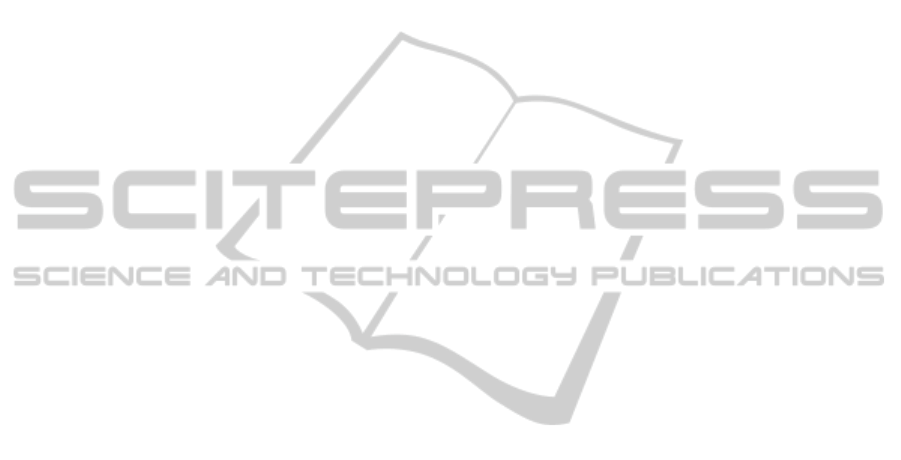
gical focus of the system. In accuracy-focused sys-
tems, emphasis on the reduction of grammar errors
seems to motivate system designers to use unam-
biguous, explicit feedback. The German Tutor and
CALLJ systems use metalinguistic descriptions to
provide error-specific feedback that includes explana-
tions, such as in the example “Watch out! The verb
WOHNEN and the subject ICH do not agree. ICH is
singular” from the German Tutor. Other options are
explicit recasts or clarification requests which omit
detailed metalinguistic language and instead supply
corrected versions of an utterance. An example of
this kind of feedback from the Te Kaitito system is
“There’s a mistake in that sentence. Maybe you mean
-suggested-correction-? Let’s try again”.
For fluency-focused systems, we observed that
there is a tendency towards implicit feedback, feed-
back that is less recognizably corrective and more eas-
ily integrated into the simulation. Perhaps the most
simple example can be found in TLCTS, in which
characters respond to errors with simple (and perhaps
realistic) requests for clarification such as “Sorry, I
don’t understand”. A second example, this time of an
implicit recast from the Bergenholtz system, can be
seen in the following interaction: “Student: How old
years is Dirk? System: How old is Dirk? Dirk is 30
years (old)”. An explanation for the tendency towards
implicit feedback is that fluency-focused practice ex-
ercises are designed to be authentic and that frequent
error correction with overly explicit feedback such as
metalinguistic descriptions may detract from the real-
ism of the simulation.
A second factor that influences feedback in
ICALL systems is technical feasibility. Reliable per-
formance of the language and speech processing mod-
ules that detect and classify errors is important, as
poor performance may result in errors going unno-
ticed or the potentially worse case of errors being re-
ported for well-formed utterances. For this reason,
system developers must take the limits of ASR com-
ponents into consideration when designing feedback
properties of a system. The current shortage of stud-
ies investigating the reliability of corrective feedback
for grammar in ASR-enabled systems prevents strong
conclusions, but existing studies suggest that correc-
tive feedback is more reliable in constrained practice
of the type seen in accuracy-focused systems. Perfor-
mance results for CALLJ suggest adequate reliabil-
ity, while data for the SPELL and Let’s Go systems
suggest otherwise, leading the authors of the first sys-
tem to state that performance is “not high enough to
support precise analysis of learner errors” (Anderson
et al., 2008, p.616).
In some systems, feedback properties may also be
affected by the proficiency level of the learner. In the
next section, we look at how feedback and other as-
pects of the system can be adapted to meet individual
learner needs.
3.3 Individualized Instruction
Computer systems have excellent logging capabili-
ties which can be used to make detailed recordings
of the interaction between the system and the learner.
ICALL systems employ student models to organize
the information they collect into data structures that
can capture different information about the learner.
The type of information captured in a student model
varies between systems, but some of the more com-
mon types concern study activity, including lessons
completed and time on task, and performance on ex-
ercises and quizzes (for examples, see TLCTS and
the German Tutor). Additionally, some systems la-
bel practice exercises with metadata related to peda-
gogical objectives, with the benefit being that results
of completed exercises can then be taken as an indi-
cation of the degree of mastery with respect to the
objectives. Examples of metadata types are linguistic
items, such as particular classes of words or gram-
matical constructions (Heift and Nicholson, 2001), or
task-oriented skills the learner is expected to acquire
(Johnson and Valente, 2009). More advanced student
models, in addition to the above, also capture type
and frequency information about errors the learner
produces, such as spelling, word order, and specific
grammar errors (Heift and Nicholson, 2001).
On its own, the information captured by a student
model is valuable to the student and educators as a
form of summative feedback which can serve as an
indicator of progress towards the goals of the system.
However, the most advanced systems go one step fur-
ther and use this information to adapt different aspects
of the system with the aim of optimizing the instruc-
tion to a learner’s specific needs. For example, the
German Tutor uses a student model to adjust proper-
ties of the feedback by varying the amount of detail
that is included in the error message according to the
learner’s skill level. Other uses of the student model
in this system include guiding decisions about what
errors to provide feedback on when multiple errors
are detected in learner input and generation of reme-
dial exercises based on observed student difficulties.
Summarising our observations, we find that prac-
tice in the systems surveyed can be grouped into
accuracy- and fluency-focused systems. Practice in
both groups provides opportunities for written and
spoken output. Feedback types differ, with grammar-
focused systems use explicit feedback to point out er-
CSEDU 2011 - 3rd International Conference on Computer Supported Education
358