrieval
techniques can be achieved in the long-run.
6.1 Dependency Among
Opinionated Sentences
Each sentence in an opinionated document may on
its own represent certain degree of opinion.
However, some sentences depend on prior (i.e.
sentence before) or latter (i.e. sentence after)
sentences in order to capture adequate opinion being
expressed. Detecting context-dependent opinion at
sentence-level could as well include looking into
dependencies between sentences
(Bermingham and
Smeaton, 2009). Therefore, opinion retrieval
techniques could consider multi-dependency in
opinionated sentences.
6.2 Multi-lingual Analysis
Opinionated documents appear in different types of
languages (Bruce et al, 2009). Unfortunately, many
research works avoid non-English opinionated
documents (Kim et al, 2010), while few research
works performed bi-lingual analysis of opinions. For
example in blogs, contributors have different lingual
backgrounds (Bruce et al, 2009), which is why
detecting collective opinion without lingual
differences is still a general challenge. Therefore,
future research works should be aware of this
limitation and its significance to the overall opinion
retrieval task.
7 SUMMARY & FUTURE WORK
Challenges in state-of-the-art opinion retrieval
techniques were reviewed. The cause of major
challenges can be summarized as ineffective way of
detecting context-dependent opinion at a lesser
computational cost. Opinions are context-dependant
and a grammar-based opinion retrieval technique is
can solve the above mentioned problems. In this
paper, we proposed grammar-based approach for
detecting context-dependent opinion by using CCG.
This approach can be quite useful for faceted-
opinion retrieval and personalized web search. In
our future work, we plan to implement our sentence-
level contextual model for opinion retrieval task.
REFERENCES
Bermingham, A. and Smeaton, A.F. (2009). A study of
inter-annotator agreement for opinion retrieval. In
SIGIR'09: Proceedings of the 32nd international
conference on Research and development in
information retrieval, pages 784-785. ACM.
Bruce, E. et al., (2009) Mapping the Arabic blogosphere:
politics, culture and dissent. Berkman Center for
Internet and Society at Harvard University.
Ding, X., Liu, B. and Yu, P.S. (2008). A holistic lexicon-
based approach to opinion mining. In Proceedings of
the international conference on Web search and web
data mining. Pages 231-240.ACM.
Hannah, D., Macdonald, C., Peng, J., He, B., Ounis, I.
(2007). University of Glasgow at TREC 2007:
Experiments in Blog and Enterprise Tracks with
Terrier. In TREC, 2007.
Baldridge , J.M., Kruijiff, G-J.M. (2004). Course Notes on
Combinatory Categorial Grammar.
Kim, J., Li, J-J. and Lee, J-H. (2010). Evaluating
multilanguage-comparability of subjectivity analysis
systems. In ACL'10: Proceedings of the 48th Annual
Meeting of the Association for Computational
Linguistics, pages 595-603.
Krahmer, E. (2010) What Computational Linguists Can
Learn from Psychologists. Association for
Computational Linguistics, 36(2): 285-294.
Liu, B. (2010) Sentiment Analysis and Subjectivity.
Handbook of Natural Language Processing, Second
Edition.
Sarmento, L., Carvalho, P., Silva, J.M., Oliveira, E. (2009)
Automatic creation of a reference corpus for political
opinion mining in user-generated content. In CIKM
'09: Proceeding of the 1st international CIKM
workshop on Topic-sentiment analysis for mass
opinion, pages 29-36. ACM.
Lv, Y. and Zhai, C. (2009). Positional language models
for information retrieval. In SIGIR'09: Proceedings of
the 32nd international conference on Research and
development in information retrieval, pages 299-306.
ACM.
Pang, B. and Lee, L. (2008). Opinion Mining and
Sentiment Analysis. In Found. Trends Inf. Retr.,2(1-
2): 1-135.
Zhai,C. (Statistical Language Models for Information
Retrieval: A Critical Review. In Found. Trends Inf.
Retr., 2(3):137–213.
Gerani, S., Carman, M.J. Crestani, F. (2010). Proximity-
Based Opinion Retrieval. In SIGIR, page 978. ACM.
Siersdorfer, S., Chelaru, S. and Pedro, J.S. (2010). How
Useful are Your Comments? Analyzing and Predicting
YouTube Comments and Comment Ratings. In
International World Wide Web Conference, pages
891-900.
Wei, Z. and Clement, Y. (2006). UIC at TREC 2006 Blog
Track, In TREC, 2006.
WEBIST 2011 - 7th International Conference on Web Information Systems and Technologies
610