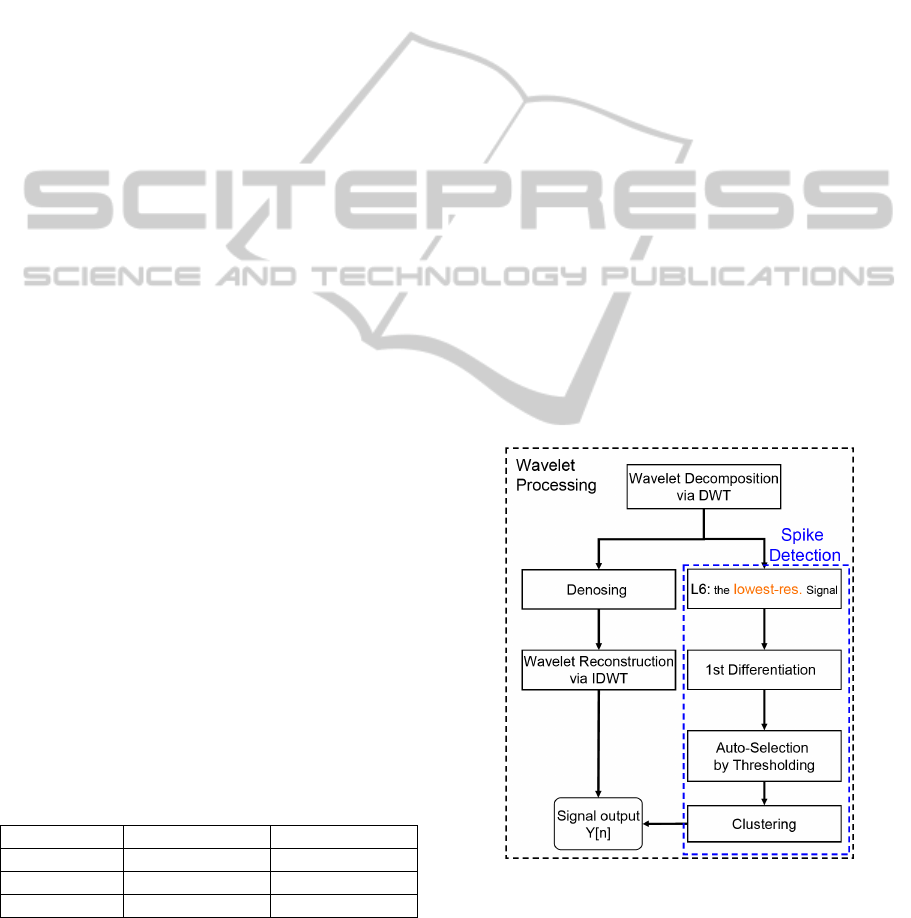
The wavelet transform (WT) is an efficient tool
for multi-resolution analysis of non-stationary and
fast transient signals. These properties make it
especially suitable to study the neurophysiological
signals. Numerous WT applications in biosignal
analysis have been proposed, including for EMG
analysis (Arikidis et al., 2002, Kumar et al., 2003).
In our previous study (Jiang and Kuo, 2008), we
have developed a wavelet denosing method that can
automatically detect the occurrence of SEMG
epochs and render more consistent and stable epoch
strength. Based on this denoising method, this study
further applies feature extraction and analysis of
SEMG signal in both time and frequency domain to
explore the changes in neuromuscular function with
MPF.
2 MATERIALS AND METHODS
2.1 SEMG Measurement
We recruited two groups of participants with the age
ranged from 30 to 50 years old. One was the patient
group and the other is normal group. Table 1
provides the descriptive information of the
participants. In order to make a consistent condition,
only the right-handed participants were selected.
The active electrodes MA-411 were attached on
the taut-band loci at the right side of the upper back
to measure the SEMG signal. The analogue signal
was amplified up to 3800 times and band-passed
(20Hz to 3,000Hz) by MA-411 and then digitized
with 5000 kHz sampling rate by instruNet 100 data
acquisition card and transferred to computer for
further analysis.
The participant conducted only five repetitive
trials. For each trial, participants lay down on their
stomach steadily for standby at the first three beats
and lifted both their arms toward the ceiling on the
4th beat with their maximal force, released the hold
on the 5th beat. One beat last one second, so each
trial last for 5 seconds.
Table 1: Descriptive information about participants.
Variable patient group normal group
Number 13 13
age (years) 41.2±6.2 39.5±7.3
weight (kg) 58.6±7.5 59.3±8.7
2.2 Denoising and Epoch Detection
The overall procedure to detect the occurrences of 1-
sec epoch for each contraction is summarized in
Figure 1. According to our previous comparative
study, we first applied Universal-soft denoising
method to yield the reconstructed signal with the
best signal quality. Following that, the 1
st
differentiation of the denoised signal was calculated
to detect the abrupt spikes, contained in each epoch.
Finally, the dominate spike, indicating the central
location of the epoch, was detected by using our
developed multi-resolution thresholding algorithm
based on statistical clustering process. The principle
of the algorithm is based on the existence of an
optimal threshold that should separate the population
into two groups with maximal between-class
variance. This concept was originally proposed by
Otsu for image segmentation (Otsu, 1979).
However, the distribution of the SEMG signal
derivatives is not like an image histogram with
definite discrete levels. To circumvent this problem
of indefinite derivatives, we developed a novel auto-
thresholding algorithm with multi-resolution
concept. The algorithm is described in detail in the
reference(Jiang and Kuo, 2008).
Once the central location of the epoch is
determined, then the duration can be spread
symmetrically toward both side of it within 1 sec.
(each side contains 2500 sampling points). The
following feature analysis was subject to the
detected epochs.
Figure 1: Wavelet processing.
2.3 SEMG Analysis
Three features were extracted from the SEMG
activity for group comparisons to examine the
SIGMAP 2011 - International Conference on Signal Processing and Multimedia Applications
204