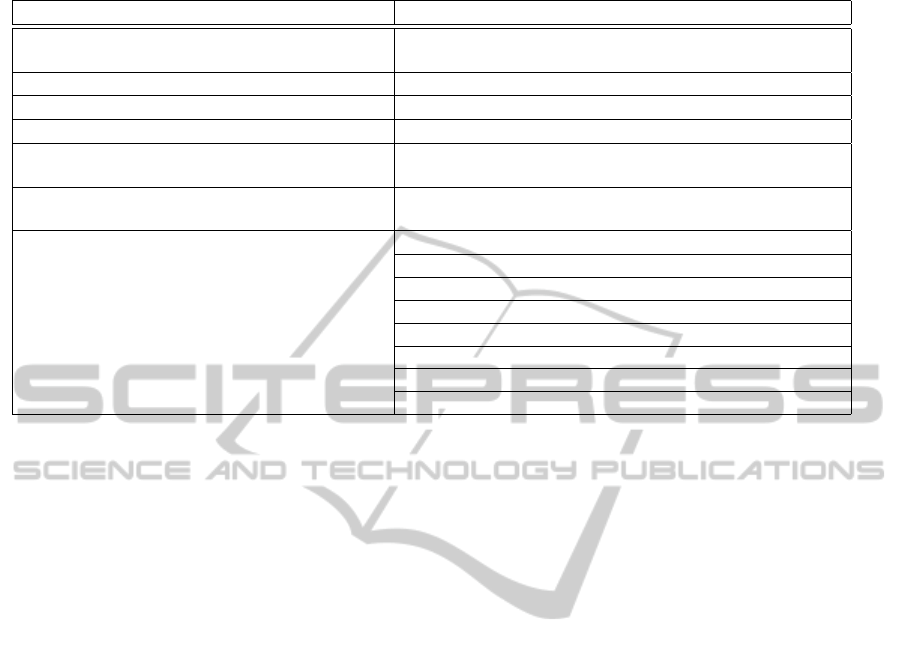
Table 4: Applied result to Thunderbird threads.
Manually generated clusters Concept-based algorithm clusters
Cluster 1: 216223, 216225,
227883
Cluster 1: 216223, 216225, 227883, 232432,
259951, 259959, 259331, 229879
Cluster 2: 218774, 240476, 240138, 243631 Cluster 2: 218774
Cluster 3: 232967, 289375, 284030 Cluster 3: 220173, 232967, 284030, 289375
Cluster 4: 229179, 229740, 230241 Cluster 4: 227841
Cluster 5: 220173, 231959, 234811,
234707
Cluster 5: 228300, 233944, 232433, 232432,
231960, 230925
Cluster 6: 227841, 230700, 232699, 231552,
229879, 228300
Cluster 6: 229179, 230241, 259289,
243631, 234707, 240476, 240138
Cluster 7: 257378, 259227, 273422, 259958,
259951, 259331, 259321, 259317, 259959,
259289, 258897, 258447
Cluster 7: 229740
Cluster 8: 230700
Cluster 9: 230925, 231552, 231960, 233944, 257378
Cluster 10: 231959, 234811
Cluster 11: 232433, 258897, 259317, 259321, 273422
Cluster 12: 232699, 258447
Cluster 13: 259227
Cluster 14: 259958
huge cluster containing all the documents in a corpus.
However, further testing of this clustering procedure
is probably needed to test its trustworthiness.
REFERENCES
Arimura, H., Abe, J., Fujino, R., Sakamoto, H., Shimozono,
S., and Arikawa, S. (2000). Text data mining: Discov-
ery of important keywords in the cyberspace. In Inter-
national Conference on Digital Libraries: Research
and Practice, pp.220-226.
Clifton, C., Cooley, R., and Rennie, J. (2004). Topcat: Data
mining for topic identification in a text corpus. IEEE
Transactions on Knowledge and Data Engineering,
Vol.16, No.8, pp.949-964.
Dan, K. and Christopher, D. M. (2003). Accurate unlexical-
ized parsing. In the 41st Meeting of the Association for
Computational Linguistics, pp. 423-430.
Ghani, R. and Fano, A. (2002). Using text mining to
infer semantic attributes for retail data mining. In
2002 IEEE International Conference on Data Min-
ing(ICDM 2002), pp.195-202.
Hammouda, K. and Kamel, M. (2004). Efficient phrase-
based document indexing for web document cluster-
ing. IEEE Transactions on Knowledge and Data En-
gineering, Vol.16, No.10, pp.1279-1296.
Hung, C. and Xiaotie, D. (2008). Efficient phrase-based
document similarity for clustering. IEEE Transactions
on Knowledge and Data Engineering, Vol.20, No.9,
pp.1217-1229.
Jing, P., Dong-qing, Y., Jian-wei, W., Meng-qing, W., and
Jun-gang, W. (2007). A clustering algorithm for short
documents based on concept similarity. In IEEE Pa-
cific Rim Conference on Communications, Computers
and Signal Processing, pp.42-45.
Li, Y., Bandar, Z., and Mclean, D. (2003). An approach
for measuring semantic similarity between words us-
ing multiple information sources. IEEE Transactions
on Knowledge and Data Engineering, Vol.15, No.4,
pp.871-882.
Li, Y., McLean, D., Bandar, Z., O’Shea, J., and Crockett,
K. (2006). Sentence similarity based on semantic nets
and corpus statistics. IEEE Transactions on Knowl-
edge and Data Engineering, Vol.18, No.8, pp.1138-
1150.
Malin, J., Millward, C., Schwarz, H., Gomez, F., Throop,
D., and Thronesbery, C. (2009). Linguistic text
mining for problem reports. In IEEE International
Conference on Systems, Man and Cybernetics(SMC
2009), pp.1578-1583.
Porter, M. (1980). An algorithm for suffix stripping. Pro-
gram, Vol.14, No.3, pp.130-137.
Shehata, S., Karray, F., and Kamel, M. (2010). An efficient
concept-based mining model for enhancing text clus-
tering. IEEE Transactions on Knowledge and Data
Engineering, Vol.22, No.10, pp.1360-1370.
Shen, W. and Angryk, R. (2007). Measuring semantic simi-
larity using wordnet-based context vectors. In IEEE
International Conference on Systems, Man and Cy-
bernetics(SMC 2007), pp.908-913.
Terachi, M., Saga, R., and Tsuji, H. (2006). Trends recogni-
tion in journal papers by text mining. In IEEE Interna-
tional Conference on Systems, Man and Cybernetics
2006(SMC2006), pp.4784-4789.
CONCEPT-BASED CLUSTERING FOR OPEN-SOURCED SOFTWARE(OSS) DEVELOPMENT FORUM THREADS
695