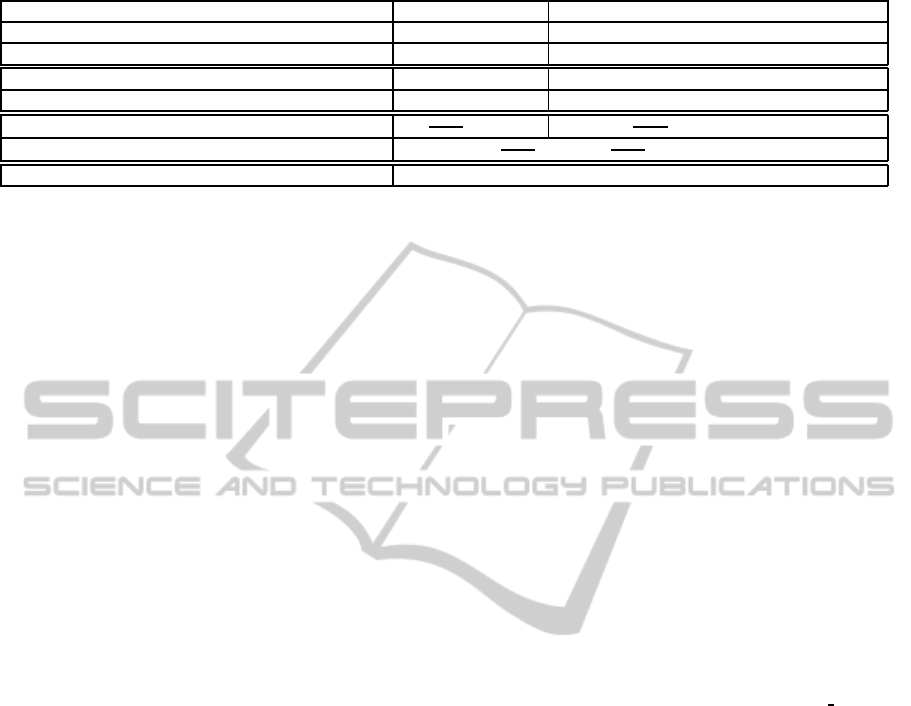
Table 2: Maximum possible savings through network planning improvements.
Current situation With our network planning improvement
Channels with a time-based pricing scheme 30 29
Channels with a flat-rate pricing scheme - 1
Cost per instance for a time-based pricing scheme X X
Cost with a time-based pricing scheme per instance - Y
Cost
48849
30
× 30X
47003
29
× 29X + 1846 ×Y
Saving
48849
30
× 30X −
47003
29
× 29X + 1846 ×Y
Saving for X = 10 and Y = 9 monetary units 35074
of the most widely applied algorithms. We could have
used other clustering algorithms and compared the re-
sults. The incremental clustering method is, however,
the only one that with our data generates an explicit
knowledge representation model that describes clus-
tering in such a way that it can be easily visualised
and understood (Witten and Frank, 2005).
We could have also incremented the granularity
of the data, splitting the date attribute by year, month
and day. Nevertheless, since the dataset in the exper-
iment corresponded to one month, this criterion did
not affect the results. We did not study whether or not
the attributes are interdependent. Thus, for this ex-
periment, all of the attributes were treated as covari-
ant; additionally, Bayesian-network-based algorithms
(Ruiz-Agundez et al., 2010b) may assist in the study
of such dependencies.
We have proposed an improvement in network
planning that could result in savings regarding tele-
phone calls. The change consisted of adopting a flat-
rate pricing scheme for one of the telephone channels
(i.e., trunk lines) for a set of calls that would be more
expensive with a time-based pricing scheme. We have
contributed to improving the resource management
and the service tariffing of the experiment corpora-
tion, further, this methodology could be generalised
to any size corporation as the service usage data can
always be clustered.
Finally, it is worth highlighting that the most ex-
citing result of the proposed methodology is, in our
opinion, the ability to produce highly representative
results that could generate a high level of knowl-
edge. The benefits and possible applications of such a
methodology include: (i) user behaviour modelling,
(ii) fraud detection through anomalous behaviour
analysis (Ruiz-Agundez et al., 2010a), (iii) technical
and economical implications (e.g. network planning),
and (iv) pricing scheme modelling (Falkner et al.,
2009).
Future work includes analysis of clustered data
for use as training data for classification algorithms.
These algorithms should allow us to predict the val-
ues of certain attributes. For example, we should be
able to predict within a given probability a call’s du-
ration or the channel used to route the call given its
source and destination.
REFERENCES
Chang, X. and Petr, D. (2001). A survey of pricing for inte-
grated service networks. Computer communications,
24(18):1808–1818.
Chi, E. (2000). A taxonomy of visualization techniques
using the data state reference model. In Proc. of the
IEEE Symposium on Information Visualization, pages
69–75.
Falkner, M., Devetsikiotis, M., and Lambadaris, I. (2009).
An overview of pricing concepts for broadband IP net-
works. IEEE Communications Surveys, 3(2):2–13.
Keim, D. and Hinneburg, A. (1999). Clustering techniques
for large data sets from the past to the future. In Tu-
torial notes of the fifth ACM SIGKDD international
conference on Knowledge discovery and data mining,
pages 141–181. ACM.
Kim, W. (2009). Survey topic: Parallel Clustering Algo-
rithms. http://www.cs.gsu.edu/∼wkim/index files/
annotbib.pdf.
Nocke, T., Schumann, H., and Bohm, U. (2004). Methods
for the visualization of clustered climate data. Com-
putational Statistics, 19(1):75–94.
Ruiz-Agundez, I., Penya, Y. K., and Bringas, P. G. (2010a).
Fraud detection for voice over ip services on next-
generation networks. In Samarati, P., editor, Proceed-
ings of the 4th Workshop in Information Security The-
ory and Practices (WISTP 2010), LNCS 6033, pages
199–212, Passau, Germany. IFIP International Feder-
ation for Information Processing 2010, Springer.
Ruiz-Agundez, I., Penya, Y. K., and Bringas, P. G. (2010b).
Optimal bayesian network design for efficient intru-
sion detection. In Proceedings of the 3rd International
Conference on Human System Interaction (HSI 2010),
pages 444–451, Rzeszow, Poland. IEEE.
Snasel, V., Abraham, A., Owais, S., Platos, J., and Kromer,
P. (2010). User Profiles Modeling in Information
Retrieval Systems. Emergent Web Intelligence: Ad-
vanced Information Retrieval, page 169.
Sugar, C. and James, G. (2003). Finding the Number of
Clusters in a Dataset. Journal of the American Statis-
tical Association, 98(463):750–763.
Witten, I. H. and Frank, E. (2005). Data mining. Practical
machine learning tools and techniques. Diane Cerra.
DCNET 2011 - International Conference on Data Communication Networking
88