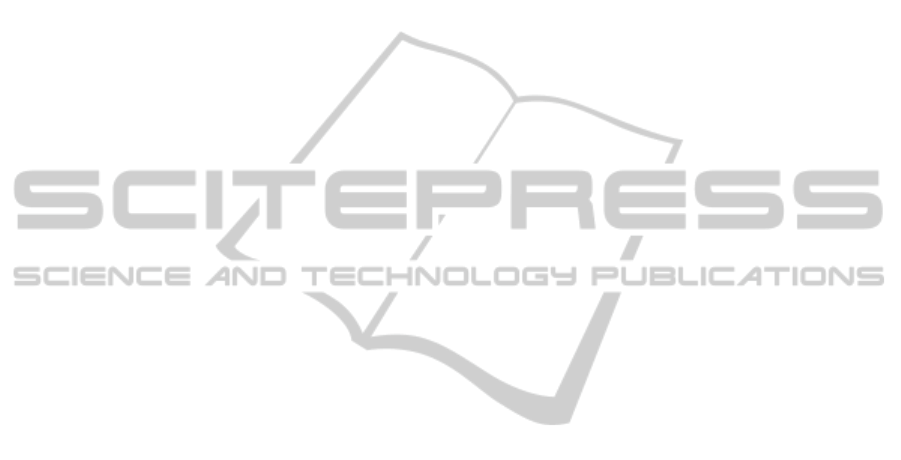
ploiting our involvement in a European industrial re-
search project: MUSING. In this way, we had at our
disposal an integrated framework and a real set of
data. Our analysis tool mainly solves, in this domain,
the problem of the availability of the expert knowl-
edge. In fact, in the economic field, obtaining a cog-
nitive net of relationships from experts is a hard task,
either for the complexity of the matter, or for the lack
of specific studies (very often these rules are based on
the expert believes or his/her own experience).
A final consideration deals with the application
fields and the system extension. In the paper, we fo-
cused on the economic domain using the IRs for aug-
menting a set of “similar” (for meaning, structure and
objective) rules. Nevertheless, it is important to point
out that the system is fully general and can be used in
several domains i.e. in all the domains that can be de-
scribed by an ontology and where instances are avail-
able. Moreover, the new extension further enriches
the system, making the IRs much more informative
and interesting than before.
ACKNOWLEDGEMENTS
This paper was supported by MUSING Project (IP
FP-027097) which provided an useful and convenient
framework.
REFERENCES
(2006). Musing Project - http://www.musing.eu/.
Baglioni, M., Bellandi, A., Furletti, B., Spinsanti, L., and
Turini, F. (2008). Ontology-based business plan clas-
sification. In EDOC 08: Proceedings of the 2008
12th International IEEE Enterprise Distributed Ob-
ject Computing Conference, pages 365–371.
Baglioni, M., Furletti, B., and Turini, F. (2005). Drc4.5:
Improving c4.5 by means of prior knowledge. In SAC
05: Proceedings of the 2005 ACM symposium on Ap-
plied computing, pages 474–481.
Bellandi, A., Furletti, B., Grossi, V., and Romei, A. (2007).
Pushing constraints in association rule mining: An
ontology-based approach. In Proceedings of the
IADIS International Conference WWW/INTERNET.
Bellini, L. (2007). Yadt-drb: Yet another decision tree do-
main rule builder. Masters Thesis.
Bonchi, F., Giannotti, F., Lucchese, C., Orlando, S., Perego,
R., and Trasarti, R. (2006). Conquest: a constraint-
based querying system for exploratory pattern discov-
ery. In ICDE.
Ciaramita, M., Gangemi, A., Ratsch, E., Saric, J., and Ro-
jas, I. (2008). Unsupervides learning of semantic re-
lations for molecular biology ontologies. In Ontology
Learning and Population: Bridging the Gap between
Text and Knowledge.
Elsayed, A., El-Beltagy, S. R., Rafea, M., and Hegazy, O.
(2007). Applying data mining for ontology building.
In In the proceedings of The 42nd Annual Conference
On Statistics, Computer Science, and Operations Re-
search.
Furletti, B. (2009). Ontology-driven knowledge dis-
covery. Ph.D. Thesis: http://www.di.unipi.it/˜
furletti/papers/PhDThesisFurletti2009.pdf.
Geller, J., Zhou, X., Prathipati, K., Kanigiluppai, S., and
Chen, X. (2005). Raising data for improved support
in rule mining: How to raise and how far to raise. In
Intelligent Data Analysis, volume 9, pages 397–415.
Kleinberg, J. (1998). Authoritative sources in a hyperlinked
environment. In ACM-SIAM Symposium on Discrete
Algorithms, pages 668–677.
Parekh, V., Gwo, J., and Finin, T. (2004). Mining domain
specific texts and glossaries to evaluate and enrich do-
main ontologies. In Proceedings of the International
Conference of Information and Knowledge Engineer-
ing.
Quinlan, J. (1993). C4.5: programs for machine learning.
Morgan Kaufmann Publishers Inc.
Singh, S., Vajirkar, P., and Lee, Y. (2003). Context-based
data mining using ontologies. In Conceptual Model-
ing - ER 2003, volume 2813.
Vela, M. and Declerck, T. (2008). Heuristics for automated
text-based shallow ontology generation. In Proceed-
ings of the International Semantic Web Conference
(Posters & Demos).
APPENDIX
The qualitative questionnaire aims at collecting the
qualitative information of the company/financial
institution that accesses the Online Self Assessment
service. Here is the list of questions and the corre-
sponding answers.
For being processed, the questionnaire has been
suitable codified. In the ontology, at the schema level,
each question is a datatype property of a concept.
The codification, with the syntax
Concept.datatypeProperty
, is also provided.
• Diversification of Production.
1. The company operates in more than one sector.
2. The company operates in just one sector with
flexible production processes.
3. The company operates in just one sector with
no flexible production processes.
DiversificationOfProduction.hasDivOfProdValue
• Commercial Diversification.
1. Customers base well diversified, with no con-
centration of sales.
ICSOFT 2011 - 6th International Conference on Software and Data Technologies
332