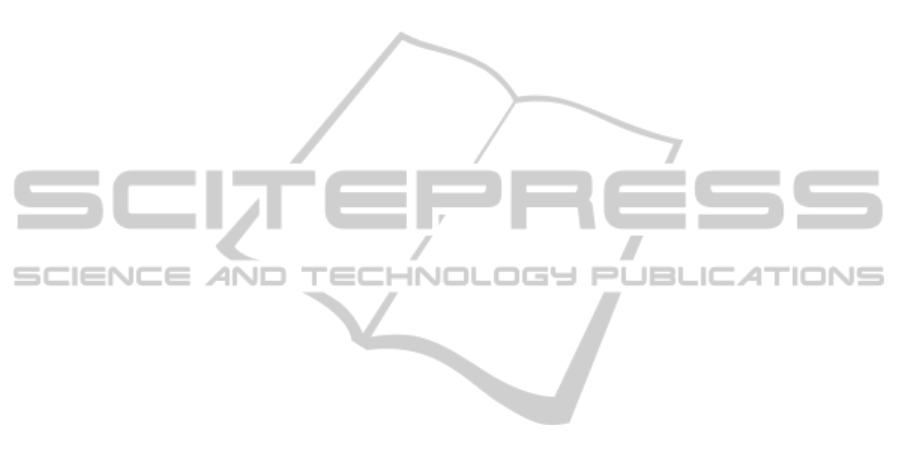
7 CONCLUSIONS
We incorporated the information about the target
variable into the process of split point identification
in PLR. The proposed approach is an easily
interpretable method which makes it very
convenient for experts of different fields of research
to use PLR, interpret the patterns and make
conclusions from the forecasts.
In this paper, the application of PLR in seasonal
forecasting in time series with nonlinear patterns is
newly introduced. The applicability and accuracy of
the proposed approach are demonstrated in a case
study at the City of Calgary.
The results show that the proposed approach is a
close competitor of the NN. This close performance
could be attributed to the NN’s non-linear nature
which provides the opportunity to relate different
variables to a target variable.
In this paper, we just based the forecasts on the
most recent linear pattern that corresponds to the
prediction point rather than considering the similar
patterns happening earlier than that. And that’s
because, as we mentioned in Section 1, “the recent
past contains more information about the immediate
future than the distant past”. However, this does not
take into account the possible changes on the
patterns (e.g. changes in the width or position of
time slots). In addition to this limitation of this
study, there is a limitation related to our dataset. The
available history data is limited to almost 2 years,
and that is because the residential Blue Cart program
was just launched in April 2009.
In our future work we intend to study the effect
of integrating a competitive learning method -similar
to the one used in learning the synopsis weight in
competitive neural networks- into the proposed
forecasting piecewise linear regression approach in
this paper. In this way, we will be able to take into
account the variation of the coefficients –which we
talked about in Section 1- in different time slots.
ACKNOWLEDGEMENTS
This research is part of the project CRD #386808-09
supported by NSERC Canada and the City of
Calgary (CoC). Special thanks to Scott Banack from
WRS at CoC for providing the data.
REFERENCES
Brown, R. L., Durbin, J. & Evans, J. M. 1975. Techniques
for Testing the Constancy of Regression Relationships
over Time. Journal of the Royal Statistical Society.
Series B (Methodological), 37, 149-192.
Cherkassky V. & Ma Y. 2005. Multiple Model Regression
Estimation, IEEE Transactions on Neural Networks,
16, 785-798.
Cortes, C. and Vapnik, V. 1995. Support-vector networks.
Machine Learning, 20, 273-297.
Ferrari-Trecate, G., Muselli, M. 2002. A new learning
method for piecewise linear regression In:
International Conference on Artificial Neural
Networks, Springer.
Fischer, M. 2008. Modeling and forecasting energy
demand: Principles and difficulties. In: Proceedings
of the NATO Advanced Research Workshop on
Weather/Climate Risk Management for the Energy
Sector, 207-226.
Guthery, S. B. 1974. Partition regression. Journal of the
American Statistical Association, 69, 945-947.
Hathaway, R. J. & Bezdek, J. C. 1993. Switching
regression models and fuzzy clustering. IEEE
Transactions on Fuzzy Systems 1, 195–204.
Honkela, A. 2001. Nonlinear Switching State-Space
Models, Master’s Thesis, Helsinki University of
Technology, Dep. of Engineering Physics and
Mathematics.
Höppner, F. & Klawonn, F. 2003. Improved fuzzy
partitions for fuzzy regression models. International
Journal of Approximate Reasoning 32, 85–102.
Jahandideh, S. et al. 2009. The use of artificial neural
networks and multiple linear regression to predict rate
of medical waste generation. Waste Management, 21,
2874-2879.
Kalaba, R., Rasakhoo, N. & Tesfatsion, L. 1989. A
FORTRAN program for time-varying linear regression
via flexible least squares. Computational Statistics &
Data Analysis, 7, 291-309.
Kuchenhof, H. 1996. An exact algorithm for estimating
breakpoints in segmented generalized linear models.
University of Munich.
Loesch, D. Z., et al. 2006. Transcript levels of the
intermediate size or grey zone fragile X mental
retardation 1 alleles are raised, and correlate with the
number of CGG repeats. Journal of medical genetics,
44.
Nusser, S., Otte, C. & Hauptmann, W. 2008. An EM-
Based Piecewise Linear Regression Algorithm.
Lecture notes in computer science, 466-474.
Niknafs, A., Sun, B., Richter, M. & Ruhe, G. 2011.
Predictions in Time Series with Repeated Patterns,
Using Piecewise Linear Regression, Technical Report
SEDS-TR-094/2011, University of Calgary.
RAPID-I, http://rapid-i.com/content/view/60/200/,
accessed at December 2010.
Sikka, G., Kaur, A. & Uddin, M. 2010. Estimating
Function points: Using Machine Learning and
Regression Models. In: 2nd International Conforence
on Education Technology and Computer, 52-56.
ICEIS 2011 - 13th International Conference on Enterprise Information Systems
182