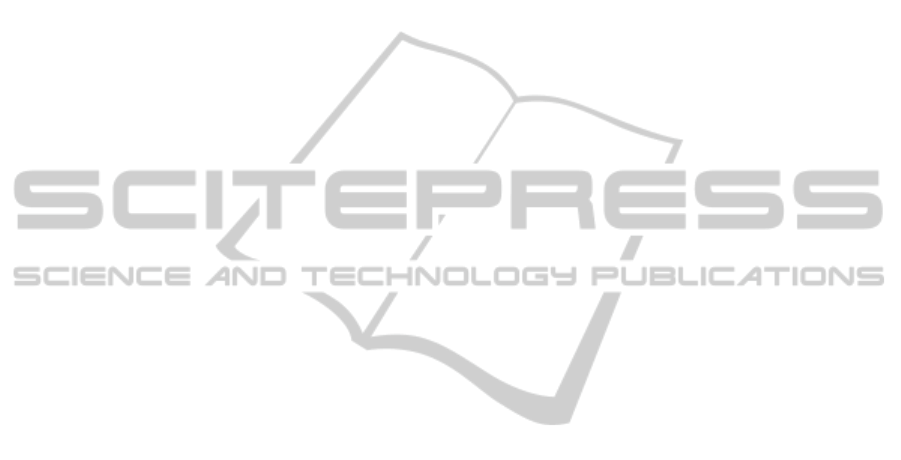
metric segmentation based on gaussian multiscale ag-
gregation. This method is able to isolate hand from
background in different situations, simulated by an
own synthetic public database, with a total of 408000
images.
The results highlight the fact that hand is isolated
with a competitive accuracy, providing a good result
for a posterior feature extraction, independently on
the background of the hand image.
Applications of this method are very suitable for
mobile applications, since hand mobile biometrics
must be able to identify individuals everywhere, with-
out no constrains on the background. However, more
efforts must be done to adapt this approach for mo-
bile biometrics, since its temporal performance is far
at present from being adequate for real-time applica-
tions. In addition, the time performance is still low
(18 seconds), when compared to other similar ap-
proaches in literature, and considering the challeng-
ing backgrounds to segment.
Future work regards an improvement and refine-
ment in implementation, together with a mobile ori-
entation, so that mobile hand biometrics could benefit
of a reliable segmentation algorithm, and therefore,
increase their identification accuracy.
ACKNOWLEDGEMENTS
This research has been supported by the Ministry
of Industry, Tourism and Trade of Spain, in the
framework of the project CENIT-Segur@, reference
CENIT-2007 2004.
REFERENCES
Alpert, S., Galun, M., Basri, R., and Brandt, A. (2007). Im-
age segmentation by probabilistic bottom-up aggrega-
tion and cue integration. In IEEE Conference on Com-
puter Vision and Pattern Recognition, 2007. CVPR
’07., pages 1–8.
Chen, S., Cao, L., Wang, Y., Liu, J., and Tang, X. (2010).
Image segmentation by map-ml estimations. Image
Processing, IEEE Transactions on, 19(9):2254 –2264.
Comaniciu, D., Meer, P., and Member, S. (2002). Mean
shift: A robust approach toward feature space analy-
sis. IEEE Transactions on Pattern Analysis and Ma-
chine Intelligence, 24:603–619.
de Berg, M., van Kreveld, M., Overmars, M., and
Schwarzkopf, O. (2008). Computational Geometry:
Algorithms and Applications. Springer, 3rd edition.
Felzenszwalb, P. F. and Huttenlocher, D. P. (2004). Efficient
graph-based image segmentation. Int. J. Comput. Vi-
sion, 59:167–181.
Garc´ıa-Casarrubios Mu˜noz, A., de Santos-Sierra, A.,
S´anchez-
´
Avila, C., Guerra-Casanova, J., Bailador-del
Pozo, G., and Jara-Vera, V. (2010). Hand biomet-
ric segmentation by means of fuzzy multiscale ag-
gregation for mobile devices. In Emerging Tech-
niques and Challenges for Hand-Based Biometrics
(ETCHB), 2010 International Workshop on, pages 1
–6.
Gonzalez, R. C. and Woods, R. E. (1992). Digital Im-
age Processing. Addison-Wesley Longman Publish-
ing Co., Inc., Boston, MA, USA.
Kang, W.-X., Yang, Q.-Q., and Liang, R.-P. (2009). The
comparative research on image segmentation algo-
rithms. In ETCS ’09: Proceedings of the 2009 First
International Workshop on Education Technology and
Computer Science, pages 703–707, Washington, DC,
USA. IEEE Computer Society.
Kukula, E. and Elliott, S. (2005). Implementation of hand
geometry at purdue university’s recreational center:
an analysis of user perspectives and system perfor-
mance. In Security Technology, 2005. CCST ’05. 39th
Annual 2005 International Carnahan Conference on,
pages 83–88.
Kukula, E. and Elliott, S. (2006). Implementation of hand
geometry: an analysis of user perspectives and sys-
tem performance. Aerospace and Electronic Systems
Magazine, IEEE, 21(3):3–9.
Meilˇa, M. (2005). Comparing clusterings: an axiomatic
view. In Proceedings of the 22nd international confer-
ence on Machine learning, ICML ’05, pages 577–584,
New York, NY, USA. ACM.
Mojsilovic, A., Hu, H., and Soljanin, E. (2002). Ex-
traction of perceptually important colors and similar-
ity measurement for image matching, retrieval and
analysis. Image Processing, IEEE Transactions on,
11(11):1238 – 1248.
Rory Tait Neilson, B. N. and McDonald, S. (2007). Image
segmentation by weighted aggregation with gradient
orientation histograms. Southern African Telecom-
munication Networks and Applications Conference
(SATNAC).
Sharon, E., Brandt, A., and Basri, R. (2000). Fast mul-
tiscale image segmentation. In IEEE Conference on
Computer Vision and Pattern Recognition, 2000. Pro-
ceedings., volume 1, pages 70 –77 vol.1.
Sharon, E., Brandt, A., and Basri, R. (2001). Segmenta-
tion and boundary detection using multiscale inten-
sity measurements. In IEEE Computer Society Con-
ference on Computer Vision and Pattern Recognition,
2001. CVPR 2001. Proceedings of the 2001., vol-
ume 1, pages I–469 – I–476 vol.1.
Sharon, E., Galun, M., Sharon, D., Basri, R., and Brandt, A.
(2006). Hierarchy and adaptivity in segmenting visual
scenes. Macmillan Publishing Ltd.
Shi, J. and Malik, J. (2000). Normalized cuts and image
segmentation. IEEE Transactions on Pattern Analysis
and Machine Intelligence, 22:888–905.
Shirakawa, S. and Nagao, T. (2009). Evolutionary image
segmentation based on multiobjective clustering. In
HAND IMAGE SEGMENTATION BY MEANS OF GAUSSIAN MULTISCALE AGGREGATION FOR BIOMETRIC
APPLICATIONS
45