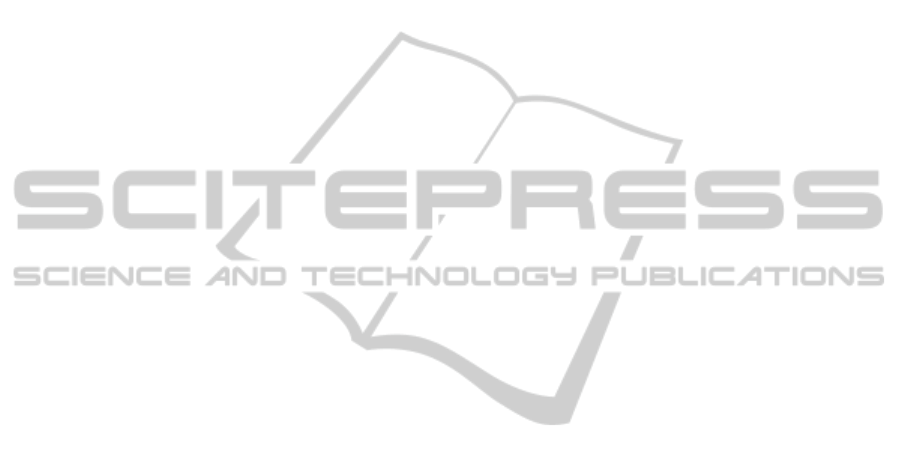
REFERENCES
Androutsopoulos, I., Koutsias, J., Chandrinos, K.,
Paliouras, G., and Spyropoulos, C. (2000a). An eval-
uation of naive bayesian anti-spam filtering. In Pro-
ceedings of the workshop on Machine Learning in the
New Information Age, pages 9–17.
Androutsopoulos, I., Koutsias, J., Chandrinos, K., and Spy-
ropoulos, C. (2000b). An experimental comparison
of naive Bayesian and keyword-based anti-spam fil-
tering with personal e-mail messages. In Proceedings
of the 23
rd
annual international ACM SIGIR confer-
ence on Research and development in information re-
trieval, pages 160–167.
Androutsopoulos, I., Paliouras, G., Karkaletsis, V., Sakkis,
G., Spyropoulos, C., and Stamatopoulos, P. (2000c).
Learning to filter spam e-mail: A comparison of a
naive bayesian and a memory-based approach. In Pro-
ceedings of the Machine Learning and Textual Infor-
mation Access Workshop of the 4
th
European Confer-
ence on Principles and Practice of Knowledge Dis-
covery in Databases.
Awad, A., Polyvyanyy, A., and Weske, M. (2008). Semantic
querying of business process models. In IEEE Inter-
national Conference on Enterprise Distributed Object
Computing Conference (EDOC 2008), pages 85–94.
Baeza-Yates, R. A. and Ribeiro-Neto, B. (1999). Mod-
ern Information Retrieval. Addison-Wesley Longman
Publishing Co., Inc., Boston, MA, USA.
Bates, M. and Weischedel, R. (1993). Challenges in natural
language processing. Cambridge Univ Pr.
Becker, J. and Kuropka, D. (2003). Topic-based vector
space model. In Proceedings of the 6th International
Conference on Business Information Systems, pages
7–12.
Blanzieri, E. and Bryl, A. (2007). Instance-based spam
filtering using SVM nearest neighbor classifier. Pro-
ceedings of FLAIRS-20, pages 441–442.
Bratko, A., Filipiˇc, B., Cormack, G., Lynam, T., and Zupan,
B. (2006). Spam filtering using statistical data com-
pression models. The Journal of Machine Learning
Research, 7:2673–2698.
Cano, J., Herrera, F., and Lozano, M. (2006). On the combi-
nation of evolutionary algorithms and stratified strate-
gies for training set selection in data mining. Applied
Soft Computing Journal, 6(3):323–332.
Carnap, R. (1955). Meaning and synonymy in natural lan-
guages. Philosophical Studies, 6(3):33–47.
Carpinter, J. and Hunt, R. (2006). Tightening the net: A
review of current and next generation spam filtering
tools. Computers & security, 25(8):566–578.
Carreras, X. and M´arquez, L. (2001). Boosting trees for
anti-spam email filtering. In Proceedings of RANLP-
01, 4th international conference on recent advances in
natural language processing, pages 58–64. Citeseer.
Cohen, D. (1974). Explaining linguistic phenomena. Hal-
sted Press.
Cranor, L. and LaMacchia, B. (1998). Spam! Communica-
tions of the ACM, 41(8):74–83.
Cruse, D. (1975). Hyponymy and lexical hierarchies.
Archivum Linguisticum, 6:26–31.
Czarnowski, I. and Jedrzejowicz, P. (2006). Instance reduc-
tion approach to machine learning and multi-database
mining. In Proceedings of the Scientific Session orga-
nized during XXI Fall Meeting of the Polish Informa-
tion Processing Society, Informatica, ANNALES Uni-
versitatis Mariae Curie-Skłodowska, Lublin, pages
60–71.
Dash, M. and Liu, H. (2003). Consistency-based search
in feature selection. Artificial Intelligence, 151(1-
2):155–176.
Dietterich, T., Lathrop, R., and Lozano-P´erez, T. (1997).
Solving the multiple instance problem with axis-
parallel rectangles. Artificial Intelligence, 89(1-2):31–
71.
Drucker, H., Wu, D., and Vapnik, V. (1999). Support vector
machines for spam categorization. IEEE Transactions
on Neural networks, 10(5):1048–1054.
Elkan, C. (2001). The foundations of cost-sensitive learn-
ing. In Proceedings of the 2001 International Joint
Conference on Artificial Intelligence, pages 973–978.
Heron, S. (2009). Technologies for spam detection. Net-
work Security, 2009(1):11–15.
Ide, N. and V´eronis, J. (1998). Introduction to the special
issue on word sense disambiguation: the state of the
art. Computational linguistics, 24(1):2–40.
Jagatic, T., Johnson, N., Jakobsson, M., and Menczer, F.
(2007). Social phishing. Communications of the ACM,
50(10):94–100.
Jung, J. and Sit, E. (2004). An empirical study of spam traf-
fic and the use of DNS black lists. In Proceedings of
the 4th ACM SIGCOMM conference on Internet mea-
surement, pages 370–375. ACM New York, NY, USA.
Karlberger, C., Bayler, G., Kruegel, C., and Kirda, E.
(2007). Exploiting redundancy in natural language
to penetrate bayesian spam filters. In Proceedings of
the 1
st
USENIX workshop on Offensive Technologies
(WOOT), pages 1–7. USENIX Association.
Kent, J. (1983). Information gain and a general measure of
correlation. Biometrika, 70(1):163–173.
Kohavi, R. (1995). A study of cross-validation and boot-
strap for accuracy estimation and model selection. In
International Joint Conference on Artificial Intelli-
gence, volume 14, pages 1137–1145.
Kołcz, A., Chowdhury, A., and Alspector, J. (2004). The
impact of feature selection on signature-driven spam
detection. In Proceedings of the 1
st
Conference on
Email and Anti-Spam (CEAS-2004).
Kuropka, D. (2004). Modelle zur Repr¨asentation
nat¨urlichsprachlicher Dokumente-Information-
Filtering und-Retrieval mit relationalen Datenbanken.
Advances in Information Systems and Management
Science, 10.
ANOMALY-BASED SPAM FILTERING
13