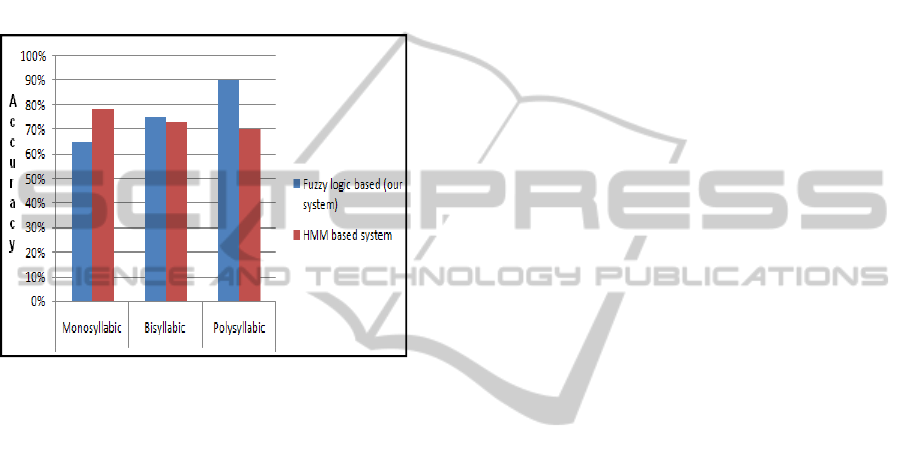
rates that we found are illustrated below in Figure
13.
It is an interesting finding that the fuzzy logic
based recognition recognizes the relatively more
difficult words (polysyllabic) better than HMM
systems to a greater extent than that of easier or
shorter words. It also coincides to our understanding
that our system gives better performance in Bangla
speech since it has been specifically trained for
Bangla word recognition and it works on the “word-
level” rather than the “phonetic level.”
Figure 13: Comparative result of HMM and Fuzzy logic
based system.
5 CONCLUSIONS
The system developed by us is one of the first
speech recognition attempts in Bangla speech using
fuzzy logic. However it is not without its limitations.
This particular system could be extended to
recognize continuous speech. Moreover the overall
accuracy of the system could be further improved
using the modern technical tools of today (even
though fuzzy logic has to be the base for all
linguistic ambiguity-related problems). As an end-
note it can be said that speech recognition was an
“open” problem before our system and it remains the
same upon completion of the system – but it is a
considerable step in reaching one of the solutions to
an “open” problem using spectral analysis and fuzzy
logic in Bangla speech.
REFERENCES
Abul, Md. H., Jabir, M., Mumit, K, 2007. Isolated and
Continuous Bangla Speech Recognition:
Implementation, Performance and application
perspective, in SNLP 07, Kasetsart University,
Bangok, Thailand
Davies, K. H., Biddulph, R., Balashek, S., 1952.
Automatic Speech Recognition of Spoken Digits, J.
Acoust. Soc. Am. 24(6) pp.637 –642.
Dragon Natural Speaking (DNS), 2010, Wikipedia
Encyclopedia, 2010. Available:
http://en.wikipedia.org/wiki/Dragon_NaturallySpeakin
g
Fletcher, H., 1922. The Nature of Speech and its
Interpretations, Bell Syst. Tech. J., Vol 1, pp. 129-
144.
Hasan, M. R., Nath, B., Alauddin B. M. , 2003. Bengali
Phoneme Recognition: A New Approach, in 6th ICCIT
conference, Dhaka.
Illinois Image Formation and Processing (IIFP), 2010.
DSP Mini-Project: An Automatic Speaker Recognition
System [Online]. Available:
http://www.ifp.illinois.edu/~minhdo/teaching/speaker_
recognition/speaker_recognition.html
Islam, M. R., Sohail, A. S. M., Sadid, M. W. H.M.,
Mottalib, A., 2005. Bangla Speech Recognition using
three layer Back-Propagation Neural Network, in
NCCPB, Dhaka.
Juang, B. H., Rabiner, L. R., 2005. Automatic Speech
Recognition -A Brief History of the Technology,
Elsevier Encyclopedia of Language and Linguistics,
Second Edition, Amsterdam, Holland.
Karim, A H M. R, Rahman, Md. S., Iqbal, Md.Zafar,
2002. Recognition of Spoken Letters in Bangla, in 6th
ICCIT conference, Dhaka.
Nuance Communications (NComm), (2010) Available:
http://www.nuance.com/naturallyspeaking/
Rahman, K. J., Hossain,M.A., Das, D., Islam, T. A. Z. and
Ali, M.G., 2003. Continuous Bangla Speech
Recognition System, in 6th Int. Conf. on Computer and
Information Technology (ICCIT), Dhaka.
Roy, K., Das, D., Ali, M.G, 2002. Development of the
Speech Recognition System Using Artificial Neural
Network, in 5th ICCIT conference, Dhaka.
Spectrogram on Wikipedia Encyclopedia, 2010. [Online].
Available: http://en.wikipedia.org/wiki/Spectrogram
Short-time Fourier Transform (STFT),Wikipedia
Encyclopedia, 2010. [Online]. Available:
http://en.wikipedia.org/wiki/STFT
Traunmüller, H., Eriksson, A., 1995. Publications of
Hartmut Traunmüller, Stockholm University, Sweden
[Online]. Available:
http://www.ling.su.se/staff/hartmut/f0_m&f.pdf
Weiss, M., 2006 . Indo-European Language and Culture,
Journal of the American Oriental Society [Online] .
Available:
http://findarticles.com/p/articles/mi_go2081/is_2_126/
ai_n29428508/
ICEIS 2011 - 13th International Conference on Enterprise Information Systems
82