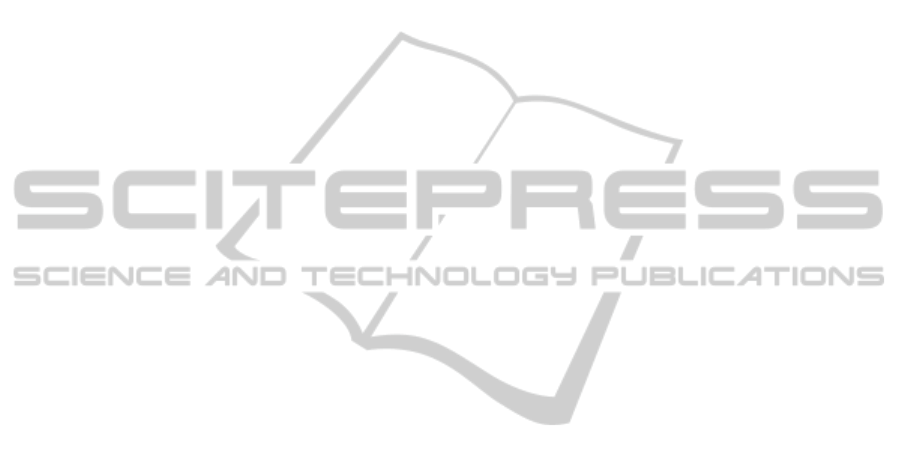
2 CONSTRUCTION OF
WARNING MODEL FOR
COMMERCIAL BANK
PERSONAL LOAN DEFAULT
RISK
Commercial bank personal loans default risk
warning model is to analyze the characteristics of
individual loans to impact on the probability of
default. Dependent variable is whether the breach of
contract, 1 default, 0 compliance. Default means an
individual cannot repay the loan principal and
interest on due, including subprime loans, doubtful
loans and loss loans. Subprime loan is borrower's
repayment ability apparent problem, totally
dependent on their normal income cannot guarantee
full repayment of loan principal and interest, even if
the guarantee may also cause some loss; Doubtful
loan is borrower cannot full repay the loan principal
and interest, even if the collateral or security, but
also sure to cause greater loss; Loss loan is taking all
possible measures and all necessary legal
procedures, the loan principal and interest is still not
recovered, or can only recover a very small part.
Compliance refers to a personal debt to maturity,
including the normal loans and interest loans.
Normal loan is the borrower to fulfill contract, there
is not enough reason to doubt the timely and full
repayment of loan principal and interest. The interest
loan is although the borrower has ability to repay the
loan principal and interest currently, but there are
some possible negative impact factors on the
repayment. To understand default standards, the key
is to grasp the core of possibility. Different levels of
inherent loans risk, the repayment probability is
different. The classification of loan risk divided into
the breach of default and undefault by the possibility
of repayment, and in order to reveal the true value of
the loan.
Personal loan risk including: qualification of
unqualified borrowers; borrowers to apply the
information false or non-compliance or not
complete. Personal credit information system is not
perfect now, banks cannot fully assess the
borrower's credit and solvency, the borrower may be
intentional fraud, fraudulent bank loans through
forgery of personal credit information, the bank
suffered financial loss; Risk posed by repayment
ability of borrowers reduce. There are many
personal loans are long-term loans, the borrower’s
repay ability decline is very likely to occur, could be
transformed into the bank's loan risk. Personal loan
default factor is related to characteristics of
individual loans, including age(A), education
level(B), length of service(C), residence(D), family
income(E), loan income ratio(F), credit card debts
(G), other debts(H), sex, the value of fixed assets,
loan term, whether mortgage, the family structure.
Education levels are usually inversely proportional
to the individual loan default, the higher the
education level, the smaller the likelihood of default;
The relationship between age and the default may
change with age; the higher length of service, the
probability of default should be smaller; The longer
the Living, the possibility of default should be
smaller; the higher family income, the probability of
default should be smaller; the higher loans income
ratio, the probability of default should be higher; the
more credit card debts, the higher probability of
default; the higher other debt, the more likelihood of
default; Women prefer stable, less likely to select
default than men; The more value of fixed assets, the
probability of default should be smaller; the longer
the loan period, the greater the likelihood of default;
The higher value of collateral, the smaller likelihood
of default; usually the more stable family structure,
the smaller likelihood of default.
3 APRIORI ALGORITHM OF
ASSOCIATION RULES
Association rules is to identify the data at a time or
things will appear: If item A is a part of the event,
the item B also appears in the probability of event
X%. Association rules with a specific set of
conditions linked to conclusions. Association rules
algorithm to automatically find those visualization
techniques can be found through the association,
such as web nodes, the advantage may exist in the
data associated with any attribute, it tries to find out
the number of rules, each rule can draw a
appropriate conclusions; The disadvantage is that it
can be very large, in an attempt to find model search
space, will cost a long time. It uses a generation-test
method to find the rules - simple rules originally
generated, and was the data set proved to be
effective. Good rules are stored, all the rules are
subject to different constraints, and then be
specialized. Specialization is a condition of
accession to the rules of procedure. These new rules
are then the data proved to be effective, then the
process is repeated to store to find the best or the
most significant rules. Users often a prerequisite for
the number of rules that may make some restrictions.
Or an effective indexing mechanism based on
ICEIS 2011 - 13th International Conference on Enterprise Information Systems
130