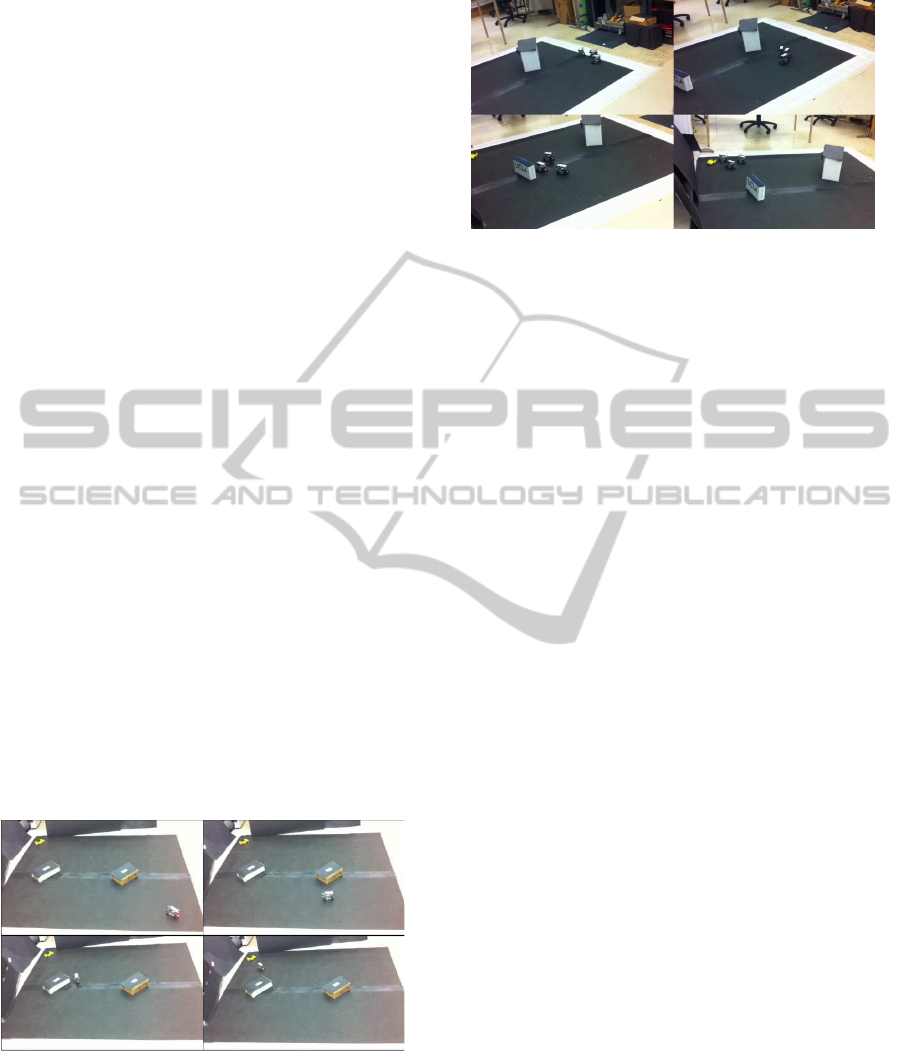
sensed, so that cars may occasionally turn into nearby
barriers that lie at their sides. We find, though, that the
relatively slow motion and large turning radius of the
cars minimizes these issues, and that swarms of cars
employing this algorithm, in conjunction with Eq. 4,
typically navigate the barriers successfully, as show
in Fig. 8
4 FUTURE WORK AND
CONCLUSIONS
Though the third generation of the AMLT has already
surpassed previous versions in terms of successfully
performed experiments, there are still many aspects
of the new hardware that have not yet been utilized.
Foremost amongst these is the potential for a true
peer-to-peer communication network for the vehicles.
Creating such a network would allow for the imple-
mentation of many advanced algorithms. For exam-
ple, it would allow for the vehicles in our daisy-chain
setup to determine their nearest neighbors in realtime,
decreasing the chances of collisions occurring.
Another avenue of exploration is greater usage of
the many on-board sensors. The optical encoder and
gyroscopes could allow each car to estimate its own
position over time with less reliance on the tracking
computer, assuming the car’s initial coordinates are
known. This would mimic the intermittent GPS out-
ages expected in field vehicles, and give methods for
dealing with them. Also, the on-board camera could
perhaps be used in conjunction with the IR sensor for
enhanced barrier detection, or possibly target detec-
tion when the location of the target is unknown.
Figure 7: Four frames of an experimental run of tracking
camera-assisted barrier avoidance, with the yellow object
in the top left of each frame as the target. The frames are
ordered from left to right, top to bottom. The car detects the
first barrier in the second frame and begins to avoid it. It
continues toward the target and avoids the second barrier in
frame three and reaches the target in frame four.
Figure 8: Four frames of an experimental run of IR sensor
barrier avoidance, with the yellow object near the top left of
frames three and four as the target. The frames are ordered
from left to right, top to bottom. The cars detect the first
barrier in the second frame and avoid it. They continue to-
ward the target and avoid the second barrier in frame three
and reach the target in frame four.
ACKNOWLEDGEMENTS
This paper is supported by ARO MURI grant 50363-
MA-MUR and NSF grants DMS-0914856, DMS-
0907931, DMS-0601395, and EFRI-1024765.
REFERENCES
Azarnasab, E. and Hu, X. (2007). An integrated multi-robot
test bed to support incremental simulation-based de-
sign. In Proceedings of the IEEE International Con-
ference on System of Systems Engineering.
Bhattacharya, S., Michael, N., and Kumar, V. (2010). Dis-
tributed coverage and exploration in unknown non-
convex environments. In 10th International Sympo-
sium on Distributed Autonomous Robots. Springer.
Cruz, D., McClintock, J., Perteet, B., Orqueda, O. A. A.,
Cao, Y., and Fierro, R. (2007). Decentralized cooper-
ative control - a multivehicle platform for research in
networked embedded systems. Control Systems Mag-
azine, IEEE, 27(3):58 – 78.
Hermina Martinez, D. S. (2010). Integration of 3rd gener-
ation vehicles to the applied mathematics laboratory
autonomous micro-vehicle testbed. Master’s thesis,
Department of Electrical Engineering, University of
California, Los Angeles.
Hsieh, C. H., Chuang, Y. L., Huang, Y., Leung, K. K.,
Bertozzi, A. L., and Frazzoli, E. (2006). An eco-
nomical micro-car testbed for validation of coopera-
tive control strategies. In Proceedings of the American
Control Conference, pages 1446–1451.
Jin, Z., Waydo, S., Wildanger, E. B., Lammers, M.,
Scholze, H., Foley, P., Held, D., and Murray, R. M.
(2004). MVWT-II: the second generation Caltech
Multi-Vehicle Wireless Testbed. In Proceedings of the
American Control Conference, pages 5321 – 5326.
A THIRD GENERATION MICRO-VEHICLE TESTBED FOR COOPERATIVE CONTROL AND SENSING
STRATEGIES
19