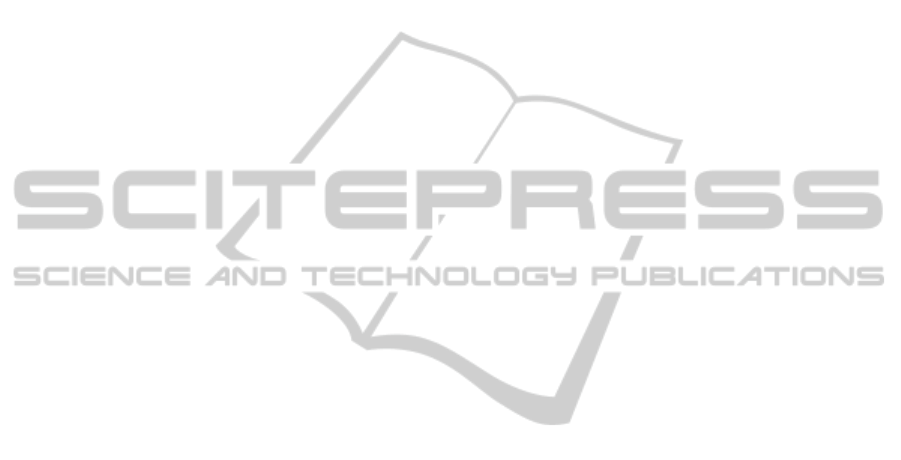
The artificial neural network grew up in the
1980s, developing through several periods
including initial growth, upsurge and climax,
troughs and the second rapid growth. The artificial
neural network imitates the real biological neural
network, consisting of quantities of neurons that
have none-linear mapping ability and connecting
each other with weight coefficients. The massive
parallel structure helps the network to acquire
wonderful characteristics like Automatic
Knowledge Acquisition, robustness, fault-
tolerance, learning ability and adaptability, and to
show superiority in setting up models for
complicated systems than traditional model
analysis models.
Because of its specific structure and methods in
dealing information, the artificial neural network is
being made great use of in numerous actual
applications, such as automatic control, image
processing, pattern recognition, signal processing,
robot control, welding, geographical analysis, data
mining, military affairs, transportation application,
mining industry, agriculture research, and
meteorological analysis and so on. Among a few
frequently-used neural networks, the BP neural
network is used most extensively, and its practical
utility is best embodied in forecasting time series
like national economy and population
development.
As a none-linear simulation technology with
fault-tolerance, learning ability and adaptability,
the artificial neural network provides a feasible and
practicable solution to solving problems concerning
high-speed railway passengers’ travel choices.
2 PRINCIPLES AND THE
STRUCTURE OF THE BP
NEURAL NETWORK MODEL
FOR FORECASTING
PASSENGERS’ TRAVEL
CHOICES OUT OF
ENVIRONMENTAL FACTORS
The study attempted to build a forecast model
using BP neural network to research on
environmental factors that affect high-speed
railway passengers’ travel choices based on a
survey conducted from January 1 to January 30 in
2010 with 1270 questionnaires that were
distributed at random to high-speed railway
passengers. Environmental factors that affect
passengers’ travel choice are definitely of various
kinds, the questionnaires used in the research
picked two main aspects including comfort level
and security level. Each of the aspects was set to
separate into eight degrees from “extraordinary
bad” to “extraordinary good”, from which
respondents could choose according to their own
experiences and feelings. Considering that different
means of conveyances would have various
influences on passengers’ selections, a third impact
factor was taken into account, namely “means of
conveyance”. The options set in this factor were:
1.hard-seat, 2.soft-seat, 3.hard sleeper, 4.soft
sleeper, 5.standing-room ticket, 6.unknown. For
the reason that some other factors besides
environmental factors would also have impacts on
passengers’ travel choices, all the others factors
were concluded into a forth aspect falling under
passengers’ degree of satisfaction as a whole in
order not to jeopardize the research on
environmental factors. The options set in the forth
aspect were also separated into eight degrees from
“extraordinary satisfied” to “extraordinary
unsatisfied” for respondents to choose from. In
view of the prediction of passenger’s next choice
on various traffic modes, a fifth question was set as
“The next traffic mode you would choose:”, and
the options were as follows: 1. the Multiple Units,
2. The Through Train, 3. The inter-city bus, 4.
Aircrafts, 5. Self-driving. The ultimate result of the
survey consisting of 1270 eligible questionnaire,
from which 503 valid records were screened based
on Rough Set methods.
The BP Neural network usually consists of
three layers including the input layer, the hidden
layer and the output layer. The numbers of nodes in
the input and output layer are decided by sample
dimensions constructed by data processor, and the
number of nodes in the hidden layer is determined
by both of the numbers of the input and output
layer. The work process of the Neural network is
composed of two stages, one is the work period, in
which the weights connected each nodes are fixed
and calculating each unit’s state change in order to
keep stability; the other is the learning period, in
which each calculation unit’s state is fixed while
the weights connecting them are changeable and
modified. A neural network is train for the purpose
that a group of input vectors could produce a group
of expecting and efficient output vectors. The
training process is realized through certain process
(Learning Algorithm) in which the network’s
weights are modified based on a series of training
samples.
HIGH-SPEED RAILWAY PASSENGERS' CHOICES OF TRAVEL FORECAST BASED ON MATLAB NEURAL
NETWORK
255