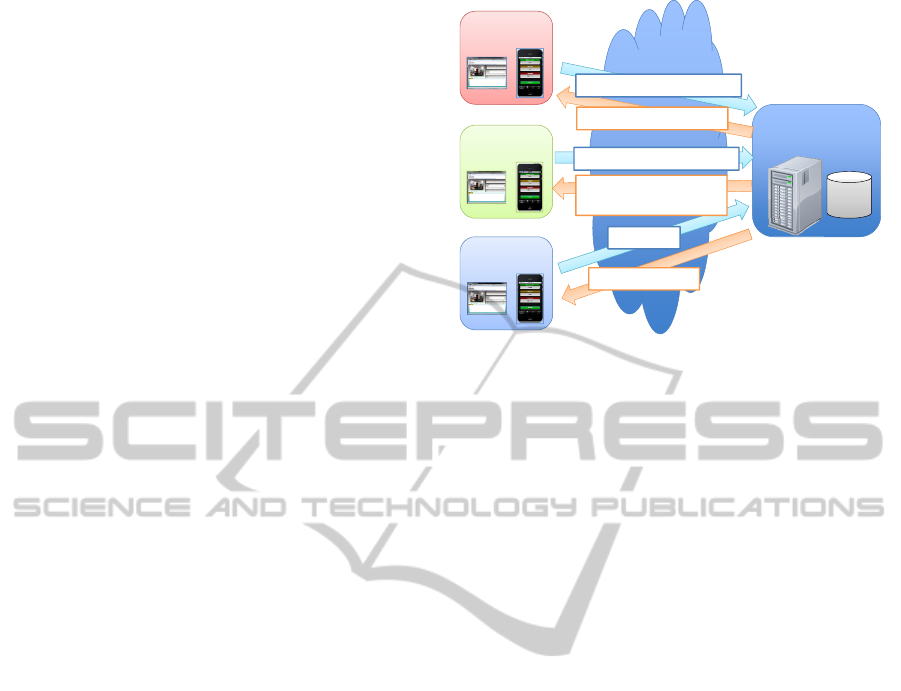
2 RELATED WORK
Activity recognition requires a large amount of
training data such as labels and accelerometer data.
There is already a work for gathering large-scale
activity information by using the previous ALKAN.
(Hattori et al., 2010). ALKAN can collect a large
amount of accelerometer data and label data from
mobile device such as iPhone/iPod Touch to the
server. However, ALKAN does not bring a benefit
to user, because ALKAN only has a feature of data
gathering. In ALKAN2 system, every user can enjoy
contents include movies, and contents provider can
get response and reputation from other users, like as
other CGM (Consumer Generated Media) system
represented by YouTube or other video sharing
system. Additionally, ALKAN cannot gather a broad
spectrum of activities, because a user can only select
from pre-set activities. In ALKAN2 system, we
permit users to define actions that they want or
require. This is because ALKAN2 can gather a
broad spectrum of activities.
In the literature, Berchtold analyzes ten type of
activity using mobile devices and a server. However,
they analyze only three activities per attachment
position. Berchtold et al. proposes a system for
activity recognition service using mobile phones and
cloud computers. (Berchtold and Buddle, 2010). The
proposed system provides activity recognition
service to mobile phones, as well as it evolves the
recognition model gathering the data to the cloud
computer.
Although our system does not provide activity
recognition so far, we propose another kind of
reasons to use the system for users by mimics of
actions. Moreover, our system can also be extended
to activity recognition service when the data are
gathered massively. Thus, our system can be
positioned as a new approach to gather training data
for evolving recognition models.
3 ALKAN2 SYSTEM
Fig.1 shows ALKAN2 system overview. The system
consists of smart phone software and information
gathering server. In ALKAN2 system, users collect
and upload acceleration sensor data with smart
phones. They also record and upload video data
through web browsers on client PCs. Users also
watch activity data and make evaluations on them.
In ALKAN2, we gather information of where the
smartphone
is, such as waist, held in hand or in a
Figure 1: ALKAN2 system overview.
pocket. If we gather an adequate amount of location
information, we can recognise the location of
smartphone from sensor data. Furthermore, we can
recognise human activity regardless of its location.
ALKAN2 system is suitable for gathering
characteristic activity information, such as step of
dances, forms of sports and other gestures, because
everyone can valuate such activities with watching
video of them. We apply ALKAN2 system to
training of darts, practice of dance and training of
manners. We also expect that we can apply
ALKAN2 system to job training such as medical
care, agricultural work and guidance of health care.
3.1 User Roles
In ALKAN2, we classify the user roles into the
following 3 types, in which any user can switch to
any role:
3.1.1 Provider
A user with role “provider” creates a new activity.
Firstly, Providers collect sensor data using a
smart phone and records video using a camera.
Then, uploads them to the server, and finally, binds
the sensor and video data as activity information the
Providers can receive evaluations of for the activity
from Viewers.
We consider sports trainer, work instructor, and
dancer who wants to bring her/his dance into vogue
as Providers. They are valued their activity from
many people, mimicked by Mimics as described
later. Eventually, they can earn a reputation, and
lead the trend in users’ community.
Provider
Mimic
Viewer
Internet
ALKAN
Server
Sensordata&moviedata
Sensordata&moviedata
EvaluationfromViewer
EvaluationfromViewer
Evaluationscore
Activitycontents
Evaluation
PECCS 2011 - International Conference on Pervasive and Embedded Computing and Communication Systems
558