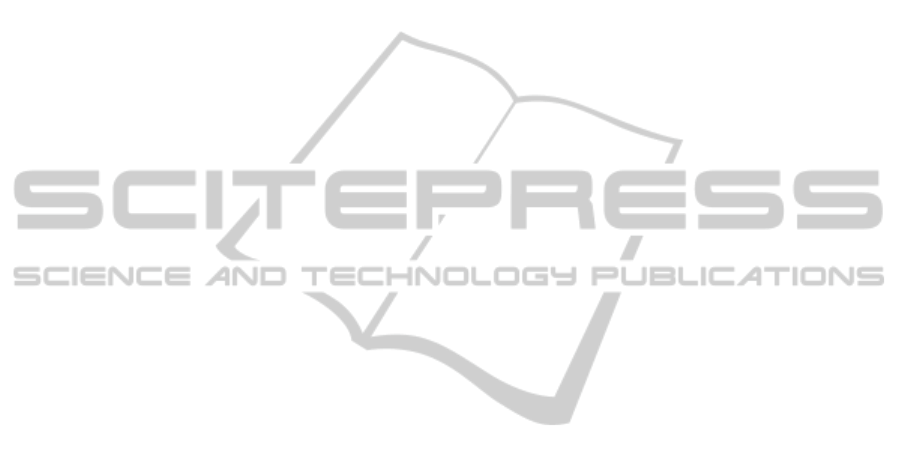
6 CONCLUSIONS
In this study a software sensor was developed with
the proposed method, a fault signature by means of
PCA contribution plots, to classify qualitative vari-
ables from quantitative variables of the process for
future released batches.
To prove the proposed method a biological nutri-
ent removal (BNR) sequencing batch reactor (SBR)
for wastewater treatment was used as an example of
a batch process. In this example the objective was
the diagnosis of the biological nutrient removal (qual-
itative variable) of the effluent wastewater processed
by classifying the batches detected as faulty. The re-
sult for the diagnosis featured high classification rates
for the faulty batches, where the correct classification
of the nutrients was an accuracy of more than above
97%.
The accuracy of the method to predict the quality
of the product, in this case the processed wastewater,
helps in reduced time to know if the product has a
high biological nutrient removal efficiency, and will
lead to faster action to correct a faulty process. In this
example case, the implementation of the system can
save a high investment with respect to the purchase of
sensors that measure water quality that can cost more
than an entire wastewater plant of small dimensions.
For future studies, applying the method in differ-
ent batch processes would improve the robustness of
the software sensor. Improve representations of the
faulty stages for the fault signature would help clas-
sify qualitative variables and would therefore result in
an accurate diagnosis of the product released.
ACKNOWLEDGEMENTS
The authors wish to thank the Spanish Government
(CTQ2008-06865-C02-02), with the support of the
CUR, the DIUE, the Generalitat of Catalonia and the
European Social Fund.
REFERENCES
Barker, M. and Rawtani, J. (2005). Practical Batch Process
Management. Elsevier.
Cleary, J. G. and Trigg, L. E. (1995). K*: An instance-
based learner using an entropic distance measure. In
12th International Conference on Machine Learning
(1995), pages 108–114.
Fan, L. and Xu, Y. (2007). A pca-combined neural net-
work software sensor for sbr processes. In Liu, D.,
Fei, S., Hou, Z., Zhang, H., and Sun, C., editors, Ad-
vances in Neural Networks ISNN 2007, volume 4492
of Lecture Notes in Computer Science, pages 1042–
1047. Springer Berlin / Heidelberg.
Hong, S. H., Lee, M. W., Lee, D. S., and Park, J. M. (2007).
Monitoring of sequencing batch reactor for nitrogen
and phosphorus removal using neural networks. Bio-
chemical Engineering Journal, 35(3):365 – 370.
Kim, Y., Bae, H., Poo, K., Kim, J., Moon, T., Kim, S., and
Kim, C. (2006). Soft sensor using pnn model and rule
base for wastewater treatment plant. In Wang, J., Yi,
Z., Zurada, J., Lu, B.-L., and Yin, H., editors, Ad-
vances in Neural Networks - ISNN 2006, volume 3973
of Lecture Notes in Computer Science, pages 1261–
1269. Springer Berlin / Heidelberg.
Kourti, T. (2005). Application of latent variable methods
to process control and multivariate statistical process
control in industry. Int. J. Adapt. Control, 19:213–246.
Lee, D. S. and Park, J. M. (1999). Neural network mod-
eling for on-line estimation of nutrient dynamics in
a sequentially-operated batch reactor. Journal of
Biotechnology, 75(2-3):229 – 239.
Lee, Y.-H., Lee, D.-Y., and Han, C. (1999). Rmbatch: Intel-
ligent real-time monitoring and diagnosis system for
batch processes. Computers & Chemical Engineer-
ing, 23(Supplement 1):S699 – S702. European Sym-
posium on Computer Aided Process Engineering, Pro-
ceedings of the European Symposium.
Leung, D. and Romagnoli, J. (2002). An integration mech-
anism for multivariate knowledge-based fault diagno-
sis. Journal of Process Control, 12(1):15 – 26.
Nomikos, P. and MacGregor, J. F. (1994). Monitoring batch
processes using multiway principal component analy-
sis. AIChE J., 40(8):1361–1375.
Nomikos, P. and MacGregor, J. F. (1995). Multivariate spc
charts for monitoring batch processes. Technometrics,
37(1):41–59.
Puig, S., Corominas, L., Balaguer, M., and Colprim, J.
(2007). Biological nutrient removal by applying sbr
technology in small wastewater treatment plants: Car-
bon source and c/n/p ratio effects. Water Sci. Technol.,
55(7):135–141.
Westerhuis, J. A., G., S. P., and Smilde, A. K. (2000).
Generalize contribution plots in multivariate statisti-
cal process monitoring. Chemom. Intell. Lab. Syst.,
51:95–114.
Wold, S., Esbensen, K., and Geladi, P. (1987). Princi-
pal component analysis. Chemom. Intell. Lab. Syst.,
2(1):37–52.
Wong, A., Colomer, J., Coma, M., and Colprim, J. (2010).
Pca intelligent contribution analysis for fault diagno-
sis in a sequencing batch reactor. In Proceedings of
the iEMSs Fifth Biennial Conference, volume 3, pages
2230–2237.
Xiao, F., Wang, S., Xu, X., and Ge, G. (2009). An isolation
enhanced pca method with expert-based multivariate
decoupling for sensor fdd in air-conditioning systems.
Applied Thermal Engineering, 29(4):712 – 722.
ICEIS 2011 - 13th International Conference on Enterprise Information Systems
228