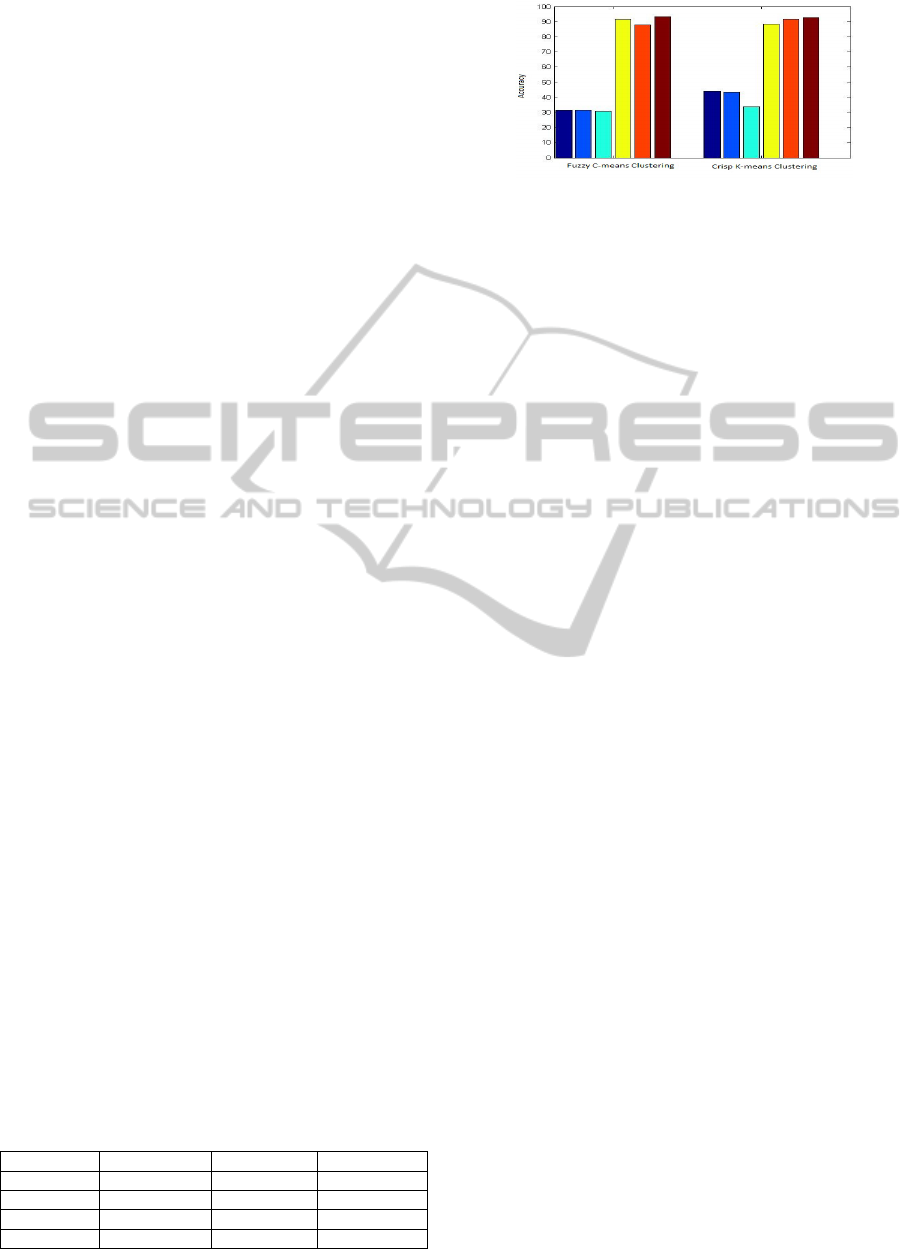
3.3 Dimensionality Reduction
The dimension that is found after the key word
extraction is huge to handle so we had to reduce the
dimension. As there are almost 10,000 features in a
document, we got the vectors with 10,000
dimensions. We use PCA first to reduce this huge
dimension. Then we use the Factor Analysis (FA)
and LDA. The PCA first reduce the dimension to
250. Then two other techniques, one is supervised
(LDA) and other is unsupervised (FA) to make it
down to 4 and 5. We have tested with other
dimensions and find that the clustering algorithms
do not perform well in higher dimensions. It
performs best in 4th and 5
th
dimensions.
3.4 Clustering
We use both traditional and fuzzy c-means
clustering to group articles. We analyze the
performance through confusion matrix. The samples
in the fuzzy clustering algorithm if had a
membership to a particular cluster as maximum
membership for that sample m, then, we checked
whether the sample gives k*m membership to other
clusters. This will introduce the concept that we
explained earlier that which article goes to which
cluster can sometimes vary on the context. This way
we could create the opportunity to classify one
article as crime and say, politics if necessary. The
crisp c-means doesn’t have that special feature.
4 RESULT ANALYSIS AND
DISCUSSION
The performance of the two clustering methods were
not much apart in terms of the accuracy. Although
our expectation was that the fuzzy clustering would
perform better, the traditional c-means algorithm
also perfom well. The variaion of the results is due
to different feature reduction technique.The
accuracy of the clustering techniques is presented in
Table 2.
The blue shades in Figure 3 are the accuracy rates
for Factor Analysis feature reduction technique and
others are for LDA feature reduction technique. The
graph clearly visualizes the differences between the
Table 2: Accuracy Rates.
3 Feat 4 Feat 5 Feat
Fuzzy LDA 91.5663 87.9518 93.3735
Fuzzy FA 31.3253 31.3253 30.7229
Crisp LDA 88.5542 91.5663 92.7711
Crisp FA 43.9759 43.3735 33.7349
Figure 3: Accuracy plots.
two feature reductions techniques although, the
algorithm for the clustering does not much differ.
Both of the algorithms are iterative and they
recalculate the centroids for each cluster using some
data for the particular algorithm. The traditional c-
means algorithm calculates the Euclidian distance to
calculate the centroids in each iteration and the
fuzzy c-means calculates the centroids using the
membership values which again are calculated by
the Euclidian distance. So, getting the similar result
is more likely.
LDA is a supervised dimensionality reduction
technique. Therefore, the extracted features get
biased towards a particular class. This helps the
samples of the same class to converge to a particular
cluster. Since FA is unsupervised, i.e., it does not
use any class information, hence samples are
grouped more sparsely than LDA in dimension
space.
The features for the data are extracted in two
different techniques. First we use LDA to reduce to
5 features. Each row in the Table 3 represents a
single class of article that was collected into the
database. Each column represents the cluster into
which a particular sample has been included.
However, the cluster number does not necessarily
correspond to the class number. In this table, what is
important to note is, how samples of a particular
class exists in a particular cluster. For example, most
of the samples of class 4 have been clustered in
cluster 4 (cl4). On the other hand, most samples of
class 7 belong to cluster 1, the rest belong to cluster
8. The point that we want to stress is that the cluster
number is arbitrary and has nothing to do with a
particular class. In this case 92% of class 7 is
clustered in cluster 1; we consider this as the
accuracy of the clustering for class 7. Using the
above theory, the average accuracy of this system, is
93.3735%. Now let us take a look at the membership
values for all of one class into all clusters. Here the
following example shows the membership values for
article class 6 for the current system.
INDEXING BANGLA NEWSPAPER ARTICLES USING FUZZY AND CRISP CLUSTERING ALGORITHMS
363