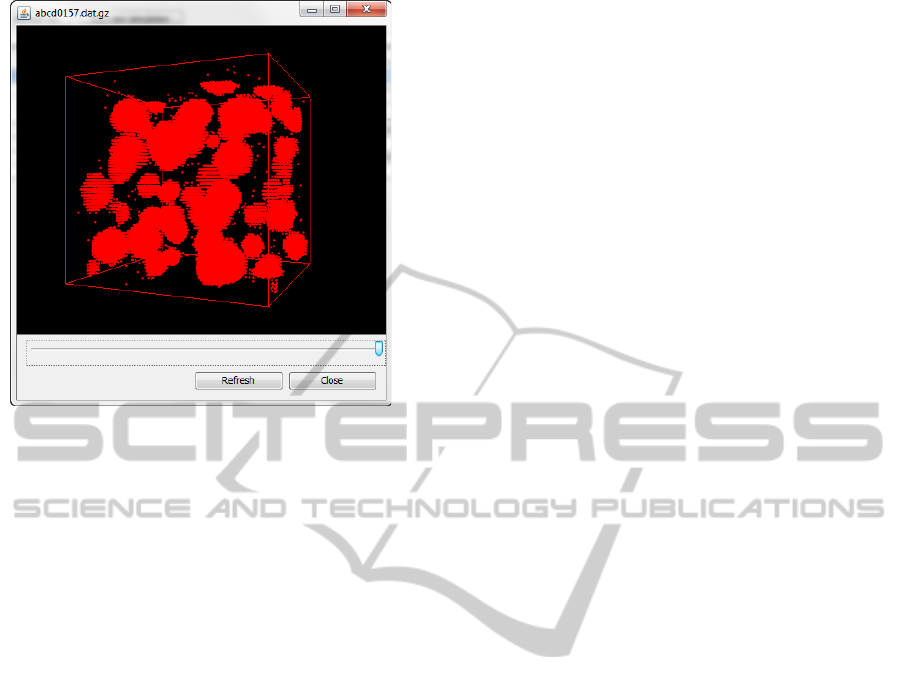
Figure 4: 3D preview of the simulation snapshots (Hotta,
2010).
4 CONCLUSIONS
In this paper we have presented a concept for an
infrastructure that enables the distributed execution
of scientific simulations. Core of the infrastructure
are simulation workflows that reflect the logic of
simulations and a resource manager that controls the
work distribution on the participating machines and
that works as storage for simulation data. The
infrastructure addresses main requirements of
scientists on a simulation environment and hence
can improve the tool support for scientific
simulations, e.g. to automate manual tasks, to enable
distributed execution of legacy software.
As a proof of concept we implemented an MC
simulation of solid bodies based on BPEL and tested
it with realistic data. The software improves the
simulation process and the former application to a
great extent. The scientists now have a GUI to start
and monitor their simulations, some of the manual
steps could be automated (e.g. start of post-
processing and visualization of results), and multiple
CPU cores and distributed computing can be
exploited. We are convinced that our consideration
can help scientists with their every day work.
ACKNOWLEDGEMENTS
The authors would like to thank the German
Research Foundation (DFG) for financial support of
the project within the Cluster of Excellence in
Simulation Technology (EXC 310/1) at the
University of Stuttgart. We thank Peter Binkele who
contributed the MC simulation code opal to our
work.
REFERENCES
Barga, R., Jackson, J., Araujo, N. et al., 2008. The Trident
Scientific Workflow Workbench. In Proc. of the IEEE
International Conference on eScience.
Binkele, P., Schmauder, S., 2003. An atomistic Monte
Carlo simulation for precipitation in a binary system.
In International Journal for Materials Research, 94,
pp. 1-6.
Deelman E., Blythe, J., Gil, Y. et al., 2004. Pegasus:
Mapping Scientific Workflows Onto The Grid. In
Proceedings of the 2
nd
European AcrossGrids
Conference, pp. 11-20, Springer-Verlag.
Foster, I., Kesselman, C., 2004. The Grid 2: Blueprint for
a New Computing Infrastructure. Morgan Kaufmann,
2
nd
edition.
Goerlach, K., Sonntag, M., Karastoyanova, D. et al., 2011.
Conventional Workflow Technology for Scientific
Simulation. In: Yang, X., Wang, L., Jie, W., 2011.
Guide to e-science. Springer-Verlag.
Hotta, S., 2010. Ausführung von Festkörpersimulationen
auf Basis der Workflow Technologie. Diploma Thesis
No. 3029, University of Stuttgart.
Kizler, P., Uhlmann, D., Schmauder, S., 2000. Linking
Nanoscale and Macroscale: Calculation of the Change
in Crack Growth Resistance of Steels with Different
States of Cu Precipitation Using a Modification of
Stress-strain Curves Owing to Dislocation Theory. In
Nuclear Engineering and Design, 196, pp. 175-183.
Leymann, F., Roller, D., 2000. Production Workflow –
Concepts and Techniques. Prentice Hall.
Molnar, D., Binkele, P., Hocker, S., Schmauder, S., 2010.
Multiscale Modelling of Nano Tensile Tests for
Different Cu-precipitation States in α-Fe. In:
Proceedings of the 5
th
International Conference on
Multiscale Materials Modelling, pp. 235-239,
Fraunhofer Verlag.
Schmauder, S., Binkele, P., 2002. Atomistic Computer
Simulation of the Formation of Cu-Precipitates in
Steels. Computational Materials Science, 24,
pp. 42-53.
Soisson, F., Barbu, A., Martin, G., 1996. Monte-Carlo
Simulations of Copper Precipitates in Dilute Iron-
Copper Alloys During Thermal Ageing and Under
Electron Irradiation. In Acta Materialia, 44,
pp. 3789-3800.
Sonntag, M., Karastoyanova, D., Deelman, E., 2010.
Bridging the Gap Between Business and Scientific
Workflows. In 6
th
IEEE International Conference on
e-Science.
ICSOFT 2011 - 6th International Conference on Software and Data Technologies
94