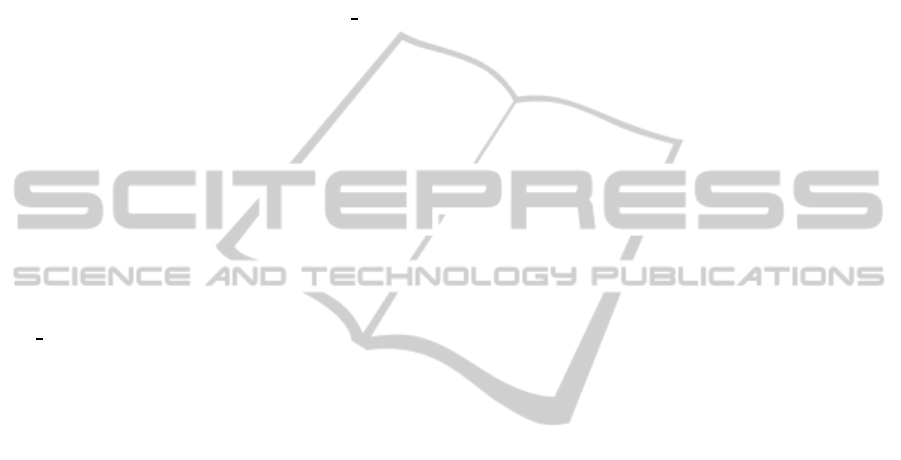
the methods have been statistically compared using
Wilcoxon, Friedman and Holm tests. From our exper-
iments results we make 3 observations: (i) Feature Se-
lection techniques gave poor performances. The rea-
son is that the Laplacianan Score selected all categori-
cal features and very few categorical features. Hence,
the dissimilarity measured applied by each OCC can-
not be calculated correctly with certain types of data.
(ii) In a few sites, OCC did not work well because our
characteristics set does not contain any feature that
discriminates against these classes. (iii) Gauss dd was
the best method for almost all sites because feature
values fit closely to a normal distribution.
5 CONCLUSIONS AND FUTURE
WORK
In this paper, we discussed OCC techniques for solv-
ing Information Extractor verify problems. Five basic
OCC methods were studied. A comprehensive eval-
uation of these methods was conducted to compare
their performances which enable us to conclude that
Gauss dd outperforms all the testing techniques.
Still, there are several problems that are open for
research. Feature database and pre-processing phases
have not been exploited very much for our problem.
Another point to note here is that classifier ensembles
and other sophisticated OCC like SVM or Bayesian
Network approach have not been investigated. Also,
data complexity measures would be an interesting ex-
ercise, if we want a quick way to choose an OCC
yielding good performance for a particular site.
ACKNOWLEDGEMENTS
This work is supported by the European Commission
(FEDER), the Spanish and the Andalusian R&D&I
programmes (grants TIN2007-64119, P07-TIC-2602,
P08-TIC-4100, TIN2008-04718-E, TIN2010-
21744, TIN2010-09809-E, TIN2010-10811-E, and
TIN2010-09988-E).
REFERENCES
Bernstein, P. A. and Haas, L. M. (2008). Information inte-
gration in the enterprise. Commun. ACM, 51(9):72–
79.
Chandola, V., Banerjee, A., and Kumar, V. (2009).
Anomaly detection: A survey. ACM Computing Sur-
veys, 41(3).
Chidlovskii, B., Roustant, B., and Brette, M. (2006). Doc-
umentum eci self-repairing wrappers: performance
analysis. In SIGMOD ’06: Proceedings of the 2006
ACM SIGMOD international conference on Manage-
ment of data, pages 708–717, New York, NY, USA.
ACM.
Demsar, J. (2006). Statistical comparisons of classifiers
over multiple data sets. Journal of Machine Learning
Research, 7:1–30.
Garc´ıa, S., Fern´andez, A., Luengo, J., and Herrera, F.
(2010). Advanced nonparametric tests for multi-
ple comparisons in the design of experiments in
computational intelligence and data mining: Exper-
imental analysis of power. Information Sciences,
180(10):2044–2064. Special Issue on Intelligent Dis-
tributed Information Systems.
Hempstalk, K., Frank, E., and Witten, I. H. (2008). One-
class classification by combining density and class
probability estimation. In Proceedings of the 2008 Eu-
ropean Conference on Machine Learning and Knowl-
edge Discovery in Databases - Part I, ECML PKDD
’08, pages 505–519, Berlin, Heidelberg. Springer-
Verlag.
Hodge, V. J. and Austin, J. (2004). A survey of outlier de-
tection methodologies. Artificial Intelligence Review,
22:2004.
Kushmerick, N. (2000). Wrapper induction: Efficiency and
expressiveness. Artificial Intelllligence, 118(1-2):15–
68.
Lerman, K., Minton, S. N., and Knoblock, C. A. (2003).
Wrapper maintenance: A machine learning approach.
Journal of Artificial Intelligence Research, 18:2003.
Madhavan, J., Cohen, S., Halevy, A. Y., Jeffery, S. R.,
Dong, X. L., Ko, D., and Yu, C. (2007). Web-scale
data integration: You can afford to pay as you go. In
CIDR, pages 342–350.
McCann, R., AlShebli, B., Le, Q., Nguyen, H., Vu, L.,
and Doan, A. (2005). Mapping maintenance for data
integration systems. In VLDB ’05: Proceedings of
the 31st international conference on Very large data
bases, pages 1018–1029. VLDB Endowment.
Tax, D. (2009). Ddtools, the data description toolbox for
matlab. version 1.7.3.
Tax, D. M. J. (2001). One-class classification, concept
learning in the absence of counter example. PhD the-
sis, Delft University of Technology.
Villalba, S. D. and Cunningham, P. (2007). An evaluation of
dimension reduction techniques for one-class classifi-
cation. Artificial Intelligence Review, 27(4):273–294.
Weiss, J. (2005). Aligning relationships: Optimizing the
value of strategic outsourcing. Technical report, IBM.
ICSOFT 2011 - 6th International Conference on Software and Data Technologies
46