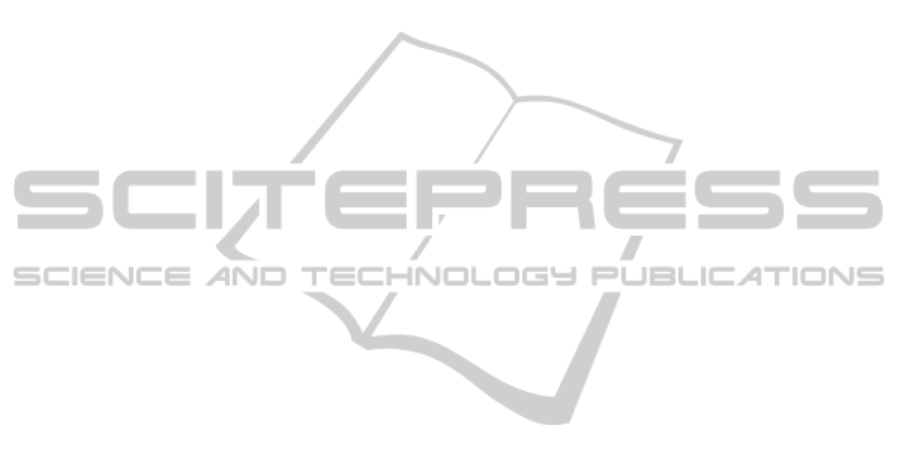
member’s knowledge. In this work, we take the
group perspective into consideration to offset the
drawback of the personal perspective. However, the
group perspective may neglect the information needs
of an individual because it focuses on the needs of
the majority of the group’s members. Since the
group-based method and the personalized method
have distinct advantages, we combined them to
exploit their respective merits. Our experiment
results show that the hybrid method certainly
improve the recommendation quality.
ACKNOWLEDGEMENTS
This research was supported by the National Science
Council of the Taiwan under the grant NSC 96-
2416-H-009-007-MY3 and NSC 99-2410-H-009-
034-MY3.
REFERENCES
Baeza-Yates, R. & Ribeiro-Neto, B. 1999. Modern
Information Retrieval, Boston, Addison-Wesley.
Balabanovic, M. & Shoham, Y. 1997. Fab: content-based,
collaborative recommendation. Communication of the
ACM, 40, 66-72.
Breese, J. S., Heckerman, D. & Kadie, C. 1998. Empirical
Analysis of Predictive Algorithms for Collaborative
Filtering. In: Proceedings of the Fourteenth Annual
Conference on Uncertainty in Artificial Intelligence,
43-52.
Herlocker, J. L., Konstan, J. A., Terveen, L. G. & Riedl, J.
T. 2004. Evaluating collaborative filtering
recommender systems. ACM Transactions on
Information Systems (TOIS), 22, 5-53.
Holz, H., Maus, H., Bernardi, A. & Rostanin, O. 2005. A
lightweight approach for proactive, task-specific
information delivery. In: Proceedings of the 5th
International Conference on Knowledge Management
(I-Know), 101-127.
Jain, A. K., Murty, M. N. & Flynn, P. J. 1999. Data
clustering: a review. ACM Computing Surveys (CSUR),
31, 264-323.
Jameson, A. 2004. More than the sum of its members:
challenges for group recommender systems. In:
Proceedings of the working conference on Advanced
visual interfaces, Gallipoli, Italy, 48-54.
Kim, J. K., Kim, H. K., Oh, H. Y. & Ryu, Y. U. 2010. A
group recommendation system for online communities.
International Journal of Information Management, 30,
212-219.
Konstan, J. A., Miller, B. N., Maltz, D., Herlocker, J. L.,
Gordon, L. R. & Riedl, J. 1997. GroupLens: applying
collaborative filtering to Usenet news.
Communications of the ACM, 40, 77-87.
Lai, C. H. & Liu, D. R. 2009. Integrating Knowledge Flow
Mining and Collaborative Filtering to Support
Document Recommendation. Journal of Systems and
Software, 82, 2023-2037.
Liu, D. R., Wu, I. C. & Yang, K. S. 2005. Task-based K-
Support system: disseminating and sharing task-
relevant knowledge. Expert Systems With Applications,
29, 408-423.
Luo, X., Hu, Q., Xu, W. & Yu, Z. 2008. Discovery of
Textual Knowledge Flow Based on the Management
of Knowledge Maps. Concurrency and Computation:
Practice and Experience, 20, 1791-1806.
Mccarthy, J. F. & Anagnost, T. D. 1998. MusicFX: an
arbiter of group preferences for computer supported
collaborative workouts. In: Proceedings of the ACM
conference on computer supported cooperative work
(CSCW), Seattle, Washington, United States, 363-372.
O'connor, M., Cosley, D., Konstan, J. A. & Riedl, J. 2001.
PolyLens: a recommender system for groups of users.
In: Proceedings of the seventh conference on
European Conference on Computer Supported
Cooperative Work, Bonn, Germany, 199-218.
Oguducu, S. G. & Ozsu, M. T. 2006. Incremental click-
stream tree model: Learning from new users for web
page prediction. Distributed and Parallel Databases,
19, 5-27.
Zhuge, H. 2002. A Knowledge Flow Model for Peer-to-
peer Team Knowledge Sharing and Management.
Expert Systems With Applications, 23, 23-30.
Zhuge, H. 2006. Discovery of Knowledge Flow in Science.
Communications of the ACM, 49, 101-107.
RECOMMENDING DOCUMENTS VIA KNOWLEDGE FLOW-BASED GROUP RECOMMENDATION
349