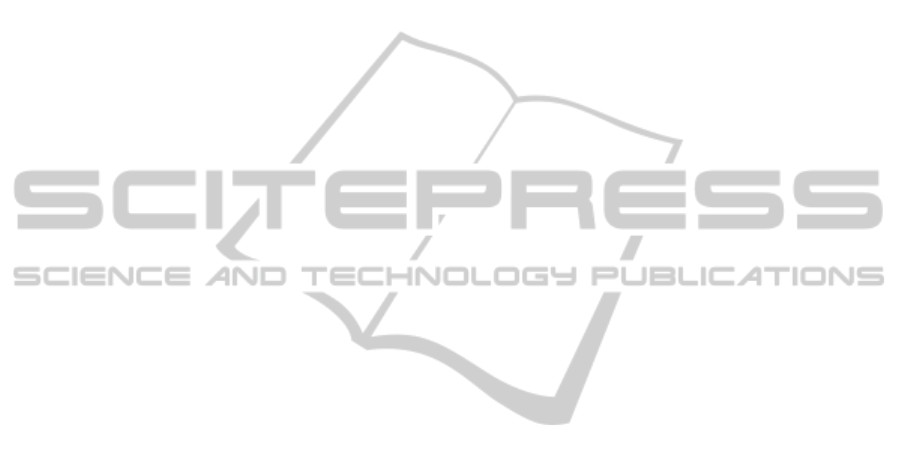
2003). In addition, according to one researcher, once
accommodation has been booked, the vacation
itinerary is relatively predetermined and fixed
(Hyde, 2008).
Survey respondents’ attitude of desirability
toward online travel booking is shaped by their
beliefs that online travel booking is safe using their
credit card, easy, enjoyable, and they highly regard
the importance of its convenience. A desirable
attitude coupled with a positive one are the key
variables leading to online travel booking intention.
Respondents believe it is more expensive and
inconvenient booking with an agent than a website.
Also, it is more enjoyable and easier booking with a
website.
Furthermore, in the Model, the variable “Internet
access other ~2” recorded an odds ratio of 1.589
meaning that the expected odds of booking travel
online (‘highly likely’ to book) is almost 1.6 times
greater among respondents indicating they had
access to the Internet asides from home or work,
than respondents who said they do not have such
access, controlling for all other factors in the model.
With categorical variables Stata creates k indicator
variable sets. The procedure is to omit the first group
of variables so it acts as a baseline for other
categories to help understand their odds ratios. Other
key variables from hypotheses tests H1g, H1i, H1j
and Hik are significant in this Final Model.
6 CONCLUSIONS
The predictive Final Model contains socio and
psychographic variables of the traveler’s decision
making process when booking travel products, and it
quantifies the strength of these variables through the
interpretation of odds ratios as indicated above. Such
a quantitative model for predicting travel products is
unique in travel and e-business literature and thus it
is a valued contribution.
In addition to uncovering these predictors, the
probability of group membership in each category
(online booking intention where, 1 = highly likely,
2 = likely, and 3 = somewhat likely) was determined
using logistic regression. When one combines these
probabilities with demographic parameters
(Conyette, 2011), it gives a website operator
valuable information for targeting consumers.
This study holds important strategic implications
for the travel industry, and the following are offered
to travel website operators and travel agents so they
will prosper in the new marketplace.
Some implications deal with consumers’ beliefs
and attitudes. Websites should note the perceptions
online consumers have that booking with a travel
website is positive. Understanding what particular
attributes of online shopping make people feel this
way is important. It could be that online bookers
regard the savings in time and money as a positive
benefit. Yet, these savings are not always
Table 1: Final Model.
realized by using a website, so the online retailer
should consider how to convey the belief that
savings will result. However, many online bookers
are more motivated by the rich information available
on the Web and the convenience of accessing it.
These bookers find the use of travel agents
inconvenient and probably inefficient. Offline
consumers often want the assistance and interaction
only a human could provide. Travel agencies are
best advised to make their services convenient,
efficient, useful and very personable.
Travel agents could be trained on the usage of
intelligent online tools and combine the assistance
these tools provide with the unique aspects of advice
that comes from a human touch (human
intelligence). Agents could think about inimitable
aspects of human knowledge, intelligence and
reasoning that cannot be currently provided by
online intelligent tools. Combining these methods in
novel and powerful ways will exceed the
expectations of consumers. Planning long haul or
complex trips seems to be the strength of travel
Ordered logistic regression Number of obs = 856
LR chi2(27) = 279.47
Prob > chi2 = 0.0000
Log likelihood = -709.04845 Pseudo R2 = 0.1646
------------------------------------------------------------------------------
Online booking intent| Odds Ratio Std. Err. z P>|z| [95% Conf. Interval]
-------------+----------------------------------------------------------------
Some of my friends _2 1.490089 .3075721 1.93 0.053 .9942923 2.233111
Some of my friends _3 2.635803 .5708602 4.47 0.000 1.724092 4.029633
Some of my friends _4 3.846541 1.003958 5.16 0.000 2.306239 6.415587
Belief convenientA_2 .8059347 .2195409 -0.79 0.428 .4725286 1.374585
Belief convenientA_3 .6573546 .1672914 -1.65 0.099 .3991864 1.082489
Belief convenientA_4 .6292642 .1552033 -1.88 0.060 .3880525 1.020412
Belief convenientA_5 .4359062 .1128951 -3.21 0.001 .262386 .724178
Belief convenientA_6 .5405856 .1592311 -2.09 0.037 .3034873 .9629159
Belief convenientA_7 .5648519 .1587146 -2.03 0.042 .3256568 .9797359
Belief expensiveA_2 1.037616 .2842878 0.13 0.893 .6064888 1.775212
Belief expensiveA_3 1.395234 .3590141 1.29 0.196 .8425983 2.310328
Belief expensiveA_4 1.234324 .3131459 0.83 0.407 .7507253 2.029447
Belief expensiveA_5 1.625916 .3987905 1.98 0.048 1.005363 2.629501
Internet access other~2 1.589834 .2889482 2.55 0.011 1.113392 2.270154
How long using net_2 .7402478 .1117285 -1.99 0.046 .5506833 .9950671
umber of trips~2 .6097605 .1305857 -2.31 0.021 .4007438 .9277945
Destination tour _1 .5910556 .1000929 -3.11 0.002 .4241122 .8237131
Hotels_1 .6086096 .122005 -2.48 0.013 .4108682 .9015193
Airline tickets_1 .5602512 .1240503 -2.62 0.009 .3630033 .8646794
Car rentals_1 .6281079 .1085975 -2.69 0.007 .4475738 .8814626
Long-distance train_1 .367288 .1489853 -2.47 0.014 .165855 .8133637
Attitude positiveW_2 1.654819 .3965239 2.10 0.036 1.034636 2.646754
Attitude positive W_3 1.968378 .5129192 2.60 0.009 1.181143 3.280305
Attitude positiveW_4 1.934779 .4825798 2.65 0.008 1.186647 3.154577
Attitude desirableW_2 1.188753 .25725 0.80 0.424 .7778375 1.816747
Attitude desirableW_3 1.6572 .3936764 2.13 0.033 1.040321 2.639868
Attitude desirableW_4 1.749352 .4169593 2.35 0.019 1.096459 2.791012
-------------+----------------------------------------------------------------
/cut1 -.0922823 .352925 -.7840026 .599438
/cut2 1.092407 .3544086 .3977792 1.787035
MODELING FACTORS THAT INFLUENCE ONLINE TRAVEL BOOKING
209